Dr.-Ing. Paul Bodesheim
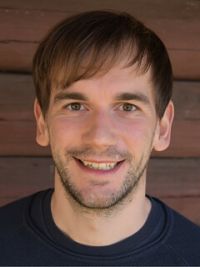
Address: | Computer Vision Group Department of Mathematics and Computer Science Friedrich Schiller University of Jena Ernst-Abbe-Platz 2 07743 Jena Germany |
Phone: | +49 (0) 3641 9 46410 |
E-mail: | paul (dot) bodesheim (at) uni-jena (dot) de |
Room: | 1218 |
Links: | DBLP, GoogleScholar, LinkedIn, ORCID, publons, ResearchGate, SemanticScholar, XING |
Curriculum Vitae
since Oct 2018 |
Research Associate / Postdoc Computer Vision Group (Prof. Joachim Denzler), Friedrich Schiller University Jena |
|
Aug 2022 to Sep 2022 |
Visiting Academic for two month at the University of Bristol (UK) Research stay in the group of Tilo Burghardt, Department of Computer Science |
|
Jun 2020 to Dec 2022 |
Team Leader: “Computer Vision and Machine Learning” Computer Vision Group (Prof. Joachim Denzler), Friedrich Schiller University Jena |
|
Jan 2015 to Sep 2018 |
Research Associate / Postdoc Department Biogeochemical Integration (Prof. Markus Reichstein), Max Planck Institute for Biogeochemistry |
|
Apr 2011 to Dec 2014 |
Research Associate / PhD Student Computer Vision Group (Prof. Joachim Denzler), Friedrich Schiller University Jena PhD Thesis: “Discovering unknown visual objects with novelty detection techniques” |
|
Oct 2006 to Mar 2011 |
Studies in Computer Science (Diploma) Friedrich Schiller University Jena Focus: Digitial Image Processing / Computer Vision Diploma Thesis: “Object Discovery – Unsupervised Learning of Object Categories” |
Awards
- Best Paper Award at DeLTA 2024
(Kim Bjerge, Paul Bodesheim, Henrik Karstoft: “Few-Shot Learning with Novelty Detection”) - Best Paper Award at Anomaly Detection Workshop of ICML 2016
(Rodner, Barz, Guanche, Flach, Mahecha, Bodesheim, Reichstein, Denzler: “Maximally Divergent Intervals for Anomaly Detection”) - Best Poster Award at FEAST Workshop of ICPR 2014
(Freytag, Rühle, Bodesheim, Rodner, Denzler: “Seeing through bag-of-visual-word glasses: towards understanding quantization effects in feature extraction methods”) - Best Paper Honorable Mention Award at ACCV 2012
(Freytag, Rodner, Bodesheim, Denzler: “Rapid Uncertainty Computation with Gaussian Processes and Histogram Intersection Kernels”)
Research Interests
- Visual Object Recognition
- Learning from Small and Imbalanced Data
- Fine-grained Recognition and Applications in Biodiversity Research
- Novelty Detection and Open Set Recognition
- Active Learning and Lifelong Learning
Projects (Third-Party Funds)
Ongoing
- LEPMON: Monitoring Biodiversity of Moths (Lepidoptera) Using Automated Camera Traps and Artificial Intelligence (since 2023)
- InsectAI: EU COST Action Network on Using Image-based AI for Insect Monitoring & Conservation (since 2023)
Former
- BACI: Towards a Biosphere Atmosphere Change Index (Research Associate, 2015-2019)
- AMMOD: Automated Multisensor station for Monitoring Of species Diversity (Subproject Leader, 2019-2023)
Projects (Further)
Ongoing
Former
Reviewer Activities
- CVPPA 2021 Workshop
- Mammalian Biology (2021)
- IEEE Transactions on Pattern Analysis and Machine Intelligence (TPAMI, 2020, 2024)
- Journal of Machine Learning Research (JMLR, 2020)
- Signal Processing: Image Communication (IMAGE, 2017-2018)
- IEEE Transactions on Neural Networks and Learning Systems (TNNLS, 2014)
Teaching
Winter Terms
- “Maschinelles Lernen und Datamining” / Machine Learning and Data Mining
-
- Lecture (4 SWS): 2022/2023, 2021/2022
- “Informatik (B.Sc. Werkstoffwissenschaften)” / Computer Science for Materials Scientists
-
- Lecture (3 SWS): 2024/2025, 2022/2023, 2021/2022, 2020/2021, 2019/2020, 2018/2019
- Exercise (3 SWS): 2018/2019
- “Informatik II (B.Sc. Physik)” / Computer Science II for Physicists
-
- Lecture (2 SWS): 2022/2023, 2021/2022, 2020/2021, 2019/2020, 2018/2019
- Exercise (1 SWS): 2019/2020, 2018/2019
Summer Terms
- “Mustererkennung” / Pattern Recognition
- Lecture (4 SWS): 2022
- “Visuelle Objekterkennung” / Visual Object Recognition
- Lecture (2 SWS): 2024, 2022, 2021, 2020, 2019
- “Informatik I (B.Sc. Physik)” / Computer Science I for Physicists
- Lecture (2 SWS): 2024, 2022, 2021, 2020, 2019
- Exercise (1 SWS): 2020, 2019
Supervised Theses
- Felix Kleinsteuber: “Anomaly Detection in Camera Trap Images”. Bachelor Thesis, 2022. (joint supervision with Daphne Auer)
- Philip Fürste: “Detection of Wildlife in Camera Trap Images using Transformers”. Master Thesis, 2022. (joint supervision with Gideon Stein)
- Eric Tröbs: “Klassenhierarchien im lebenslangen Lernen” (“Class Hierarchies in Lifelong Learning”). Master Thesis, 2021. (joint supervision with Clemens-Alexander Brust and Dimitri Korsch)
- Jan Blunk: “Object Tracking in Wildlife Identification”. Bachelor Thesis, 2021. (joint supervision with Matthias Körschens)
- Daphne Auer: “Applying Wavelet Transforms prior to Convolutional Neural Networks for Image Categorization”. Bachelor Thesis, 2020.
- Hendrik Happe: “Aktivierungsfunktionen in neuronalen Netzen für die visuelle Objekterkennung” (“Activation Functions in Neural Networks for Visual Object Recognition”). Master Thesis, 2020. (joint supervision with Christian Reimers)
- Frank Prüfer: “Detektion von unbekannten Objekten durch Entscheidungswälder und Gauß-Prozesse” (“Detecting Unknown Objects with Decision Forests and Gaussian Processes”). Diploma Thesis, 2013.
- Sven Sickert: “Strategien des Aktiven Lernens” (“Strategies in Active Learning”). Diploma Thesis, 2012. (joint supervision with Alexander Freytag)
Publications
2025
Aishwarya Venkataramanan, Paul Bodesheim, Joachim Denzler:
Probabilistic Embeddings for Frozen Vision-Language Models: Uncertainty Quantification with Gaussian Process Latent Variable Models.
International Conference on Uncertainty in Artificial Intelligence (UAI). 2025. (accepted)
[bibtex] [doi] [abstract]
Probabilistic Embeddings for Frozen Vision-Language Models: Uncertainty Quantification with Gaussian Process Latent Variable Models.
International Conference on Uncertainty in Artificial Intelligence (UAI). 2025. (accepted)
[bibtex] [doi] [abstract]
Vision-Language Models (VLMs) learn joint representations by mapping images and text into a shared latent space. However, recent research highlights that deterministic embeddings from standard VLMs often struggle to capture the uncertainties arising from the ambiguities in visual and textual descriptions and the multiple possible correspondences between images and texts. Existing approaches tackle this by learning probabilistic embeddings during VLM training, which demands large datasets and does not leverage the powerful representations already learned by large-scale VLMs like CLIP. In this paper, we propose GroVE, a post-hoc approach to obtaining probabilistic embeddings from frozen VLMs. GroVE builds on Gaussian Process Latent Variable Model (GPLVM) to learn a shared low-dimensional latent space where image and text inputs are mapped to a unified representation, optimized through single-modal embedding reconstruction and cross-modal alignment objectives. Once trained, the Gaussian Process model generates uncertainty-aware probabilistic embeddings. Evaluation shows that GroVE achieves state-of-the-art uncertainty calibration across multiple downstream tasks, including cross-modal retrieval, visual question answering, and active learning.
Kim Bjerge, Paul Bodesheim, Henrik Karstoft:
Deep Image Clustering with Model-Agnostic Meta-Learning.
International Joint Conference on Computer Vision, Imaging and Computer Graphics Theory and Applications (VISAPP). Pages 286-297. 2025.
[bibtex] [pdf] [doi] [abstract]
Deep Image Clustering with Model-Agnostic Meta-Learning.
International Joint Conference on Computer Vision, Imaging and Computer Graphics Theory and Applications (VISAPP). Pages 286-297. 2025.
[bibtex] [pdf] [doi] [abstract]
Deep clustering has proven successful in analyzing complex, high-dimensional real-world data. Typically, features are extracted from a deep neural network and then clustered. However, training the network to extract features that can be clustered efficiently in a semantically meaningful way is particularly challenging when data is sparse. In this paper, we present a semi-supervised method to fine-tune a deep learning network using Model-Agnostic Meta-Learning, commonly employed in Few-Shot Learning. We apply episodic training with a novel multivariate scatter loss, designed to enhance inter-class feature separation while minimizing intra-class variance, thereby improving overall clustering performance. Our approach works with state-of-the-art deep learning models, spanning convolutional neural networks and vision transformers, as well as different clustering algorithms like K-means and Spectral clustering. The effectiveness of our method is tested on several commonly used Few-Shot Le arning datasets, where episodic fine-tuning with our multivariate scatter loss and a ConvNeXt backbone outperforms other models, achieving adjusted rand index scores of 89.7% on the EU moths dataset and 86.9% on the Caltech birds dataset, respectively. Hence, our proposed method can be applied across various practical domains, such as clustering images of animal species in biology.
Tom August, Mario V Balzan, Paul Bodesheim, Gunnar Brehm, Lisette Cantú-Salazar, Sílvia Castro, Joseph Chipperfield, Guillaume Ghisbain, Alba Gomez-Segura, Jérémie Goulnik, Quentin Groom, Laurens Hogeweg, Chantal Huijbers, Andreas Kamilaris, Karolis Kazlauskis, Wouter Koch, Dimitri Korsch, João Loureiro, Youri Martin, Angeliki F Martinou, Kent McFarland, Xavier Mestdagh, Denis Michez, Charlie Outhwaite, Luca Pegoraro, Nadja Pernat, Lars B. Pettersson, Pavel Pipek, Cristina Preda, David Rolnick, Tobias Roth, David B. Roy, Helen Roy, Veljo Runnel, Martina Sasic, Dmitry Schigel, Julie Koch Sheard, Cecilie Svenningsen, Heliana Teixeira, Nicolas Titeux, Thomas Tscheulin, Elli Tzirkalli, Marijn van der Velde, Roel van Klink, Nicolas J Vereecken, Sarah Vray, Toke Thomas Høye:
Using Image-based AI for Insect Monitoring and Conservation - InsectAI COST Action.
Research Ideas and Outcomes. 11 : pp. e134825. 2025.
[bibtex] [pdf] [doi] [abstract]
Using Image-based AI for Insect Monitoring and Conservation - InsectAI COST Action.
Research Ideas and Outcomes. 11 : pp. e134825. 2025.
[bibtex] [pdf] [doi] [abstract]
The InsectAI COST action will support insect monitoring and conservation at the national and continental scale in order to understand and counteract widespread insect declines. The Action will bring together a critical mass of researchers and stakeholders in image-based insect AI technologies to direct and drive the research agenda, build research capacity across Europe and support innovation and application.There is mounting evidence that populations of insects around the world are in sharp decline. Understanding trends in species and their drivers is key to knowing the size of the challenge, its causes and how to address it. To identify solutions that lead to sustainable biodiversity alongside economic prosperity, insect monitoring should be efficient and provide standardised and frequently updated status indicators to guide conservation actions.The EU Biodiversity Strategy 2030 identifies the critical challenge of delivering standardised information about the state of nature and image-based insect AI can contribute to this. Specifically, the EU Nature Restoration Law will likely set binding targets for the high resolution data that cameras can provide. Thus, outputs of the Action will contribute directly to EU policies implementation, where biodiversity monitoring is considered a key component.The InsectAI COST Action will organise workshops, conferences, short-term scientific missions, hackathons, design-sprints and much more, across four Working Groups. These groups will address how image-based insect AI technologies can best address Societal Needs, support innovation in Image Collection hardware, create standardised approaches for Image Processing and develop novel Data Analysis and Integration methods for turning data into actionable insights.
