Dr.-Ing. habil. Maha Shadaydeh
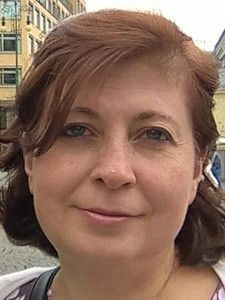
Address: | Computer Vision Group |
Department of Mathematics and Computer Science | |
Friedrich Schiller University of Jena | |
Ernst-Abbe-Platz 2 | |
07743 Jena | |
Germany | |
Phone: | +49 (0) 3641 9 46428 |
E-mail: | maha (dot) shadaydeh (at) uni-jena (dot) de |
Room: | 1221 |
Links: | GoogleScholar |
Curriculum Vitae
2019 – 2022 | Team Leader: “Event Detection and Causal Reasoning” |
Computer Vision Group, Friedrich Schiller University Jena | |
since 2017 | Scientific Associate |
Computer Vision Group, Friedrich Schiller University Jena | |
2012 – 2017 | Research Fellow |
Machine Perception Research Laboratory, Institute for Computer Science and Control, | |
Hungarian Academy of Sciences | |
2008 – 2012 | Associate Professor |
Electronic Engineering Department, Damascus University | |
Theses | |
Ph.D. | “Analysis of Dynamical Behavior of 2-D Adaptive Digital Filters” |
Electrical Communications Engineering, Tohoku University, Japan | |
M. Sc. | “Identification of Non-Minimum Phase FIR Systems using Higher-Order Statistics and Genetic Algorithm” |
Information and Computer Sciences, Toyohashi University of Technology, Japan |
Projects
Ongoing
- A Virtual “Werkstatt” for the Digitization in the Sciences, Zeiss Foundation, 2019-2024
- Understanding and modeling of the Earth system with machine learning (USMILE), European Research Council (ERC) Synergy Grant, 2019-2025
- Detection and attribution of changes in soil moisture and temperature as affected by plant diversity and climate, iDiv Flexpool Project, 2021-2024
- Deep state space models for understanding changes in ecosystems, DFG individual research grant SH 1682/1-1, 2021-2024
Former
Publications
2024
Gideon Stein, Jonas Ziemer, Carolin Wicker, Jannik Jaenichen, Gabriele Demisch, Daniel Kloepper, Katja Last, Joachim Denzler, Christiane Schmullius, Maha Shadaydeh, Clémence Dubois:
Data-driven Prediction of Large Infrastructure Movements Through Persistent Scatterer Time Series Modeling.
IEEE International Geoscience and Remote Sensing Symposium (IGARSS). 2024. (accepted)
[bibtex] [pdf]
Data-driven Prediction of Large Infrastructure Movements Through Persistent Scatterer Time Series Modeling.
IEEE International Geoscience and Remote Sensing Symposium (IGARSS). 2024. (accepted)
[bibtex] [pdf]
Gideon Stein, Maha Shadaydeh, Joachim Denzler:
Embracing the Black Box: Heading Towards Foundation Models for Causal Discovery from Time Series Data.
AAAI Workshop on AI for Time-series (AAAI-WS). 2024.
[bibtex] [pdf] [web] [abstract]
Embracing the Black Box: Heading Towards Foundation Models for Causal Discovery from Time Series Data.
AAAI Workshop on AI for Time-series (AAAI-WS). 2024.
[bibtex] [pdf] [web] [abstract]
Causal discovery from time series data encompasses many existing solutions, including those based on deep learning techniques. However, these methods typically do not endorse one of the most prevalent paradigms in deep learning: End-to-end learning. To address this gap, we explore what we call Causal Pretraining. A methodology that aims to learn a direct mapping from multivariate time series to the underlying causal graphs in a supervised manner. Our empirical findings suggest that causal discovery in a supervised manner is possible, assuming that the training and test time series samples share most of their dynamics. More importantly, we found evidence that the performance of Causal Pretraining can increase with data and model size, even if the additional data do not share the same dynamics. Further, we provide examples where causal discovery for real-world data with causally pretrained neural networks is possible within limits. We argue that this hints at the possibility of a foundation model for causal discovery.
