Dr. Aishwarya Venkataramanan
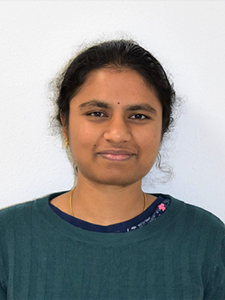
Address: | Computer Vision Group |
Department of Mathematics and Computer Science | |
Friedrich Schiller University of Jena | |
Ernst-Abbe-Platz 2 | |
07743 Jena | |
Room: | 1223 |
Phone: | +49 (0) 3641 9 46426 |
E-mail: | aishwarya (dot) venkataramanan (at) uni-jena (dot) de |
Links: |
Curriculum Vitae
since Jun 2024 | Research Associate / Postdoc |
Computer Vision Group, Friedrich Schiller University Jena | |
2020 – 2023 | PhD Student |
University of Lorraine and IRL GT-CNRS, France | |
PhD Thesis: “Automatic Identification of Diatoms using Deep Learning to Improve | |
Ecological Diagnosis of Aquatic Environments” | |
2020 | Research Engineer |
DREAM Lab, Georgia Tech-CNRS IRL2958 | |
2018 – 2020 | M. Sc. Studies in Electrical and Computer Engineering |
Georgia Institute of Technology, Metz, France | |
Master Thesis: “Generation of Realistic Tree Barks using Deep Learning” | |
2014 – 2018 | B. Eng. Studies in Electrical and Electronics |
Sri Sivasubramaniya Nadar College of Engineering (Anna University) |
Research Interests
- Computer Vision
- Deep Learning
- Uncertainty Quantification
Publications
2023
Aishwarya Venkataramanan, Assia Benbihi, Martin Laviale, Cédric Pradalier:
Gaussian Latent Representations for Uncertainty Estimation using Mahalanobis Distance in Deep Classifiers.
ICCV Workshop on Workshop on Uncertainty Quantification for Computer Vision (ICCV-WS). 2023.
[bibtex] [web] [abstract]
Gaussian Latent Representations for Uncertainty Estimation using Mahalanobis Distance in Deep Classifiers.
ICCV Workshop on Workshop on Uncertainty Quantification for Computer Vision (ICCV-WS). 2023.
[bibtex] [web] [abstract]
Recent works show that the data distribution in a network's latent space is useful for estimating classification uncertainty and detecting Out-of-distribution (OOD) samples. To obtain a well-regularized latent space that is conducive for uncertainty estimation, existing methods bring in significant changes to model architectures and training procedures. In this paper, we present a lightweight, fast, and high-performance regularization method for Mahalanobis distance-based uncertainty prediction, and that requires minimal changes to the network's architecture. To derive Gaussian latent representation favourable for Mahalanobis Distance calculation, we introduce a self-supervised representation learning method that separates in-class representations into multiple Gaussians. Classes with non-Gaussian representations are automatically identified and dynamically clustered into multiple new classes that are approximately Gaussian. Evaluation on standard OOD benchmarks shows that our method achieves state-of-the-art results on OOD detection with minimal inference time, and is very competitive on predictive probability calibration. Finally, we show the applicability of our method to a real-life computer vision use case on microorganism classification.
Aishwarya Venkataramanan, Martin Laviale, Cédric Pradalier:
Integrating Visual and Semantic Similarity Using Hierarchies for Image Retrieval.
International Conference on Computer Vision Systems (ICVS). Pages 422-431. 2023.
[bibtex] [web] [doi] [abstract]
Integrating Visual and Semantic Similarity Using Hierarchies for Image Retrieval.
International Conference on Computer Vision Systems (ICVS). Pages 422-431. 2023.
[bibtex] [web] [doi] [abstract]
Most of the research in content-based image retrieval (CBIR) focus on developing robust feature representations that can effectively retrieve instances from a database of images that are visually similar to a query. However, the retrieved images sometimes contain results that are not semantically related to the query. To address this, we propose a method for CBIR that captures both visual and semantic similarity using a visual hierarchy. The hierarchy is constructed by merging classes with overlapping features in the latent space of a deep neural network trained for classification, assuming that overlapping classes share high visual and semantic similarities. Finally, the constructed hierarchy is integrated into the distance calculation metric for similarity search. Experiments on standard datasets: CUB-200-2011 and CIFAR100, and a real-life use case using diatom microscopy images show that our method achieves superior performance compared to the existing methods on image retrieval.