Vivian Holzhauer, Dennis Böttger, Paul Bodesheim, Gunnar Brehm:
Do Camera Light Traps for Moths Provide Similar Data as Conventional Funnel Light Traps?.
bioRxiv. pp. 2025.02.06.636905. 2025.
[bibtex] [pdf] [doi] [abstract]
Do Camera Light Traps for Moths Provide Similar Data as Conventional Funnel Light Traps?.
bioRxiv. pp. 2025.02.06.636905. 2025.
[bibtex] [pdf] [doi] [abstract]
Insects are of crucial importance for terrestrial ecosystems but many populations decline rapidly. Conventional collecting methods are usually time-consuming, resulting in a low temporal, spatial and taxonomic resolution of data. Automated camera light traps (CLT) allow non-lethal monitoring of species-rich moths (Lepidoptera) and other nocturnal insects, but so far little is known about their performance compared to conventional collecting methods.By observing the behaviour of moths in previous field work, we hypothesized that CLT perform well in moth groups in which species tend to sit down quietly after approaching the lamp (such as Geometridae) but worse in moth groups in which species are persistently active (such as Sphingidae).We tested the performance of two CLTs, equipped with Sony alpha 7II (24 megapixel sensor) cameras that resulted in images with approx. 420 dpi resolution. The study was carried out in a forested area near Bielefeld in NW Germany for 196 nights in a row from April to September 2023, and photos were taken every two minutes during the night. All macromoths recognizable in the photos were identified and counted individually. We directly compared the data from the CLTs with moth samples obtained from conventional funnel light traps (FLTs) during 12 nights which were spread across the flight season.The resulting 420 dpi images from the CLTs allowed reliable species identification of all observed macromoths with only few exceptions due to technical problems. In direct comparison during 12 nights, CLTs recorded 39 species exclusively, FLTs recorded 48 species exclusively, and 53 species were recorded by both methods equally. During the whole sampling period of 196 nights in a row, a total of 225 moth species were recorded by CLTs. CLTs tended to record Geometridae better than FLTs whereas Sphingidae tended to be undersampled by the new method. Familes differed in the length to which they remained on the screen of the CLTs.Our study is the first to systematically compare the methods and it shows that CLTs perform overall very well. Results from CLTs differ to a certain extent from conventional trapping methods because they seem to perform worse in groups with highly active species and perform better in calmer groups like geometrid moths. CLTs are promising devices for insect monitoring since they deliver data with high resolution in time, space and taxonomy. The use of artificial intelligence (AI) for the analysis of images is intended as the next logic step.Competing Interest StatementThe authors have declared no competing interest.
2024
Kim Bjerge, Paul Bodesheim, Henrik Karstoft:
Few-Shot Learning with Novelty Detection.
International Conference on Deep Learning Theory and Applications (DeLTA). Pages 340-363. 2024. Best Paper Award
[bibtex] [pdf] [web] [doi] [abstract]
Few-Shot Learning with Novelty Detection.
International Conference on Deep Learning Theory and Applications (DeLTA). Pages 340-363. 2024. Best Paper Award
[bibtex] [pdf] [web] [doi] [abstract]
Machine learning has achieved considerable success in data-intensive applications, yet encounters challenges when confronted with small datasets. Recently, few-shot learning (FSL) has emerged as a promising solution to address this limitation. By leveraging prior knowledge, FSL exhibits the ability to swiftly generalize to new tasks, even when presented with only a handful of samples in an accompanied support set. This paper extends the scope of few-shot learning by incorporating novelty detection for samples of categories not present in the support set of FSL. This extension holds substantial promise for real-life applications where the availability of samples for each class is either sparse or absent. Our approach involves adapting existing FSL methods with a cosine similarity function, complemented by the learning of a probabilistic threshold to distinguish between known and outlier classes. During episodic training with domain generalization, we introduce a scatter loss function designed to disentangle the distribution of similarities between known and outlier classes, thereby enhancing the separation of novel and known classes. The efficacy of the proposed method is evaluated on commonly used FSL datasets and the EU Moths dataset characterized by few samples. Our experimental results showcase accuracy, ranging from 95.4\% to 96.7\%, as demonstrated on the Omniglot dataset through few-shot-novelty learning (FSNL). This high accuracy is observed across scenarios with 5 to 30 classes and the introduction of novel classes in each query set, underscoring the robustness and versatility of our proposed approach.
Matthias Körschens, Solveig Franziska Bucher, Paul Bodesheim, Josephine Ulrich, Joachim Denzler, Christine Römermann:
Determining the Community Composition of Herbaceous Species from Images using Convolutional Neural Networks.
Ecological Informatics. 80 : pp. 102516. 2024.
[bibtex] [pdf] [web] [doi] [abstract]
Determining the Community Composition of Herbaceous Species from Images using Convolutional Neural Networks.
Ecological Informatics. 80 : pp. 102516. 2024.
[bibtex] [pdf] [web] [doi] [abstract]
Global change has a detrimental impact on the environment and changes biodiversity patterns, which can be observed, among others, via analyzing changes in the composition of plant communities. Typically, vegetation relevées are done manually, which is time-consuming, laborious, and subjective. Applying an automatic system for such an analysis that can also identify co-occurring species would be beneficial as it is fast, effortless to use, and consistent. Here, we introduce such a system based on Convolutional Neural Networks for automatically predicting the species-wise plant cover. The system is trained on freely available image data of herbaceous plant species from web sources and plant cover estimates done by experts. With a novel extension of our original approach, the system can even be applied directly to vegetation images without requiring such cover estimates. Our extended approach, not utilizing dedicated training data, performs similarly to humans concerning the relative species abundances in the vegetation relevées. When trained on dedicated training annotations, it reflects the original estimates more closely than (independent) human experts, who manually analyzed the same sites. Our method is, with little adaptation, usable in novel domains and could be used to analyze plant community dynamics and responses of different plant species to environmental changes.
2023
Jan Blunk, Niklas Penzel, Paul Bodesheim, Joachim Denzler:
Beyond Debiasing: Actively Steering Feature Selection via Loss Regularization.
DAGM German Conference on Pattern Recognition (DAGM-GCPR). Pages 394-408. 2023.
[bibtex] [pdf] [doi] [abstract]
Beyond Debiasing: Actively Steering Feature Selection via Loss Regularization.
DAGM German Conference on Pattern Recognition (DAGM-GCPR). Pages 394-408. 2023.
[bibtex] [pdf] [doi] [abstract]
It is common for domain experts like physicians in medical studies to examine features for their reliability with respect to a specific domain task. When introducing machine learning, a common expectation is that machine learning models use the same features as human experts to solve a task but that is not always the case. Moreover, datasets often contain features that are known from domain knowledge to generalize badly to the real world, referred to as biases. Current debiasing methods only remove such influences. To additionally integrate the domain knowledge about well-established features into the training of a model, their relevance should be increased. We present a method that permits the manipulation of the relevance of features by actively steering the model's feature selection during the training process. That is, it allows both the discouragement of biases and encouragement of well-established features to incorporate domain knowledge about the feature reliability. We model our objectives for actively steering the feature selection process as a constrained optimization problem, which we implement via a loss regularization that is based on batch-wise feature attributions. We evaluate our approach on a novel synthetic regression dataset and a computer vision dataset. We observe that it successfully steers the features a model selects during the training process. This is a strong indicator that our method can be used to integrate domain knowledge about well-established features into a model.
2022
Dimitri Korsch, Paul Bodesheim, Gunnar Brehm, Joachim Denzler:
Automated Visual Monitoring of Nocturnal Insects with Light-based Camera Traps.
CVPR Workshop on Fine-grained Visual Classification (CVPR-WS). 2022.
[bibtex] [pdf] [web] [code] [abstract]
Automated Visual Monitoring of Nocturnal Insects with Light-based Camera Traps.
CVPR Workshop on Fine-grained Visual Classification (CVPR-WS). 2022.
[bibtex] [pdf] [web] [code] [abstract]
Automatic camera-assisted monitoring of insects for abundance estimations is crucial to understand and counteract ongoing insect decline. In this paper, we present two datasets of nocturnal insects, especially moths as a subset of Lepidoptera, photographed in Central Europe. One of the datasets, the EU-Moths dataset, was captured manually by citizen scientists and contains species annotations for 200 different species and bounding box annotations for those. We used this dataset to develop and evaluate a two-stage pipeline for insect detection and moth species classification in previous work. We further introduce a prototype for an automated visual monitoring system. This prototype produced the second dataset consisting of more than 27000 images captured on 95 nights. For evaluation and bootstrapping purposes, we annotated a subset of the images with bounding boxes enframing nocturnal insects. Finally, we present first detection and classification baselines for these datasets and encourage other scientists to use this publicly available data.
J. Wolfgang Wägele, Paul Bodesheim, Sarah J. Bourlat, Joachim Denzler, Michael Diepenbroek, Vera Fonseca, Karl-Heinz Frommolt, Matthias F. Geiger, Birgit Gemeinholzer, Frank Oliver Glöckner, Timm Haucke, Ameli Kirse, Alexander Kölpin, Ivaylo Kostadinov, Hjalmar S. Kühl, Frank Kurth, Mario Lasseck, Sascha Liedke, Florian Losch, Sandra Müller, Natalia Petrovskaya, Krzysztof Piotrowski, Bernd Radig, Christoph Scherber, Lukas Schoppmann, Jan Schulz, Volker Steinhage, Georg F. Tschan, Wolfgang Vautz, Domenico Velotto, Maximilian Weigend, Stefan Wildermann:
Towards a multisensor station for automated biodiversity monitoring.
Basic and Applied Ecology. 59 : pp. 105-138. 2022.
[bibtex] [pdf] [web] [doi] [abstract]
Towards a multisensor station for automated biodiversity monitoring.
Basic and Applied Ecology. 59 : pp. 105-138. 2022.
[bibtex] [pdf] [web] [doi] [abstract]
Rapid changes of the biosphere observed in recent years are caused by both small and large scale drivers, like shifts in temperature, transformations in land-use, or changes in the energy budget of systems. While the latter processes are easily quantifiable, documentation of the loss of biodiversity and community structure is more difficult. Changes in organismal abundance and diversity are barely documented. Censuses of species are usually fragmentary and inferred by often spatially, temporally and ecologically unsatisfactory simple species lists for individual study sites. Thus, detrimental global processes and their drivers often remain unrevealed. A major impediment to monitoring species diversity is the lack of human taxonomic expertise that is implicitly required for large-scale and fine-grained assessments. Another is the large amount of personnel and associated costs needed to cover large scales, or the inaccessibility of remote but nonetheless affected areas. To overcome these limitations we propose a network of Automated Multisensor stations for Monitoring of species Diversity (AMMODs) to pave the way for a new generation of biodiversity assessment centers. This network combines cutting-edge technologies with biodiversity informatics and expert systems that conserve expert knowledge. Each AMMOD station combines autonomous samplers for insects, pollen and spores, audio recorders for vocalizing animals, sensors for volatile organic compounds emitted by plants (pVOCs) and camera traps for mammals and small invertebrates. AMMODs are largely self-containing and have the ability to pre-process data (e.g. for noise filtering) prior to transmission to receiver stations for storage, integration and analyses. Installation on sites that are difficult to access require a sophisticated and challenging system design with optimum balance between power requirements, bandwidth for data transmission, required service, and operation under all environmental conditions for years. An important prerequisite for automated species identification are databases of DNA barcodes, animal sounds, for pVOCs, and images used as training data for automated species identification. AMMOD stations thus become a key component to advance the field of biodiversity monitoring for research and policy by delivering biodiversity data at an unprecedented spatial and temporal resolution.
Matthias Körschens, Paul Bodesheim, Joachim Denzler:
Beyond Global Average Pooling: Alternative Feature Aggregations for Weakly Supervised Localization.
International Conference on Computer Vision Theory and Applications (VISAPP). Pages 180-191. 2022.
[bibtex] [pdf] [doi] [abstract]
Beyond Global Average Pooling: Alternative Feature Aggregations for Weakly Supervised Localization.
International Conference on Computer Vision Theory and Applications (VISAPP). Pages 180-191. 2022.
[bibtex] [pdf] [doi] [abstract]
Weakly supervised object localization (WSOL) enables the detection and segmentation of objects in applications where localization annotations are hard or too expensive to obtain. Nowadays, most relevant WSOL approaches are based on class activation mapping (CAM), where a classification network utilizing global average pooling is trained for object classification. The classification layer that follows the pooling layer is then repurposed to generate segmentations using the unpooled features. The resulting localizations are usually imprecise and primarily focused around the most discriminative areas of the object, making a correct indication of the object location difficult. We argue that this problem is inherent in training with global average pooling due to its averaging operation. Therefore, we investigate two alternative pooling strategies: global max pooling and global log-sum-exp pooling. Furthermore, to increase the crispness and resolution of localization maps, we also investigate the application of Feature Pyramid Networks, which are commonplace in object detection. We confirm the usefulness of both alternative pooling methods as well as the Feature Pyramid Network on the CUB-200-2011 and OpenImages datasets.