Gideon Stein, Sai Karthikeya Vemuri, Yuanyuan Huang, Anne Ebeling, Nico Eisenhauer, Maha Shadaydeh, Joachim Denzler:
Investigating the Effects of Plant Diversity on Soil Thermal Diffusivity Using Physics- Informed Neural Networks.
ICLR Workshop on AI4DifferentialEquations In Science (ICLR-WS). 2024.
[bibtex] [pdf] [web]
Investigating the Effects of Plant Diversity on Soil Thermal Diffusivity Using Physics- Informed Neural Networks.
ICLR Workshop on AI4DifferentialEquations In Science (ICLR-WS). 2024.
[bibtex] [pdf] [web]
Maha Shadaydeh, Joachim Denzler, Mirco Migliavacca:
Physics Informed Modeling of Ecosystem Respiration via Dynamic Mode Decomposition with Control Input.
ICLR Workshop on Machine Learning for Remote Sensing (ICLR-WS). 2024.
[bibtex] [web] [abstract]
Physics Informed Modeling of Ecosystem Respiration via Dynamic Mode Decomposition with Control Input.
ICLR Workshop on Machine Learning for Remote Sensing (ICLR-WS). 2024.
[bibtex] [web] [abstract]
Ecosystem respiration (Reco) represents a significant component of the global carbon cycle, and accurate characterization of its dynamics is essential for a comprehensive understanding of ecosystem-climate interactions and the impacts of climate extremes on the ecosystem. In this paper, we present a novel data-driven and physics-aware method for estimating Reco dynamics using the dynamic mode decomposition with control input (DMDc), an emerging tool for analyzing nonlinear dynamical systems. The proposed model represents Reco as a state space model with an autonomous component and an exogenous control input. The control input can be any ecosystem driver(s), such as air temperature, soil temperature, or soil water content. This unique modeling approach allows controlled intervention to study the effects of different inputs on the system. We further discuss using time delay embedding of both components to characterize Reco dynamics. Experimental results using Fluxnet2015 data show that the prediction accuracy of Reco dynamics achieved with DMDc is comparable to state-of-the-art methods, making it a promising tool for analyzing the dynamic behavior of different vegetation ecosystems on multi-temporal scales in response to different climatic drivers.
Nico Eisenhauer, Karin Frank, Alexandra Weigelt, Bartosz Bartkowski, Rémy Beugnon, Katja Liebal, Miguel Mahecha, Martin Quaas, Djamil Al-Halbouni, Ana Bastos, Friedrich J. Bohn, Mariana Madruga de Brito, Joachim Denzler, Hannes Feilhauer, Rico Fischer, Immo Fritsche, Claudia Guimaraes-Steinicke, Martin Hänsel, Daniel B. M. Haun, Hartmut Herrmann, Andreas Huth, Heike Kalesse-Los, Michael Koetter, Nina Kolleck, Melanie Krause, Marlene Kretschmer, Pedro J. Leitão, Torsten Masson, Karin Mora, Birgit Müller, Jian Peng, Mira L. Pöhlker, Leonie Ratzke, Markus Reichstein, Solveig Richter, Nadja Rüger, Beatriz Sánchez-Parra, Maha Shadaydeh, Sebastian Sippel, Ina Tegen, Daniela Thrän, Josefine Umlauft, Manfred Wendisch, Kevin Wolf, Christian Wirth, Hannes Zacher, Sönke Zaehle, Johannes Quaas:
A Belowground Perspective on the Nexus Between Biodiversity Change, Climate Change, and Human Well-being.
Journal of Sustainable Agriculture and Environment. 3 (2) : pp. e212108. 2024.
[bibtex] [web] [doi] [abstract]
A Belowground Perspective on the Nexus Between Biodiversity Change, Climate Change, and Human Well-being.
Journal of Sustainable Agriculture and Environment. 3 (2) : pp. e212108. 2024.