Aishwarya Venkataramanan, Pierre Faure-Giovagnoli, Cyril Regan, David Heudre, Cécile Figus, Philippe Usseglio-Polatera, Cédric Pradalier, Martin Laviale:
Usefulness of Synthetic Datasets for Diatom Automatic Detection using a Deep-learning Approach.
Engineering Applications of Artificial Intelligence. 117 : pp. 105594. 2023.
[bibtex] [web] [doi] [abstract]
Usefulness of Synthetic Datasets for Diatom Automatic Detection using a Deep-learning Approach.
Engineering Applications of Artificial Intelligence. 117 : pp. 105594. 2023.
[bibtex] [web] [doi] [abstract]
Benthic diatoms are unicellular microalgae that are routinely used as bioindicators for monitoring the ecological status of freshwater. Their identification using light microscopy is a time-consuming and labor-intensive task that could be automated using deep-learning. However, training such networks relies on the availability of labeled datasets, which are difficult to obtain for these organisms. Herein, we propose a method to generate synthetic microscopy images for training. We gathered individual objects, i.e. 9230 diatoms from publicly available taxonomic guides and 600 items of debris from available real images. We collated a comprehensive dataset of synthetic microscopy images including both diatoms and debris using seamless blending and a combination of parameters such as image scaling, rotation, overlap and diatom-debris ratio. We then performed sensitivity analysis of the impact of the synthetic data parameters for training state-of-the art networks for horizontal and rotated bounding box detection (YOLOv5). We first trained the networks using the synthetic dataset and fine-tuned it to several real image datasets. Using this approach, the performance of the detection network was improved by up to 25% for precision and 23% for recall at an Intersection-over-Union(IoU) threshold of 0.5. This method will be extended in the future for training segmentation and classification networks.
2022
Aishwarya Venkataramanan, Antoine Richard, Cédric Pradalier:
A Data Driven Approach to Generate Realistic 3D Tree Barks.
Graphical Models. 123 : pp. 101166. 2022.
[bibtex] [web] [doi] [abstract]
A Data Driven Approach to Generate Realistic 3D Tree Barks.
Graphical Models. 123 : pp. 101166. 2022.
[bibtex] [web] [doi] [abstract]
3D models of trees are ubiquitous in video games, movies, and simulators. It is of paramount importance to generate high quality 3D models to enhance the visual content, and increase the diversity of the available models. In this work, we propose a methodology to create realistic 3D models of tree barks from a consumer-grade hand-held camera. Additionally, we present a pipeline that makes use of multi-view 3D Reconstruction and Generative Adversarial Networks (GANs) to generate the 3D models of the barks. We introduce a GAN referred to as the Depth-Reinforced-SPADE to generate the surfaces of the tree barks and the bark color concurrently. This GAN gives extensive control on what is being generated on the bark: moss, lichen, scars, etc. Finally, by testing our pipeline on different Northern-European trees whose barks exhibit radically different color patterns and surfaces, we show that our pipeline can be used to generate a broad panel of tree species’ bark.
2021
Aishwarya Venkataramanan, Martin Laviale, Cécile Figus, Philippe Usseglio-Polatera, Cédric Pradalier:
Tackling Inter-Class Similarity and Intra-Class Variance for Microscopic Image-based Classification.
International Conference on Computer Vision Systems (ICVS). Pages 93-103. 2021.
[bibtex] [web] [doi] [abstract]
Tackling Inter-Class Similarity and Intra-Class Variance for Microscopic Image-based Classification.
International Conference on Computer Vision Systems (ICVS). Pages 93-103. 2021.
[bibtex] [web] [doi] [abstract]
Automatic classification of aquatic microorganisms is based on the morphological features extracted from individual images. The current works on their classification do not consider the inter-class similarity and intra-class variance that causes misclassification. We are particularly interested in the case where variance within a class occurs due to discrete visual changes in microscopic images. In this paper, we propose to account for it by partitioning the classes with high variance based on the visual features. Our algorithm automatically decides the optimal number of sub-classes to be created and consider each of them as a separate class for training. This way, the network learns finer-grained visual features. Our experiments on two databases of freshwater benthic diatoms and marine plankton show that our method can outperform the state-of-the-art approaches for classification of these aquatic microorganisms.