Matthias Körschens, Paul Bodesheim, Joachim Denzler:
Occlusion-Robustness of Convolutional Neural Networks via Inverted Cutout.
International Conference on Pattern Recognition (ICPR). Pages 2829-2835. 2022.
[bibtex] [pdf] [doi] [supplementary] [abstract]
Occlusion-Robustness of Convolutional Neural Networks via Inverted Cutout.
International Conference on Pattern Recognition (ICPR). Pages 2829-2835. 2022.
[bibtex] [pdf] [doi] [supplementary] [abstract]
Convolutional Neural Networks (CNNs) are able to reliably classify objects in images if they are clearly visible and only slightly affected by small occlusions. However, heavy occlusions can strongly deteriorate the performance of CNNs, which is critical for tasks where correct identification is paramount. For many real-world applications, images are taken in unconstrained environments under suboptimal conditions, where occluded objects are inevitable. We propose a novel data augmentation method called Inverted Cutout, which can be used for training a CNN by showing only small patches of the images. Together with this augmentation method, we present several ways of making the network robust against occlusion. On the one hand, we utilize a spatial aggregation module without modifying the base network and on the other hand, we achieve occlusion-robustness with appropriate fine-tuning in conjunction with Inverted Cutout. In our experiments, we compare two different aggregation modules and two loss functions on the Occluded-Vehicles and Occluded-COCO-Vehicles datasets, showing that our approach outperforms existing state-of-the-art methods for object categorization under varying levels of occlusion.
Niklas Penzel, Christian Reimers, Paul Bodesheim, Joachim Denzler:
Investigating Neural Network Training on a Feature Level using Conditional Independence.
ECCV Workshop on Causality in Vision (ECCV-WS). Pages 383-399. 2022.
[bibtex] [pdf] [doi] [abstract]
Investigating Neural Network Training on a Feature Level using Conditional Independence.
ECCV Workshop on Causality in Vision (ECCV-WS). Pages 383-399. 2022.
[bibtex] [pdf] [doi] [abstract]
There are still open questions about how the learned representations of deep models change during the training process. Understanding this process could aid in validating the training. Towards this goal, previous works analyze the training in the mutual information plane. We use a different approach and base our analysis on a method built on Reichenbach’s common cause principle. Using this method, we test whether the model utilizes information contained in human-defined features. Given such a set of features, we investigate how the relative feature usage changes throughout the training process. We analyze mul- tiple networks training on different tasks, including melanoma classifica- tion as a real-world application. We find that over the training, models concentrate on features containing information relevant to the task. This concentration is a form of representation compression. Crucially, we also find that the selected features can differ between training from-scratch and finetuning a pre-trained network.
Paul Bodesheim, Flurin Babst, David C. Frank, Claudia Hartl, Christian S. Zang, Martin Jung, Markus Reichstein, Miguel D. Mahecha:
Predicting spatiotemporal variability in radial tree growth at the continental scale with machine learning.
Environmental Data Science. 1 : pp. E9. 2022.
[bibtex] [pdf] [doi] [abstract]
Predicting spatiotemporal variability in radial tree growth at the continental scale with machine learning.
Environmental Data Science. 1 : pp. E9. 2022.
[bibtex] [pdf] [doi] [abstract]
Tree-ring chronologies encode interannual variability in forest growth rates over long time periods from decades to centuries or even millennia. However, each chronology is a highly localized measurement describing conditions at specific sites where wood samples have been collected. The question whether these local growth variabilites are representative for large geographical regions remains an open issue. To overcome the limitations of interpreting a sparse network of sites, we propose an upscaling approach for annual tree-ring indices that approximate forest growth variability and compute gridded data products that generalize the available information for multiple tree genera. Using regression approaches from machine learning, we predict tree-ring indices in space and time based on climate variables, but considering also species range maps as constraints for the upscaling. We compare various prediction strategies in cross-validation experiments to identify the best performing setup. Our estimated maps of tree-ring indices are the first data products that provide a dense view on forest growth variability at the continental level with 0.5° and 0.0083° spatial resolution covering the years 1902–2013. Furthermore, we find that different genera show very variable spatial patterns of anomalies. We have selected Europe as study region and focused on the six most prominent tree genera, but our approach is very generic and can easily be applied elsewhere. Overall, the study shows perspectives but also limitations for reconstructing spatiotemporal dynamics of complex biological processes. The data products are available at \urlhttps://www.doi.org/10.17871/BACI.248.
Paul Bodesheim, Jan Blunk, Matthias Körschens, Clemens-Alexander Brust, Christoph Käding, Joachim Denzler:
Pre-trained models are not enough: active and lifelong learning is important for long-term visual monitoring of mammals in biodiversity research. Individual identification and attribute prediction with image features from deep neural networks and decoupled decision models applied to elephants and great apes.
Mammalian Biology. 102 : pp. 875-897. 2022.
[bibtex] [pdf] [web] [doi] [abstract]
Pre-trained models are not enough: active and lifelong learning is important for long-term visual monitoring of mammals in biodiversity research. Individual identification and attribute prediction with image features from deep neural networks and decoupled decision models applied to elephants and great apes.
Mammalian Biology. 102 : pp. 875-897. 2022.
[bibtex] [pdf] [web] [doi] [abstract]
Animal re-identification based on image data, either recorded manually by photographers or automatically with camera traps, is an important task for ecological studies about biodiversity and conservation that can be highly automatized with algorithms from computer vision and machine learning. However, fixed identification models only trained with standard datasets before their application will quickly reach their limits, especially for long-term monitoring with changing environmental conditions, varying visual appearances of individuals over time that differ a lot from those in the training data, and new occurring individuals that have not been observed before. Hence, we believe that active learning with human-in-the-loop and continuous lifelong learning is important to tackle these challenges and to obtain high-performance recognition systems when dealing with huge amounts of additional data that become available during the application. Our general approach with image features from deep neural networks and decoupled decision models can be applied to many different mammalian species and is perfectly suited for continuous improvements of the recognition systems via lifelong learning. In our identification experiments, we consider four different taxa, namely two elephant species: African forest elephants and Asian elephants, as well as two species of great apes: gorillas and chimpanzees. Going beyond classical re-identification, our decoupled approach can also be used for predicting attributes of individuals such as gender or age using classification or regression methods. Although applicable for small datasets of individuals as well, we argue that even better recognition performance will be achieved by improving decision models gradually via lifelong learning to exploit huge datasets and continuous recordings from long-term applications. We highlight that algorithms for deploying lifelong learning in real observational studies exist and are ready for use. Hence, lifelong learning might become a valuable concept that supports practitioners when analyzing large-scale image data during long-term monitoring of mammals.
Roel van Klink, Tom August, Yves Bas, Paul Bodesheim, Aletta Bonn, Frode Fossoy, Toke T. Hoye, Eelke Jongejans, Myles H.M. Menz, Andreia Miraldo, Tomas Roslin, Helen E. Roy, Ireneusz Ruczynski, Dmitry Schigel, Livia Schäffler, Julie K. Sheard, Cecilie Svenningsen, Georg F. Tschan, Jana Wäldchen, Vera M.A. Zizka, Jens Aström, Diana E. Bowler:
Emerging technologies revolutionise insect ecology and monitoring.
Trends in Ecology \& Evolution. 37 (10) : pp. 872-885. 2022.
[bibtex] [pdf] [doi] [abstract]
Emerging technologies revolutionise insect ecology and monitoring.
Trends in Ecology \& Evolution. 37 (10) : pp. 872-885. 2022.
[bibtex] [pdf] [doi] [abstract]
Insects are the most diverse group of animals on Earth, but their small size and high diversity have always made them challenging to study. Recent technological advances have the potential to revolutionise insect ecology and monitoring. We describe the state of the art of four technologies (computer vision, acoustic monitoring, radar, and molecular methods), and assess their advantages, current limitations, and future potential. We discuss how these technologies can adhere to modern standards of data curation and transparency, their implications for citizen science, and their potential for integration among different monitoring programmes and technologies. We argue that they provide unprecedented possibilities for insect ecology and monitoring, but it will be important to foster international standards via collaboration.
2021
Bernd Radig, Paul Bodesheim, Dimitri Korsch, Joachim Denzler, Timm Haucke, Morris Klasen, Volker Steinhage:
Automated Visual Large Scale Monitoring of Faunal Biodiversity.
Pattern Recognition and Image Analysis. Advances in Mathematical Theory and Applications (PRIA). 31 (3) : pp. 477-488. 2021.
[bibtex] [pdf] [web] [doi] [abstract]
Automated Visual Large Scale Monitoring of Faunal Biodiversity.
Pattern Recognition and Image Analysis. Advances in Mathematical Theory and Applications (PRIA). 31 (3) : pp. 477-488. 2021.
[bibtex] [pdf] [web] [doi] [abstract]
To observe biodiversity, the variety of plant and animal life in the world or in a particular habitat, human observers make the most common examinations, often assisted by technical equipment. Measuring objectively the number of different species of animals, plants, fungi, and microbes that make up the ecosystem can be difficult. In order to monitor changes in biodiversity, data have to be compared across space and time. Cameras are an essential sensor to determine the species range, abundance, and behavior of animals. The millions of recordings from camera traps set up in natural environments can no longer be analyzed by biologists. We started research on doing this analysis automatically without human interaction. The focus of our present sensor is on image capture of wildlife and moths. Special hardware elements for the detection of different species are designed, implemented, tested, and improved, as well as the algorithms for classification and counting of samples from images and image sequences, e.g., to calculate presence, absence, and abundance values or the duration of characteristic activities related to the spatial mobilities. For this purpose, we are developing stereo camera traps that allow spatial reconstruction of the observed animals. This allows three-dimensional coordinates to be recorded and the shape to be characterized. With this additional feature data, species identification and movement detection are facilitated. To classify and count moths, they are attracted to an illuminated screen, which is then photographed at intervals by a high-resolution color camera. To greatly reduce the volume of data, redundant elements and elements that are consistent from image to image are eliminated. All design decisions take into account that at remote sites and in fully autonomous operation, power supply on the one hand and possibilities for data exchange with central servers on the other hand are limited. Installation at hard-to-reach locations requires a sophisticated and demanding system design with an optimal balance between power requirements, bandwidth for data transmission, required service and operation in all environmental conditions for at least ten years.
Christian Reimers, Niklas Penzel, Paul Bodesheim, Jakob Runge, Joachim Denzler:
Conditional Dependence Tests Reveal the Usage of ABCD Rule Features and Bias Variables in Automatic Skin Lesion Classification.
CVPR ISIC Skin Image Analysis Workshop (CVPR-WS). Pages 1810-1819. 2021.
[bibtex] [pdf] [web] [abstract]
Conditional Dependence Tests Reveal the Usage of ABCD Rule Features and Bias Variables in Automatic Skin Lesion Classification.
CVPR ISIC Skin Image Analysis Workshop (CVPR-WS). Pages 1810-1819. 2021.
[bibtex] [pdf] [web] [abstract]
Skin cancer is the most common form of cancer, and melanoma is the leading cause of cancer related deaths. To improve the chances of survival, early detection of melanoma is crucial. Automated systems for classifying skin lesions can assist with initial analysis. However, if we expect people to entrust their well-being to an automatic classification algorithm, it is important to ensure that the algorithm makes medically sound decisions. We investigate this question by testing whether two state-of-the-art models use the features defined in the dermoscopic ABCD rule or whether they rely on biases. We use a method that frames supervised learning as a structural causal model, thus reducing the question whether a feature is used to a conditional dependence test. We show that this conditional dependence method yields meaningful results on data from the ISIC archive. Furthermore, we find that the selected models incorporate asymmetry, border and dermoscopic structures in their decisions but not color. Finally, we show that the same classifiers also use bias features such as the patient's age, skin color or the existence of colorful patches.
Christian Reimers, Paul Bodesheim, Jakob Runge, Joachim Denzler:
Conditional Adversarial Debiasing: Towards Learning Unbiased Classifiers from Biased Data.
DAGM German Conference on Pattern Recognition (DAGM-GCPR). Pages 48-62. 2021.
[bibtex] [pdf] [doi] [abstract]
Conditional Adversarial Debiasing: Towards Learning Unbiased Classifiers from Biased Data.
DAGM German Conference on Pattern Recognition (DAGM-GCPR). Pages 48-62. 2021.
[bibtex] [pdf] [doi] [abstract]
Bias in classifiers is a severe issue of modern deep learning methods, especially for their application in safety- and security-critical areas. Often, the bias of a classifier is a direct consequence of a bias in the training set, frequently caused by the co-occurrence of relevant features and irrelevant ones. To mitigate this issue, we require learning algorithms that prevent the propagation of known bias from the dataset into the classifier. We present a novel adversarial debiasing method, which addresses a feature of which we know that it is spuriously connected to the labels of training images but statistically independent of the labels for test images. The debiasing stops the classifier from falsly identifying this irrelevant feature as important. Irrelevant features co-occur with important features in a wide range of bias-related problems for many computer vision tasks, such as automatic skin cancer detection or driver assistance. We argue by a mathematical proof that our approach is superior to existing techniques for the abovementioned bias. Our experiments show that our approach performs better than the state-of-the-art on a well-known benchmark dataset with real-world images of cats and dogs.
Daphne Auer, Paul Bodesheim, Christian Fiderer, Marco Heurich, Joachim Denzler:
Minimizing the Annotation Effort for Detecting Wildlife in Camera Trap Images with Active Learning.
Computer Science for Biodiversity Workshop (CS4Biodiversity), INFORMATIK 2021. Pages 547-564. 2021.
[bibtex] [pdf] [doi] [abstract]
Minimizing the Annotation Effort for Detecting Wildlife in Camera Trap Images with Active Learning.
Computer Science for Biodiversity Workshop (CS4Biodiversity), INFORMATIK 2021. Pages 547-564. 2021.
[bibtex] [pdf] [doi] [abstract]
Analyzing camera trap images is a challenging task due to complex scene structures at different locations, heavy occlusions, and varying sizes of animals.One particular problem is the large fraction of images only showing background scenes, which are recorded when a motion detector gets triggered by signals other than animal movements.To identify these background images automatically, an active learning approach is used to train binary classifiers with small amounts of labeled data, keeping the annotation effort of humans minimal.By training classifiers for single sites or small sets of camera traps, we follow a region-based approach and particularly focus on distinct models for daytime and nighttime images.Our approach is evaluated on camera trap images from the Bavarian Forest National Park.Comparable or even superior performances to publicly available detectors trained with millions of labeled images are achieved while requiring significantly smaller amounts of annotated training images.
Dimitri Korsch, Paul Bodesheim, Joachim Denzler:
Deep Learning Pipeline for Automated Visual Moth Monitoring: Insect Localization and Species Classification.
INFORMATIK 2021, Computer Science for Biodiversity Workshop (CS4Biodiversity). Pages 443-460. 2021.
[bibtex] [pdf] [web] [doi] [code] [abstract]
Deep Learning Pipeline for Automated Visual Moth Monitoring: Insect Localization and Species Classification.
INFORMATIK 2021, Computer Science for Biodiversity Workshop (CS4Biodiversity). Pages 443-460. 2021.
[bibtex] [pdf] [web] [doi] [code] [abstract]
Biodiversity monitoring is crucial for tracking and counteracting adverse trends in population fluctuations. However, automatic recognition systems are rarely applied so far, and experts evaluate the generated data masses manually. Especially the support of deep learning methods for visual monitoring is not yet established in biodiversity research, compared to other areas like advertising or entertainment. In this paper, we present a deep learning pipeline for analyzing images captured by a moth scanner, an automated visual monitoring system of moth species developed within the AMMOD project. We first localize individuals with a moth detector and afterward determine the species of detected insects with a classifier. Our detector achieves up to 99.01% mean average precision and our classifier distinguishes 200 moth species with an accuracy of 93.13% on image cutouts depicting single insects. Combining both in our pipeline improves the accuracy for species identification in images of the moth scanner from 79.62% to 88.05%.
Dimitri Korsch, Paul Bodesheim, Joachim Denzler:
End-to-end Learning of Fisher Vector Encodings for Part Features in Fine-grained Recognition.
DAGM German Conference on Pattern Recognition (DAGM-GCPR). Pages 142-158. 2021.
[bibtex] [pdf] [web] [doi] [code] [abstract]
End-to-end Learning of Fisher Vector Encodings for Part Features in Fine-grained Recognition.
DAGM German Conference on Pattern Recognition (DAGM-GCPR). Pages 142-158. 2021.
[bibtex] [pdf] [web] [doi] [code] [abstract]
Part-based approaches for fine-grained recognition do not show the expected performance gain over global methods, although explicitly focusing on small details that are relevant for distinguishing highly similar classes. We assume that part-based methods suffer from a missing representation of local features, which is invariant to the order of parts and can handle a varying number of visible parts appropriately. The order of parts is artificial and often only given by ground-truth annotations, whereas viewpoint variations and occlusions result in not observable parts. Therefore, we propose integrating a Fisher vector encoding of part features into convolutional neural networks. The parameters for this encoding are estimated by an online EM algorithm jointly with those of the neural network and are more precise than the estimates of previous works. Our approach improves state-of-the-art accuracies for three bird species classification datasets.
Julia Böhlke, Dimitri Korsch, Paul Bodesheim, Joachim Denzler:
Exploiting Web Images for Moth Species Classification.
Computer Science for Biodiversity Workshop (CS4Biodiversity), INFORMATIK 2021. Pages 481-498. 2021.
[bibtex] [pdf] [web] [doi] [abstract]
Exploiting Web Images for Moth Species Classification.
Computer Science for Biodiversity Workshop (CS4Biodiversity), INFORMATIK 2021. Pages 481-498. 2021.
[bibtex] [pdf] [web] [doi] [abstract]
Due to shrinking habitats, moth populations are declining rapidly. An automated moth population monitoring tool is needed to support conservationists in making informed decisions for counteracting this trend. A non-invasive tool would involve the automatic classification of images of moths, a fine-grained recognition problem. Currently, the lack of images annotated by experts is the main hindrance to such a classification model. To understand how to achieve acceptable predictive accuracies, we investigate the effect of differently sized datasets and data acquired from the Internet. We find the use of web data immensely beneficial and observe that few images from the evaluation domain are enough to mitigate the domain shift in web data. Our experiments show that counteracting the domain shift may yield a relative reduction of the error rate of over 60\%. Lastly, the effect of label noise in web data and proposed filtering techniques are analyzed and evaluated.
Julia Böhlke, Dimitri Korsch, Paul Bodesheim, Joachim Denzler:
Lightweight Filtering of Noisy Web Data: Augmenting Fine-grained Datasets with Selected Internet Images.
International Conference on Computer Vision Theory and Applications (VISAPP). Pages 466-477. 2021.
[bibtex] [pdf] [web] [doi] [abstract]
Lightweight Filtering of Noisy Web Data: Augmenting Fine-grained Datasets with Selected Internet Images.
International Conference on Computer Vision Theory and Applications (VISAPP). Pages 466-477. 2021.
[bibtex] [pdf] [web] [doi] [abstract]
Despite the availability of huge annotated benchmark datasets and the potential of transfer learning, i.e., fine-tuning a pre-trained neural network to a specific task, deep learning struggles in applications where no labeled datasets of sufficient size exist. This issue affects fine-grained recognition tasks the most since correct image data annotations are expensive and require expert knowledge. Nevertheless, the Internet offers a lot of weakly annotated images. In contrast to existing work, we suggest a new lightweight filtering strategy to exploit this source of information without supervision and minimal additional costs. Our main contributions are specific filter operations that allow the selection of downloaded images to augment a training set. We filter test duplicates to avoid a biased evaluation of the methods, and two types of label noise: cross-domain noise, i.e., images outside any class in the dataset, and cross-class noise, a form of label-swapping noise. We evaluate our suggested filter operations in a controlled environment and demonstrate our methods' effectiveness with two small annotated seed datasets for moth species recognition. While noisy web images consistently improve classification accuracies, our filtering methoeds retain a fraction of the data such that high accuracies are achieved with a significantly smaller training dataset.
Matthias Körschens, Paul Bodesheim, Christine Römermann, Solveig Franziska Bucher, Mirco Migliavacca, Josephine Ulrich, Joachim Denzler:
Automatic Plant Cover Estimation with Convolutional Neural Networks.
Computer Science for Biodiversity Workshop (CS4Biodiversity), INFORMATIK 2021. Pages 499-516. 2021.
[bibtex] [pdf] [doi] [abstract]
Automatic Plant Cover Estimation with Convolutional Neural Networks.
Computer Science for Biodiversity Workshop (CS4Biodiversity), INFORMATIK 2021. Pages 499-516. 2021.
[bibtex] [pdf] [doi] [abstract]
Monitoring the responses of plants to environmental changes is essential for plant biodiversity research. This, however, is currently still being done manually by botanists in the field. This work is very laborious, and the data obtained is, though following a standardized method to estimate plant coverage, usually subjective and has a coarse temporal resolution. To remedy these caveats, we investigate approaches using convolutional neural networks (CNNs) to automatically extract the relevant data from images, focusing on plant community composition and species coverages of 9 herbaceous plant species. To this end, we investigate several standard CNN architectures and different pretraining methods. We find that we outperform our previous approach at higher image resolutions using a custom CNN with a mean absolute error of 5.16%. In addition to these investigations, we also conduct an error analysis based on the temporal aspect of the plant cover images. This analysis gives insight into where problems for automatic approaches lie, like occlusion and likely misclassifications caused by temporal changes.
Matthias Körschens, Paul Bodesheim, Christine Römermann, Solveig Franziska Bucher, Mirco Migliavacca, Josephine Ulrich, Joachim Denzler:
Weakly Supervised Segmentation Pretraining for Plant Cover Prediction.
DAGM German Conference on Pattern Recognition (DAGM-GCPR). Pages 589-603. 2021.
[bibtex] [pdf] [doi] [supplementary] [abstract]
Weakly Supervised Segmentation Pretraining for Plant Cover Prediction.
DAGM German Conference on Pattern Recognition (DAGM-GCPR). Pages 589-603. 2021.
[bibtex] [pdf] [doi] [supplementary] [abstract]
Automated plant cover prediction can be a valuable tool for botanists, as plant cover estimations are a laborious and recurring task in environmental research. Upon examination of the images usually encompassed in this task, it becomes apparent that the task is ill-posed and successful training on such images alone without external data is nearly impossible. While a previous approach includes pretraining on a domain-related dataset containing plants in natural settings, we argue that regular classification training on such data is insufficient. To solve this problem, we propose a novel pretraining pipeline utilizing weakly supervised object localization on images with only class annotations to generate segmentation maps that can be exploited for a second pretraining step. We utilize different pooling methods during classification pretraining, and evaluate and compare their effects on the plant cover prediction. For this evaluation, we focus primarily on the visible parts of the plants. To this end, contrary to previous works, we created a small dataset containing segmentations of plant cover images to be able to evaluate the benefit of our method numerically. We find that our segmentation pretraining approach outperforms classification pretraining and especially aids in the recognition of less prevalent plants in the plant cover dataset.
2020
Martin Jung, Christopher Schwalm, Mirco Migliavacca, Sophia Walther, Gustau Camps-Valls, Sujan Koirala, Peter Anthoni, Simon Besnard, Paul Bodesheim, Nuno Carvalhais, Frederic Chevallier, Fabian Gans, Daniel S. Goll, Vanessa Haverd, Philipp Köhler, Kazuhito Ichii, Atul K. Jain, Junzhi Liu, Danica Lombardozzi, Julia E.M.S. Nabel, Jacob A. Nelson, Michael O’Sullivan, Martijn Pallandt, Dario Papale, Wouter Peters, Julia Pongratz, Christian Rödenbeck, Stephen Sitch, Gianluca Tramontana, Anthony Walker, Ulrich Weber, Markus Reichstein:
Scaling carbon fluxes from eddy covariance sites to globe: synthesis and evaluation of the FLUXCOM approach.
Biogeosciences. 17 (5) : pp. 1343-1365. 2020.
[bibtex] [pdf] [web] [doi] [abstract]
Scaling carbon fluxes from eddy covariance sites to globe: synthesis and evaluation of the FLUXCOM approach.
Biogeosciences. 17 (5) : pp. 1343-1365. 2020.
[bibtex] [pdf] [web] [doi] [abstract]
FLUXNET comprises globally distributed eddy-covariance-based estimates of carbon fluxes between the biosphere and the atmosphere. Since eddy covariance flux towers have a relatively small footprint and are distributed unevenly across the world, upscaling the observations is necessary to obtain global-scale estimates of biosphere–atmosphere exchange. Based on cross-consistency checks with atmospheric inversions, sun-induced fluorescence (SIF) and dynamic global vegetation models (DGVMs), here we provide a systematic assessment of the latest upscaling efforts for gross primary production (GPP) and net ecosystem exchange (NEE) of the FLUXCOM initiative, where different machine learning methods, forcing data sets and sets of predictor variables were employed. Spatial patterns of mean GPP are consistent across FLUXCOM and DGVM ensembles (R2>0.94 at 1° spatial resolution) while the majority of DGVMs show, for 70 % of the land surface, values outside the FLUXCOM range. Global mean GPP magnitudes for 2008–2010 from FLUXCOM members vary within 106 and 130 PgC yr−1 with the largest uncertainty in the tropics. Seasonal variations in independent SIF estimates agree better with FLUXCOM GPP (mean global pixel-wise R2∼0.75) than with GPP from DGVMs (mean global pixel-wise R2∼0.6). Seasonal variations in FLUXCOM NEE show good consistency with atmospheric inversion-based net land carbon fluxes, particularly for temperate and boreal regions (R2>0.92). Interannual variability of global NEE in FLUXCOM is underestimated compared to inversions and DGVMs. The FLUXCOM version which also uses meteorological inputs shows a strong co-variation in interannual patterns with inversions (R2=0.87 for 2001–2010). Mean regional NEE from FLUXCOM shows larger uptake than inversion and DGVM-based estimates, particularly in the tropics with discrepancies of up to several hundred grammes of carbon per square metre per year. These discrepancies can only partly be reconciled by carbon loss pathways that are implicit in inversions but not captured by the flux tower measurements such as carbon emissions from fires and water bodies. We hypothesize that a combination of systematic biases in the underlying eddy covariance data, in particular in tall tropical forests, and a lack of site history effects on NEE in FLUXCOM are likely responsible for the too strong tropical carbon sink estimated by FLUXCOM. Furthermore, as FLUXCOM does not account for CO2 fertilization effects, carbon flux trends are not realistic. Overall, current FLUXCOM estimates of mean annual and seasonal cycles of GPP as well as seasonal NEE variations provide useful constraints of global carbon cycling, while interannual variability patterns from FLUXCOM are valuable but require cautious interpretation. Exploring the diversity of Earth observation data and of machine learning concepts along with improved quality and quantity of flux tower measurements will facilitate further improvements of the FLUXCOM approach overall.
Matthias Körschens, Paul Bodesheim, Christine Römermann, Solveig Franziska Bucher, Josephine Ulrich, Joachim Denzler:
Towards Confirmable Automated Plant Cover Determination.
ECCV Workshop on Computer Vision Problems in Plant Phenotyping (CVPPP). 2020.
[bibtex] [pdf] [web] [doi] [supplementary] [abstract]
Towards Confirmable Automated Plant Cover Determination.
ECCV Workshop on Computer Vision Problems in Plant Phenotyping (CVPPP). 2020.
[bibtex] [pdf] [web] [doi] [supplementary] [abstract]
Changes in plant community composition reflect environmental changes like in land-use and climate. While we have the means to record the changes in composition automatically nowadays, we still lack methods to analyze the generated data masses automatically. We propose a novel approach based on convolutional neural networks for analyzing the plant community composition while making the results explainable for the user. To realize this, our approach generates a semantic segmentation map while predicting the cover percentages of the plants in the community. The segmentation map is learned in a weakly supervised way only based on plant cover data and therefore does not require dedicated segmentation annotations. Our approach achieves a mean absolute error of 5.3% for plant cover prediction on our introduced dataset with 9 herbaceous plant species in an imbalanced distribution, and generates segmentation maps, where the location of the most prevalent plants in the dataset is correctly indicated in many images.
Miguel D. Mahecha, Fabian Gans, Gunnar Brandt, Rune Christiansen, Sarah E. Cornell, Normann Fomferra, Guido Kraemer, Jonas Peters, Paul Bodesheim, Gustau Camps-Valls, Jonathan F. Donges, Wouter Dorigo, Lina M. Estupinan-Suarez, Victor H. Gutierrez-Velez, Martin Gutwin, Martin Jung, Maria C. Londono, Diego Miralles, Phillip Papastefanou, Markus Reichstein:
Earth system data cubes unravel global multivariate dynamics.
Earth System Dynamics. 11 (1) : pp. 201-234. 2020.
[bibtex] [pdf] [web] [doi] [abstract]
Earth system data cubes unravel global multivariate dynamics.
Earth System Dynamics. 11 (1) : pp. 201-234. 2020.
[bibtex] [pdf] [web] [doi] [abstract]
Understanding Earth system dynamics in light of ongoing human intervention and dependency remains a major scientific challenge. The unprecedented availability of data streams describing different facets of the Earth now offers fundamentally new avenues to address this quest. However, several practical hurdles, especially the lack of data interoperability, limit the joint potential of these data streams. Today, many initiatives within and beyond the Earth system sciences are exploring new approaches to overcome these hurdles and meet the growing interdisciplinary need for data-intensive research; using data cubes is one promising avenue. Here, we introduce the concept of Earth system data cubes and how to operate on them in a formal way. The idea is that treating multiple data dimensions, such as spatial, temporal, variable, frequency, and other grids alike, allows effective application of user-defined functions to co-interpret Earth observations and/or model–data integration. An implementation of this concept combines analysis-ready data cubes with a suitable analytic interface. In three case studies, we demonstrate how the concept and its implementation facilitate the execution of complex workflows for research across multiple variables, and spatial and temporal scales: (1) summary statistics for ecosystem and climate dynamics; (2) intrinsic dimensionality analysis on multiple timescales; and (3) model–data integration. We discuss the emerging perspectives for investigating global interacting and coupled phenomena in observed or simulated data. In particular, we see many emerging perspectives of this approach for interpreting large-scale model ensembles. The latest developments in machine learning, causal inference, and model–data integration can be seamlessly implemented in the proposed framework, supporting rapid progress in data-intensive research across disciplinary boundaries.
2019
Dimitri Korsch, Paul Bodesheim, Joachim Denzler:
Classification-Specific Parts for Improving Fine-Grained Visual Categorization.
DAGM German Conference on Pattern Recognition (DAGM-GCPR). Pages 62-75. 2019.
[bibtex] [pdf] [web] [doi] [code] [abstract]
Classification-Specific Parts for Improving Fine-Grained Visual Categorization.
DAGM German Conference on Pattern Recognition (DAGM-GCPR). Pages 62-75. 2019.
[bibtex] [pdf] [web] [doi] [code] [abstract]
Fine-grained visual categorization is a classification task for distinguishing categories with high intra-class and small inter-class variance. While global approaches aim at using the whole image for performing the classification, part-based solutions gather additional local information in terms of attentions or parts. We propose a novel classification-specific part estimation that uses an initial prediction as well as back-propagation of feature importance via gradient computations in order to estimate relevant image regions. The subsequently detected parts are then not only selected by a-posteriori classification knowledge, but also have an intrinsic spatial extent that is determined automatically. This is in contrast to most part-based approaches and even to available ground-truth part annotations, which only provide point coordinates and no additional scale information. We show in our experiments on various widely-used fine-grained datasets the effectiveness of the mentioned part selection method in conjunction with the extracted part features.
2018
Flurin Babst, Paul Bodesheim, Noah Charney, Andrew D. Friend, Martin P. Girardin, Stefan Klesse, David J.P. Moore, Kristina Seftigen, Jesper Björklund, Olivier Bouriaud, Andria Dawson, R. Justin DeRose, Michael C. Dietze, Annemarie H. Eckes, Brian Enquist, David C. Frank, Miguel D. Mahecha, Benjamin Poulter, Sydne Record, Valerie Trouet, Rachael H. Turton, Zhen Zhang, Margaret E.K. Evans:
When tree rings go global: challenges and opportunities for retro-and prospective insight.
Quaternary Science Reviews. 197 : pp. 1-20. 2018.
[bibtex] [pdf] [web] [doi] [abstract]
When tree rings go global: challenges and opportunities for retro-and prospective insight.
Quaternary Science Reviews. 197 : pp. 1-20. 2018.
[bibtex] [pdf] [web] [doi] [abstract]
The demand for large-scale and long-term information on tree growth is increasing rapidly as environmental change research strives to quantify and forecast the impacts of continued warming on forest ecosystems. This demand, combined with the now quasi-global availability of tree-ring observations, has inspired researchers to compile large tree-ring networks to address continental or even global-scale research questions. However, these emergent spatial objectives contrast with paleo-oriented research ideas that have guided the development of many existing records. A series of challenges related to how, where, and when samples have been collected is complicating the transition of tree rings from a local to a global resource on the question of tree growth. Herein, we review possibilities to scale tree-ring data (A) from the sample to the whole tree, (B) from the tree to the site, and (C) from the site to larger spatial domains. Representative tree-ring sampling supported by creative statistical approaches is thereby key to robustly capture the heterogeneity of climate-growth responses across forested landscapes. We highlight the benefits of combining the temporal information embedded in tree rings with the spatial information offered by forest inventories and earth observations to quantify tree growth and its drivers. In addition, we show how the continued development of mechanistic tree-ring models can help address some of the non-linearities and feedbacks that complicate making inference from tree-ring data. By embracing scaling issues, the discipline of dendrochronology will greatly increase its contributions to assessing climate impacts on forests and support the development of adaptation strategies.
Gaia Vaglio Laurin, Cristina Vittucci, Gianluca Tramontana, Paul Bodesheim, Paolo Ferrazzoli, Leila Guerriero, Martin Jung, Miguel D. Mahecha, Dario Papale:
SMOS Vegetation Optical Depth and Ecosystem Functional Properties: Exploring Their Relationships in Tropical Forests.
IEEE International Geoscience and Remote Sensing Symposium (IGARSS). Pages 5891-5894. 2018.
[bibtex] [pdf] [web] [doi] [abstract]
SMOS Vegetation Optical Depth and Ecosystem Functional Properties: Exploring Their Relationships in Tropical Forests.
IEEE International Geoscience and Remote Sensing Symposium (IGARSS). Pages 5891-5894. 2018.
[bibtex] [pdf] [web] [doi] [abstract]
Data from the Soil Moisture and Ocean Salinity (SMOS) mission were exploited to explore its potential in providing ecosystem information. Here the strength of an innovative relationship, between SMOS V620 algorithm data and Ecosystem Functional Properties (EFPs) derived from flux towers data, was preliminary investigated for Africa and South America forests. Correlation was also explored for above ground forest biomass. High correlation values were found between SMOS vegetation optical depth (VOD) and a AGB reference map, and with EFPs at year level (2014). Different VOD-EFPs trends were found for different latitudinal belts, characterizing wet and dry forests. The results suggest that SMOS VOD data represent a tool able to provide repeated information on forest biomass and forest processes in tropical and subtropical ranges.
Paul Bodesheim:
Discovering unknown visual objects with novelty detection techniques.
2018. Dissertation. ISBN: 978-3-8439-3546-3
[bibtex] [web] [abstract]
Discovering unknown visual objects with novelty detection techniques.
2018. Dissertation. ISBN: 978-3-8439-3546-3
[bibtex] [web] [abstract]
Humans are able to recognize objects of different categories like apple, bottle, or car without difficulty just by looking at them. Even if an unknown object occurs, they rapidly decide that they have never seen something like this before. However, for machines these tasks turn out to be much more complicated. Although recently developed algorithms of computer vision and machine learning achieve remarkably good performances in automatically recognizing objects in images and videos, their abilities are restricted to a fixed amount of known object categories that have been defined by annotated example images in a learning phase beforehand. The detection of unknown objects belonging to other categories is usually neglected by relying on the closed world assumption, which indicates that only objects from already observed categories appear in the application. This rarely holds for the real world in uncontrolled environments that are subject to change and where possibly occurring categories can not be determined in advance. For this reason, new methods and algorithmic concepts are introduced in the present thesis, which allow for an automatic discovery of objects belonging to unknown and therefore novel categories.
Paul Bodesheim, Martin Jung, Fabian Gans, Miguel D. Mahecha, Markus Reichstein:
Upscaled diurnal cycles of land-atmosphere fluxes: a new global half-hourly data product.
Earth System Science Data. 10 (3) : pp. 1327-1365. 2018.
[bibtex] [pdf] [web] [doi] [abstract]
Upscaled diurnal cycles of land-atmosphere fluxes: a new global half-hourly data product.
Earth System Science Data. 10 (3) : pp. 1327-1365. 2018.
[bibtex] [pdf] [web] [doi] [abstract]
Interactions between the biosphere and the atmosphere can be well characterized by fluxes between the two. In particular, carbon and energy fluxes play a major role in understanding biogeochemical processes on an ecosystem level or global scale. However, the fluxes can only be measured at individual sites, e.g., by eddy covariance towers, and an upscaling of these local observations is required to analyze global patterns. Previous work focused on upscaling monthly, 8-day, or daily average values, and global maps for each flux have been provided accordingly. In this paper, we raise the upscaling of carbon and energy fluxes between land and atmosphere to the next level by increasing the temporal resolution to subdaily timescales. We provide continuous half-hourly fluxes for the period from 2001 to 2014 at 0.5° spatial resolution, which allows for analyzing diurnal cycles globally. The data set contains four fluxes: gross primary production (GPP), net ecosystem exchange (NEE), latent heat (LE), and sensible heat (H). We propose two prediction approaches for the diurnal cycles based on large-scale regression models and compare them in extensive cross-validation experiments using different sets of predictor variables. We analyze the results for a set of FLUXNET tower sites showing the suitability of our approaches for this upscaling task. Finally, we have selected one approach to calculate the global half-hourly data products based on predictor variables from remote sensing and meteorology at daily resolution as well as half-hourly potential radiation. In addition, we provide a derived product that only contains monthly average diurnal cycles, which is a lightweight version in terms of data storage that still allows studying the important characteristics of diurnal patterns globally. We recommend to primarily use these monthly average diurnal cycles, because they are less affected by the impacts of day-to-day variation, observation noise, and short-term fluctuations on subdaily timescales compared to the full half-hourly flux products. The global half-hourly data products are available at <a href= https://doi.org/10.17871/BACI.224>https://doi.org/10.17871/BACI.224</a>.
2017
Erik Rodner, Alexander Freytag, Paul Bodesheim, Björn Fröhlich, Joachim Denzler:
Large-Scale Gaussian Process Inference with Generalized Histogram Intersection Kernels for Visual Recognition Tasks.
International Journal of Computer Vision (IJCV). 121 (2) : pp. 253-280. 2017.
[bibtex] [pdf] [web] [doi] [abstract]
Large-Scale Gaussian Process Inference with Generalized Histogram Intersection Kernels for Visual Recognition Tasks.
International Journal of Computer Vision (IJCV). 121 (2) : pp. 253-280. 2017.
[bibtex] [pdf] [web] [doi] [abstract]
We present new methods for fast Gaussian process (GP) inference in large-scale scenarios including exact multi-class classification with label regression, hyperparameter optimization, and uncertainty prediction. In contrast to previous approaches, we use a full Gaussian process model without sparse approximation techniques. Our methods are based on exploiting generalized histogram intersection kernels and their fast kernel multiplications. We empirically validate the suitability of our techniques in a wide range of scenarios with tens of thousands of examples. Whereas plain GP models are intractable due to both memory consumption and computation time in these settings, our results show that exact inference can indeed be done efficiently. In consequence, we enable every important piece of the Gaussian process framework - learning, inference, hyperparameter optimization, variance estimation, and online learning - to be used in realistic scenarios with more than a handful of data.
Flurin Babst, Benjamin Poulter, Paul Bodesheim, Miguel D. Mahecha, David C. Frank:
Improved tree-ring archives will support earth-system science.
Nature Ecology \& Evolution. 1 (2) : 2017.
[bibtex] [pdf] [web] [doi] [abstract]
Improved tree-ring archives will support earth-system science.
Nature Ecology \& Evolution. 1 (2) : 2017.
[bibtex] [pdf] [web] [doi] [abstract]
A steep decline in archiving could make large tree-ring datasets irrelevant. But increased spatiotemporal coverage, the addition of novel parameters at sub-annual resolution, and integration with other in situ and remote Earth observations will elevate tree-ring data as an essential component of global-change research.
Flurin Babst, Olivier Bouriaud, Benjamin Poulter, Zhen Zhang, Valerie Trouet, Margaret Evans, Noah Charney, Sydne Record, Brian Enquist, Kristina Seftigen, Jesper Björklund, Stefan Klesse, Paul Bodesheim, Miguel Mahecha, Martin Girardin, Andrew Friend, David Frank:
When tree rings go global: challenges and opportunities for retro- andprospective insights.
European Geosciences Union General Assembly (EGU): Abstract + Oral Presentation. 2017.
[bibtex] [pdf] [web] [abstract]
When tree rings go global: challenges and opportunities for retro- andprospective insights.
European Geosciences Union General Assembly (EGU): Abstract + Oral Presentation. 2017.
[bibtex] [pdf] [web] [abstract]
The demand for extensive empirical data on forest growth and its climate sensitivity is growing rapidly with continued anthropogenic warming of the Earth. In principal, tree-ring records are the only resource that can provide such information along large environmental gradients and over sub-annual to centennial time scales. Yet, global tree-ring archives have remained an underrepresented resource in Earth system science. Some of the major challenges that complicate the use of existing tree-ring archives in environmental change research include: i) their limited spatial representativity for global forests, ii) varying sampling schemes that often preclude absolute estimates of forest growth, iii) different spatial and temporal resolution compared to remotely sensed and in-situ Earth observations, and iv) uncertainties arising when past climate-growth relationships are extrapolated into the future. Here we provide a perspective on possible solutions to these issues that emerged from recent and ongoing work. Regarding existing tree-ring networks, we show how spatial interpolation, statistical upscaling, and mechanistic modelling may improve their spatiotemporal coverage. An option to account for non-climatic (e.g. CO2) effects in projections of changing climate-growth relationships is also presented. Regarding future research avenues, we advocate for intensified data collection in warm regions, improved coordination with Earth observation networks, and refined concepts to integrate tree-ring data with computational estimates of forest productivity. Such efforts are expected to elevate tree-ring data as an essential component in Earth system science.
Milan Flach, Fabian Gans, Alexander Brenning, Joachim Denzler, Markus Reichstein, Erik Rodner, Sebastian Bathiany, Paul Bodesheim, Yanira Guanche, Sebasitan Sippel, Miguel D. Mahecha:
Multivariate anomaly detection for Earth observations: a comparison of algorithms and feature extraction techniques.
Earth System Dynamics. 8 (3) : pp. 677-696. 2017.
[bibtex] [pdf] [web] [doi] [abstract]
Multivariate anomaly detection for Earth observations: a comparison of algorithms and feature extraction techniques.
Earth System Dynamics. 8 (3) : pp. 677-696. 2017.
[bibtex] [pdf] [web] [doi] [abstract]
Today, many processes at the Earth's surface are constantly monitored by multiple data streams. These observations have become central to advancing our understanding of vegetation dynamics in response to climate or land use change. Another set of important applications is monitoring effects of extreme climatic events, other disturbances such as fires, or abrupt land transitions. One important methodological question is how to reliably detect anomalies in an automated and generic way within multivariate data streams, which typically vary seasonally and are interconnected across variables. Although many algorithms have been proposed for detecting anomalies in multivariate data, only a few have been investigated in the context of Earth system science applications. In this study, we systematically combine and compare feature extraction and anomaly detection algorithms for detecting anomalous events. Our aim is to identify suitable workflows for automatically detecting anomalous patterns in multivariate Earth system data streams. We rely on artificial data that mimic typical properties and anomalies in multivariate spatiotemporal Earth observations like sudden changes in basic characteristics of time series such as the sample mean, the variance, changes in the cycle amplitude, and trends. This artificial experiment is needed as there is no "gold standard" for the identification of anomalies in real Earth observations. Our results show that a well-chosen feature extraction step (e.g., subtracting seasonal cycles, or dimensionality reduction) is more important than the choice of a particular anomaly detection algorithm. Nevertheless, we identify three detection algorithms (k-nearest neighbors mean distance, kernel density estimation, a recurrence approach) and their combinations (ensembles) that outperform other multivariate approaches as well as univariate extreme-event detection methods. Our results therefore provide an effective workflow to automatically detect anomalies in Earth system science data.
Paul Bodesheim, Martin Jung, Miguel D. Mahecha, Markus Reichstein:
Upscaling diurnal cycles of carbon fluxes.
European Geosciences Union General Assembly (EGU): Abstract + Poster Presentation. 2017.
[bibtex] [pdf] [web] [abstract]
Upscaling diurnal cycles of carbon fluxes.
European Geosciences Union General Assembly (EGU): Abstract + Poster Presentation. 2017.
[bibtex] [pdf] [web] [abstract]
Carbon fluxes like Gross Primary Production (GPP) and Net Ecosystem Exchange (NEE) are important variables for studying interactions between the atmosphere and the biosphere in different ecosystems. They are typically derived from measurements at Eddy covariance towers and the FLUXNET global network consists of hundreds of such sites. In order to diagnose global GPP and NEE patterns from FLUXNET, upscaling approaches have been used in the past to extrapolate the site measurements to continental and global scale. However, respective products have a daily or monthly temporal resolution and do not allow for analyzing patterns related to diurnal variations of GPP and NEE. To raise these upscaling approaches to the next level, we present our first results on upscaling diurnal cycles of GPP and NEE with half-hourly resolution. We use random forest regression models to estimate the relationship between predictor variables and fluxes based on more than four million half-hourly observations from FLUXNET sites. We have developed and tested two approaches that overcome the mismatch in the temporal resolution between predictor variables at daily resolution and fluxes at half-hourly resolution. Based on thorough leave-one-site-out cross-validation we show that the approach works very well. Finally, we used the trained models for computing global products of half-hourly GPP and NEE that cover the years 2001 to 2014 and present global patterns of diurnal carbon flux variations derived from the upscaling approach.
2016
Erik Rodner, Björn Barz, Yanira Guanche, Milan Flach, Miguel Mahecha, Paul Bodesheim, Markus Reichstein, Joachim Denzler:
Maximally Divergent Intervals for Anomaly Detection.
Workshop on Anomaly Detection (ICML-WS). 2016. Best Paper Award
[bibtex] [pdf] [web] [code] [abstract]
Maximally Divergent Intervals for Anomaly Detection.
Workshop on Anomaly Detection (ICML-WS). 2016. Best Paper Award
[bibtex] [pdf] [web] [code] [abstract]
We present new methods for batch anomaly detection in multivariate time series. Our methods are based on maximizing the Kullback-Leibler divergence between the data distribution within and outside an interval of the time series. An empirical analysis shows the benefits of our algorithms compared to methods that treat each time step independently from each other without optimizing with respect to all possible intervals.
Markus Reichstein, Martin Jung, Paul Bodesheim, Miguel D. Mahecha, Fabian Gans, Erik Rodner, Gustau Camps-Valls, Dario Papale, Gianluca Tramontana, Joachim Denzler, Dennis D. Baldocchi:
Potential of new machine learning methods for understanding long-term interannual variability of carbon and energy fluxes and states from site to global scale.
American Geophysical Union Fall Meeting (AGU): Abstract + Oral Presentation. 2016.
[bibtex] [web] [abstract]
Potential of new machine learning methods for understanding long-term interannual variability of carbon and energy fluxes and states from site to global scale.
American Geophysical Union Fall Meeting (AGU): Abstract + Oral Presentation. 2016.
[bibtex] [web] [abstract]
Machine learning tools have been very successful in describing and predicting instantaneous climatic influences on the spatial and seasonal variability of biosphere-atmosphere exchange, while interannual variability is harder to model (e.g. Jung et al. 2011, JGR Biogeosciences). Here we hypothesize that innterannual variability is harder to describe for two reasons. 1) The signal-to-noise ratio in both, predictors (e.g. remote sensing) and target variables (e.g. net ecosystem exchange) is relatively weak, 2) The employed machine learning methods do not sufficiently account for dynamic lag and carry-over effects. In this presentation we can largely confirm both hypotheses: 1) We show that based on FLUXNET data and an ensemble of machine learning methods we can arrive at estimates of global NEE that correlate well with the residual land sink overall and CO2 flux inversions over latitudinal bands. Furthermore these results highlight the importance of variations in water availability for variations in carbon fluxes locally, while globally, as a scale-emergent property, tropical temperatures correlate well with the atmospheric CO2 growth rate, because of spatial anticorrelation and compensation of water availability. 2) We evidence with synthetic and real data that machine learning methods with embed dynamic memory effects of the system such as recurrent neural networks (RNNs) are able to better capture lag and carry-over effect which are caused by dynamic carbon pools in vegetation and soils. For these methods, long-term replicate observations are an essential asset.
Milan Flach, Miguel Mahecha, Fabian Gans, Erik Rodner, Paul Bodesheim, Yanira Guanche-Garcia, Alexander Brenning, Joachim Denzler, Markus Reichstein:
Using Statistical Process Control for detecting anomalies in multivariate spatiotemporal Earth Observations.
European Geosciences Union General Assembly (EGU): Abstract + Oral Presentation. 2016.
[bibtex] [pdf] [web] [abstract]
Using Statistical Process Control for detecting anomalies in multivariate spatiotemporal Earth Observations.
European Geosciences Union General Assembly (EGU): Abstract + Oral Presentation. 2016.
[bibtex] [pdf] [web] [abstract]
The number of available Earth observations (EOs) is currently substantially increasing. Detecting anomalous pat-terns in these multivariate time series is an important step in identifying changes in the underlying dynamicalsystem. Likewise, data quality issues might result in anomalous multivariate data constellations and have to beidentified before corrupting subsequent analyses. In industrial application a common strategy is to monitor pro-duction chains with several sensors coupled to some statistical process control (SPC) algorithm. The basic ideais to raise an alarm when these sensor data depict some anomalous pattern according to the SPC, i.e. the produc-tion chain is considered ’out of control’. In fact, the industrial applications are conceptually similar to the on-linemonitoring of EOs. However, algorithms used in the context of SPC or process monitoring are rarely consideredfor supervising multivariate spatio-temporal Earth observations. The objective of this study is to exploit the poten-tial and transferability of SPC concepts to Earth system applications. We compare a range of different algorithmstypically applied by SPC systems and evaluate their capability to detect e.g. known extreme events in land sur-face processes. Specifically two main issues are addressed: (1) identifying the most suitable combination of datapre-processing and detection algorithm for a specific type of event and (2) analyzing the limits of the individual ap-proaches with respect to the magnitude, spatio-temporal size of the event as well as the data’s signal to noise ratio.Extensive artificial data sets that represent the typical properties of Earth observations are used in this study. Ourresults show that the majority of the algorithms used can be considered for the detection of multivariate spatiotem-poral events and directly transferred to real Earth observation data as currently assembled in different projectsat the European scale, e.g. http://baci-h2020.eu/index.php/ and http://earthsystemdatacube.net/. Known anomaliessuch as the Russian heatwave are detected as well as anomalies which are not detectable with univariate methods.
Milan Flach, Sebastian Sippel, Paul Bodesheim, Alexander Brenning, Joachim Denzler, Fabian Gans, Yanira Guanche, Markus Reichstein, Erik Rodner, Miguel D. Mahecha:
Hot spots of multivariate extreme anomalies in Earth observations.
American Geophysical Union Fall Meeting (AGU): Abstract + Oral Presentation. 2016.
[bibtex] [web] [abstract]
Hot spots of multivariate extreme anomalies in Earth observations.
American Geophysical Union Fall Meeting (AGU): Abstract + Oral Presentation. 2016.
[bibtex] [web] [abstract]
Anomalies in Earth observations might indicate data quality issues, extremes or the change of underlying processes within a highly multivariate system. Thus, considering the multivariate constellation of variables for extreme detection yields crucial additional information over conventional univariate approaches. We highlight areas in which multivariate extreme anomalies are more likely to occur, i.e. hot spots of extremes in global atmospheric Earth observations that impact the Biosphere. In addition, we present the year of the most unusual multivariate extreme between 2001 and 2013 and show that these coincide with well known high impact extremes. Technically speaking, we account for multivariate extremes by using three sophisticated algorithms adapted from computer science applications. Namely an ensemble of the k-nearest neighbours mean distance, a kernel density estimation and an approach based on recurrences is used. However, the impact of atmosphere extremes on the Biosphere might largely depend on what is considered to be normal, i.e. the shape of the mean seasonal cycle and its inter-annual variability. We identify regions with similar mean seasonality by means of dimensionality reduction in order to estimate in each region both the `normal' variance and robust thresholds for detecting the extremes. In addition, we account for challenges like heteroscedasticity in Northern latitudes. Apart from hot spot areas, those anomalies in the atmosphere time series are of particular interest, which can only be detected by a multivariate approach but not by a simple univariate approach. Such an anomalous constellation of atmosphere variables is of interest if it impacts the Biosphere. The multivariate constellation of such an anomalous part of a time series is shown in one case study indicating that multivariate anomaly detection can provide novel insights into Earth observations.
Sebastian Sippel, Holger Lange, Miguel D. Mahecha, Michael Hauhs, Paul Bodesheim, Thomas Kaminski, Fabian Gans, Osvaldo A. Rosso:
Diagnosing the Dynamics of Observed and Simulated Ecosystem Gross Primary Productivity with Time Causal Information Theory Quantifiers.
PLOS ONE. 11 (10) : pp. 1-29. 2016.
[bibtex] [pdf] [web] [doi] [abstract]
Diagnosing the Dynamics of Observed and Simulated Ecosystem Gross Primary Productivity with Time Causal Information Theory Quantifiers.
PLOS ONE. 11 (10) : pp. 1-29. 2016.
[bibtex] [pdf] [web] [doi] [abstract]
Data analysis and model-data comparisons in the environmental sciences require diagnostic measures that quantify time series dynamics and structure, and are robust to noise in observational data. This paper investigates the temporal dynamics of environmental time series using measures quantifying their information content and complexity. The measures are used to classify natural processes on one hand, and to compare models with observations on the other. The present analysis focuses on the global carbon cycle as an area of research in which model-data integration and comparisons are key to improving our understanding of natural phenomena. We investigate the dynamics of observed and simulated time series of Gross Primary Productivity (GPP), a key variable in terrestrial ecosystems that quantifies ecosystem carbon uptake. However, the dynamics, patterns and magnitudes of GPP time series, both observed and simulated, vary substantially on different temporal and spatial scales. We demonstrate here that information content and complexity, or Information Theory Quantifiers (ITQ) for short, serve as robust and efficient data-analytical and model benchmarking tools for evaluating the temporal structure and dynamical properties of simulated or observed time series at various spatial scales. At continental scale, we compare GPP time series simulated with two models and an observations-based product. This analysis reveals qualitative differences between model evaluation based on ITQ compared to traditional model performance metrics, indicating that good model performance in terms of absolute or relative error does not imply that the dynamics of the observations is captured well. Furthermore, we show, using an ensemble of site-scale measurements obtained from the FLUXNET archive in the Mediterranean, that model-data or model-model mismatches as indicated by ITQ can be attributed to and interpreted as differences in the temporal structure of the respective ecological time series. At global scale, our understanding of C fluxes relies on the use of consistently applied land models. Here, we use ITQ to evaluate model structure: The measures are largely insensitive to climatic scenarios, land use and atmospheric gas concentrations used to drive them, but clearly separate the structure of 13 different land models taken from the CMIP5 archive and an observations-based product. In conclusion, diagnostic measures of this kind provide data-analytical tools that distinguish different types of natural processes based solely on their dynamics, and are thus highly suitable for environmental science applications such as model structural diagnostics.
2015
Christoph Käding, Alexander Freytag, Erik Rodner, Paul Bodesheim, Joachim Denzler:
Active Learning and Discovery of Object Categories in the Presence of Unnameable Instances.
IEEE Conference on Computer Vision and Pattern Recognition (CVPR). Pages 4343-4352. 2015.
[bibtex] [pdf] [web] [doi] [code] [presentation] [supplementary] [abstract]
Active Learning and Discovery of Object Categories in the Presence of Unnameable Instances.
IEEE Conference on Computer Vision and Pattern Recognition (CVPR). Pages 4343-4352. 2015.
[bibtex] [pdf] [web] [doi] [code] [presentation] [supplementary] [abstract]
Current visual recognition algorithms are "hungry" for data but massive annotation is extremely costly. Therefore, active learning algorithms are required that reduce labeling efforts to a minimum by selecting examples that are most valuable for labeling. In active learning, all categories occurring in collected data are usually assumed to be known in advance and experts should be able to label every requested instance. But do these assumptions really hold in practice? Could you name all categories in every image? Existing algorithms completely ignore the fact that there are certain examples where an oracle can not provide an answer or which even do not belong to the current problem domain. Ideally, active learning techniques should be able to discover new classes and at the same time cope with queries an expert is not able or willing to label. To meet these observations, we present a variant of the expected model output change principle for active learning and discovery in the presence of unnameable instances. Our experiments show that in these realistic scenarios, our approach substantially outperforms previous active learning methods, which are often not even able to improve with respect to the baseline of random query selection.
Gustau Camps-Valls, Martin Jung, Kazuhito Ichii, Dario Papale, Gianluca Tramontana, Paul Bodesheim, Christopher Schwalm, Jakob Zscheischler, Miguel D. Mahecha, Markus Reichstein:
Ranking drivers of global carbon and energy fluxes over land.
IEEE International Geoscience and Remote Sensing Symposium (IGARSS). Pages 4416-4419. 2015.
[bibtex] [pdf] [web] [doi] [abstract]
Ranking drivers of global carbon and energy fluxes over land.
IEEE International Geoscience and Remote Sensing Symposium (IGARSS). Pages 4416-4419. 2015.
[bibtex] [pdf] [web] [doi] [abstract]
The accurate estimation of carbon and heat fluxes at global scale is paramount for future policy decisions in the context of global climate change. This paper analyzes the relative relevance of potential remote sensing and meteorological drivers of global carbon and energy fluxes over land. The study is done in an indirect way via upscaling both Gross Primary Production (GPP) and latent energy (LE) using Gaussian Process regression (GPR). In summary, GPR is successfully compared to multivariate linear regression (RMSE gain of +4.17% in GPP and +7.63% in LE) and kernel ridge regression (+2.91% in GPP and +3.07% in LE). The best GP models are then studied in terms of explanatory power based on the analysis of the lengthscales of the anisotropic covariance function, sensitivity maps of the predictive mean, and the robustness to distortions in the input variables. It is concluded that GPP is predominantly mediated by several vegetation indices and land surface temperature (LST), while LE is mostly driven by LST, global radiation and vegetation indices.
Paul Bodesheim, Alexander Freytag, Erik Rodner, Joachim Denzler:
Local Novelty Detection in Multi-class Recognition Problems.
IEEE Winter Conference on Applications of Computer Vision (WACV). Pages 813-820. 2015.
[bibtex] [pdf] [web] [doi] [supplementary] [abstract]
Local Novelty Detection in Multi-class Recognition Problems.
IEEE Winter Conference on Applications of Computer Vision (WACV). Pages 813-820. 2015.
[bibtex] [pdf] [web] [doi] [supplementary] [abstract]
In this paper, we propose using local learning for multiclass novelty detection, a framework that we call local novelty detection. Estimating the novelty of a new sample is an extremely challenging task due to the large variability of known object categories. The features used to judge on the novelty are often very specific for the object in the image and therefore we argue that individual novelty models for each test sample are important. Similar to human experts, it seems intuitive to first look for the most related images thus filtering out unrelated data. Afterwards, the system focuses on discovering similarities and differences to those images only. Therefore, we claim that it is beneficial to solely consider training images most similar to a test sample when deciding about its novelty. Following the principle of local learning, for each test sample a local novelty detection model is learned and evaluated. Our local novelty score turns out to be a valuable indicator for deciding whether the sample belongs to a known category from the training set or to a new, unseen one. With our local novelty detection approach, we achieve state-of-the-art performance in multi-class novelty detection on two popular visual object recognition datasets, Caltech-256 and Image Net. We further show that our framework: (i) can be successfully applied to unknown face detection using the Labeled-Faces-in-the-Wild dataset and (ii) outperforms recent work on attribute-based unfamiliar class detection in fine-grained recognition of bird species on the challenging CUB-200-2011 dataset.
2014
Alexander Freytag, Johannes Rühle, Paul Bodesheim, Erik Rodner, Joachim Denzler:
Seeing through bag-of-visual-word glasses: towards understanding quantization effects in feature extraction methods.
ICPR Workshop on Features and Structures (FEAST): Extended Abstract + Poster Presentation. 2014. Best Poster Award
[bibtex] [pdf] [code] [presentation]
Seeing through bag-of-visual-word glasses: towards understanding quantization effects in feature extraction methods.
ICPR Workshop on Features and Structures (FEAST): Extended Abstract + Poster Presentation. 2014. Best Poster Award
[bibtex] [pdf] [code] [presentation]
Alexander Freytag, Johannes Rühle, Paul Bodesheim, Erik Rodner, Joachim Denzler:
Seeing through bag-of-visual-word glasses: towards understanding quantization effects in feature extraction methods.
2014. Technical Report TR-FSU-INF-CV-2014-01
[bibtex] [pdf] [code] [abstract]
Seeing through bag-of-visual-word glasses: towards understanding quantization effects in feature extraction methods.
2014. Technical Report TR-FSU-INF-CV-2014-01
[bibtex] [pdf] [code] [abstract]
The bag-of-visual-word (BoW) model is one of the most common concepts for image categorization and feature extraction. Although our community developed powerful BoW approaches for visual recognition and it serves as a great ad-hoc solution, unfortunately, there are several drawbacks that most researchers might be not aware of. In this paper, we aim at seeing behind the curtains and point to some of the negative aspects of these approaches which go usually unnoticed: (i) although BoW approaches are often motivated by relating clusters to meaningful object parts, this relation does not hold in practice with low-dimensional features such as HOG, and standard clustering method, (ii) clusters can be chosen randomly without loss in performance, (iii) BoW is often only collecting background statistics, and (iv) cluster assignments are not robust to small spatial shifts. Furthermore, we show the effect of BoW quantization and the related loss of visual information by a simple inversion method called HoggleBoW.
Mahesh Venkata Krishna, Paul Bodesheim, Marco Körner, Joachim Denzler:
Temporal Video Segmentation by Event Detection: A Novelty Detection Approach.
Pattern Recognition and Image Analysis. Advances in Mathematical Theory and Applications (PRIA). 24 (2) : pp. 243-255. 2014.
[bibtex] [pdf] [web] [doi] [abstract]
Temporal Video Segmentation by Event Detection: A Novelty Detection Approach.
Pattern Recognition and Image Analysis. Advances in Mathematical Theory and Applications (PRIA). 24 (2) : pp. 243-255. 2014.
[bibtex] [pdf] [web] [doi] [abstract]
Temporal segmentation of videos into meaningful image sequences containing some particular activities is an interesting problem in computer vision. We present a novel algorithm to achieve this semantic video segmentation. The segmentation task is accomplished through event detection in a frame-by-frame processing setup. We propose using one-class classification (OCC) techniques to detect events that indicate a new segment, since they have been proved to be successful in object classification and they allow for unsupervised event detection in a natural way. Various OCC schemes have been tested and compared, and additionally, an approach based on the temporal self-similarity maps (TSSMs) is also presented. The testing was done on a challenging publicly available thermal video dataset. The results are promising and show the suitability of our approaches for the task of temporal video segmentation.
2013
Alexander Freytag, Erik Rodner, Paul Bodesheim, Joachim Denzler:
Labeling examples that matter: Relevance-Based Active Learning with Gaussian Processes.
DAGM German Conference on Pattern Recognition (DAGM-GCPR). Pages 282-291. 2013.
[bibtex] [pdf] [web] [doi] [code] [supplementary] [abstract]
Labeling examples that matter: Relevance-Based Active Learning with Gaussian Processes.
DAGM German Conference on Pattern Recognition (DAGM-GCPR). Pages 282-291. 2013.
[bibtex] [pdf] [web] [doi] [code] [supplementary] [abstract]
Active learning is an essential tool to reduce manual annotation costs in the presence of large amounts of unsupervised data. In this paper, we introduce new active learning methods based on measuring the impact of a new example on the current model. This is done by deriving model changes of Gaussian process models in closed form. Furthermore, we study typical pitfalls in active learning and show that our methods automatically balance between the exploitation and the exploration trade-off. Experiments are performed with established benchmark datasets for visual object recognition and show that our new active learning techniques are able to outperform state-of-the-art methods.
Joachim Denzler, Erik Rodner, Paul Bodesheim, Alexander Freytag:
Beyond the closed-world assumption: The importance of novelty detection and open set recognition.
GCPR/DAGM Workshop on Unsolved Problems in Pattern Recognition and Computer Vision (GCPR-WS): Extended Abstract + Oral Presentation. 2013.
[bibtex] [web]
Beyond the closed-world assumption: The importance of novelty detection and open set recognition.
GCPR/DAGM Workshop on Unsolved Problems in Pattern Recognition and Computer Vision (GCPR-WS): Extended Abstract + Oral Presentation. 2013.
[bibtex] [web]
Mahesh Venkata Krishna, Paul Bodesheim, Joachim Denzler:
Video Segmentation by Event Detection: A Novel One-class Classification Approach.
4th International Workshop on Image Mining. Theory and Applications (IMTA-4). 2013.
[bibtex] [pdf] [abstract]
Video Segmentation by Event Detection: A Novel One-class Classification Approach.
4th International Workshop on Image Mining. Theory and Applications (IMTA-4). 2013.
[bibtex] [pdf] [abstract]
Segmenting videos into meaningful image sequences of some particular activities is an interesting problem in computer vision. In this paper, a novelalgorithm is presented to achieve this semantic video segmentation. The goal is to make the system work unsupervised and generic in terms of application scenar-ios. The segmentation task is accomplished through event detection in a frame-by-frame processing setup. For event detection, we use a one-class classificationapproach based on Gaussian processes, which has been proved to be successful in object classification. The algorithm is tested on videos from a publicly avail-able change detection database and the results clearly show the suitability of our approach for the task of video segmentation.
Paul Bodesheim, Alexander Freytag, Erik Rodner, Joachim Denzler:
Approximations of Gaussian Process Uncertainties for Visual Recognition Problems.
Scandinavian Conference on Image Analysis (SCIA). Pages 182-194. 2013.
[bibtex] [pdf] [web] [doi] [abstract]
Approximations of Gaussian Process Uncertainties for Visual Recognition Problems.
Scandinavian Conference on Image Analysis (SCIA). Pages 182-194. 2013.
[bibtex] [pdf] [web] [doi] [abstract]
Gaussian processes offer the advantage of calculating the classification uncertainty in terms of predictive variance associated with the classification result. This is especially useful to select informative samples in active learning and to spot samples of previously unseen classes known as novelty detection. However, the Gaussian process framework suffers from high computational complexity leading to computation times too large for practical applications. Hence, we propose an approximation of the Gaussian process predictive variance leading to rigorous speedups. The complexity of both learning and testing the classification model regarding computational time and memory demand decreases by one order with respect to the number of training samples involved. The benefits of our approximations are verified in experimental evaluations for novelty detection and active learning of visual object categories on the datasets C-Pascal of Pascal VOC 2008, Caltech-256, and ImageNet.
Paul Bodesheim, Alexander Freytag, Erik Rodner, Michael Kemmler, Joachim Denzler:
Kernel Null Space Methods for Novelty Detection.
IEEE Conference on Computer Vision and Pattern Recognition (CVPR). Pages 3374-3381. 2013.
[bibtex] [pdf] [web] [doi] [code] [presentation] [abstract]
Kernel Null Space Methods for Novelty Detection.
IEEE Conference on Computer Vision and Pattern Recognition (CVPR). Pages 3374-3381. 2013.
[bibtex] [pdf] [web] [doi] [code] [presentation] [abstract]
Detecting samples from previously unknown classes is a crucial task in object recognition, especially when dealing with real-world applications where the closed-world assumption does not hold. We present how to apply a null space method for novelty detection, which maps all training samples of one class to a single point. Beside the possibility of modeling a single class, we are able to treat multiple known classes jointly and to detect novelties for a set of classes with a single model. In contrast to modeling the support of each known class individually, our approach makes use of a projection in a joint subspace where training samples of all known classes have zero intra-class variance. This subspace is called the null space of the training data. To decide about novelty of a test sample, our null space approach allows for solely relying on a distance measure instead of performing density estimation directly. Therefore, we derive a simple yet powerful method for multi-class novelty detection, an important problem not studied sufficiently so far. Our novelty detection approach is assessed in comprehensive multi-class experiments using the publicly available datasets Caltech-256 and ImageNet. The analysis reveals that our null space approach is perfectly suited for multi-class novelty detection since it outperforms all other methods.
2012
Alexander Freytag, Erik Rodner, Paul Bodesheim, Joachim Denzler:
Beyond Classification - Large-scale Gaussian Process Inference and Uncertainty Prediction.
Big Data Meets Computer Vision: First International Workshop on Large Scale Visual Recognition and Retrieval (NIPS-WS). 2012. This workshop article is a short version of our ACCV 2012 paper.
[bibtex] [pdf] [abstract]
Beyond Classification - Large-scale Gaussian Process Inference and Uncertainty Prediction.
Big Data Meets Computer Vision: First International Workshop on Large Scale Visual Recognition and Retrieval (NIPS-WS). 2012. This workshop article is a short version of our ACCV 2012 paper.
[bibtex] [pdf] [abstract]
Due to the massive (labeled) data available on the web, a tremendous interest in large-scale machine learning methods has emerged in the last years. Whereas, most of the work done in this new area of research focused on fast and efficient classification algorithms, we show in this paper how other aspects of learning can also be covered using massive datasets. The paper briefly presents techniques allowing for utilizing the full posterior obtained from Gaussian process regression (predictive mean and variance) with tens of thousands of data points and without relying on sparse approximation approaches. Experiments are done for active learning and one-class classification showing the benefits in large-scale settings.
Alexander Freytag, Erik Rodner, Paul Bodesheim, Joachim Denzler:
Rapid Uncertainty Computation with Gaussian Processes and Histogram Intersection Kernels.
Asian Conference on Computer Vision (ACCV). Pages 511-524. 2012. Best Paper Honorable Mention Award
[bibtex] [pdf] [web] [doi] [presentation] [abstract]
Rapid Uncertainty Computation with Gaussian Processes and Histogram Intersection Kernels.
Asian Conference on Computer Vision (ACCV). Pages 511-524. 2012. Best Paper Honorable Mention Award
[bibtex] [pdf] [web] [doi] [presentation] [abstract]
An important advantage of Gaussian processes is the ability to directly estimate classification uncertainties in a Bayesian manner. In this paper, we develop techniques that allow for estimating these uncertainties with a runtime linear or even constant with respect to the number of training examples. Our approach makes use of all training data without any sparse approximation technique while needing only a linear amount of memory. To incorporate new information over time, we further derive online learning methods leading to significant speed-ups and allowing for hyperparameter optimization on-the-fly. We conduct several experiments on public image datasets for the tasks of one-class classification and active learning, where computing the uncertainty is an essential task. The experimental results highlight that we are able to compute classification uncertainties within microseconds even for large-scale datasets with tens of thousands of training examples.
Erik Rodner, Alexander Freytag, Paul Bodesheim, Joachim Denzler:
Large-Scale Gaussian Process Classification with Flexible Adaptive Histogram Kernels.
European Conference on Computer Vision (ECCV). Pages 85-98. 2012.
[bibtex] [pdf] [web] [doi] [supplementary] [abstract]
Large-Scale Gaussian Process Classification with Flexible Adaptive Histogram Kernels.
European Conference on Computer Vision (ECCV). Pages 85-98. 2012.
[bibtex] [pdf] [web] [doi] [supplementary] [abstract]
We present how to perform exact large-scale multi-class Gaussian process classification with parameterized histogram intersection kernels. In contrast to previous approaches, we use a full Bayesian model without any sparse approximation techniques, which allows for learning in sub-quadratic and classification in constant time. To handle the additional model flexibility induced by parameterized kernels, our approach is able to optimize the parameters with large-scale training data. A key ingredient of this optimization is a new efficient upper bound of the negative Gaussian process log-likelihood. Experiments with image categorization tasks exhibit high performance gains with flexible kernels as well as learning within a few minutes and classification in microseconds for databases, where exact Gaussian process inference was not possible before.
Paul Bodesheim, Erik Rodner, Alexander Freytag, Joachim Denzler:
Divergence-Based One-Class Classification Using Gaussian Processes.
British Machine Vision Conference (BMVC). Pages 50.1-50.11. 2012.
[bibtex] [pdf] [web] [doi] [presentation] [abstract]
Divergence-Based One-Class Classification Using Gaussian Processes.
British Machine Vision Conference (BMVC). Pages 50.1-50.11. 2012.
[bibtex] [pdf] [web] [doi] [presentation] [abstract]
We present an information theoretic framework for one-class classification, which allows for deriving several new novelty scores. With these scores, we are able to rank samples according to their novelty and to detect outliers not belonging to a learnt data distribution. The key idea of our approach is to measure the impact of a test sample on the previously learnt model. This is carried out in a probabilistic manner using Jensen-Shannon divergence and reclassification results derived from the Gaussian process regression framework. Our method is evaluated using well-known machine learning datasets as well as large-scale image categorisation experiments showing its ability to achieve state-of-the-art performance.
2011
Paul Bodesheim:
Spectral Clustering of ROIs for Object Discovery.
Symposium of the German Association for Pattern Recognition (DAGM). Pages 450-455. 2011.
[bibtex] [pdf] [web] [doi] [abstract]
Spectral Clustering of ROIs for Object Discovery.
Symposium of the German Association for Pattern Recognition (DAGM). Pages 450-455. 2011.
[bibtex] [pdf] [web] [doi] [abstract]
Object discovery is one of the most important applications of unsupervised learning. This paper addresses several spectral clustering techniques to attain a categorization of objects in images without additional information such as class labels or scene descriptions. Due to the fact that background textures bias the performance of image categorization methods, a generic object detector based on some general requirements on objects is applied. The object detector provides rectangular regions of interest (ROIs) as object hypotheses independent of the underlying object class. Feature extraction is simply constrained to these bounding boxes to decrease the in uence of background clutter. Another aspect of this work is the utilization of a Gaussian mixture model (GMM) instead of k-means as usually used after feature transformation in spectral clustering. Several experiments have been done and the combination of spectral clustering techniques with the object detector is compared to the standard approach of computing features of the whole image.