[bibtex] [web] [doi] [abstract]
Abstract Soil is central to the complex interplay among biodiversity, climate, and society. This paper examines the interconnectedness of soil biodiversity, climate change, and societal impacts, emphasizing the urgent need for integrated solutions. Human-induced biodiversity loss and climate change intensify environmental degradation, threatening human well-being. Soils, rich in biodiversity and vital for ecosystem function regulation, are highly vulnerable to these pressures, affecting nutrient cycling, soil fertility, and resilience. Soil also crucially regulates climate, influencing energy, water cycles, and carbon storage. Yet, climate change poses significant challenges to soil health and carbon dynamics, amplifying global warming. Integrated approaches are essential, including sustainable land management, policy interventions, technological innovations, and societal engagement. Practices like agroforestry and organic farming improve soil health and mitigate climate impacts. Effective policies and governance are crucial for promoting sustainable practices and soil conservation. Recent technologies aid in monitoring soil biodiversity and implementing sustainable land management. Societal engagement, through education and collective action, is vital for environmental stewardship. By prioritizing interdisciplinary research and addressing key frontiers, scientists can advance understanding of the soil biodiversity–climate change–society nexus, informing strategies for environmental sustainability and social equity.
Wasim Ahmad, Maha Shadaydeh, Joachim Denzler:
Deep Learning-based Group Causal Inference in Multivariate Time-series.
AAAI Workshop on AI for Time-series (AAAI-WS). 2024.
[bibtex] [pdf] [web] [abstract]
Deep Learning-based Group Causal Inference in Multivariate Time-series.
AAAI Workshop on AI for Time-series (AAAI-WS). 2024.
[bibtex] [pdf] [web] [abstract]
Causal inference in a nonlinear system of multivariate time series is instrumental in disentangling the intricate web of relationships among variables, enabling us to make more accurate predictions and gain deeper insights into real-world complex systems. Causality methods typically identify the causal structure of a multivariate system by considering the cause-effect relationship of each pair of variables while ignoring the collective effect of a group of variables or interactions involving more than two-time series variables. In this work, we test model invariance by group-level interventions on the trained deep networks to infer causal direction in groups of variables, such as climate and ecosystem, brain networks, etc. Extensive testing with synthetic and real-world time series data shows a significant improvement of our method over other applied group causality methods and provides us insights into real-world time series. The code for our method can be found at: https://github.com/wasimahmadpk/gCause.
Wasim Ahmad, Maha Shadaydeh, Joachim Denzler:
Regime Identification for Improving Causal Analysis in Non-stationary Timeseries.
arXiv preprint arXiv:2405.02315. 2024.
[bibtex] [pdf] [web] [abstract]
Regime Identification for Improving Causal Analysis in Non-stationary Timeseries.
arXiv preprint arXiv:2405.02315. 2024.
[bibtex] [pdf] [web] [abstract]
Time series data from real-world systems often display non-stationary behavior, indicating varying statistical characteristics over time. This inherent variability poses significant challenges in deciphering the underlying structural relationships within the data, particularly in correlation and causality analyses, model stability, etc. Recognizing distinct segments or regimes within multivariate time series data, characterized by relatively stable behavior and consistent statistical properties over extended periods, becomes crucial. In this study, we apply the regime identification (RegID) technique to multivariate time series, fundamentally designed to unveil locally stationary segments within data. The distinguishing features between regimes are identified using covariance matrices in a Riemannian space. We aim to highlight how regime identification contributes to improving the discovery of causal structures from multivariate non-stationary time series data. Our experiments, encompassing both synthetic and real-world datasets, highlight the effectiveness of regime-wise time series causal analysis. We validate our approach by first demonstrating improved causal structure discovery using synthetic data where the ground truth causal relationships are known. Subsequently, we apply this methodology to climate-ecosystem dataset, showcasing its applicability in real-world scenarios.
Wasim Ahmad, Valentin Kasburg, Nina Kukowski, Maha Shadaydeh, Joachim Denzler:
Deep-Learning Based Causal Inference: A Feasibility Study Based on Three Years of Tectonic-Climate Data From Moxa Geodynamic Observatory.
Earth and Space Science. 2024. (accepted)
[bibtex] [abstract]
Deep-Learning Based Causal Inference: A Feasibility Study Based on Three Years of Tectonic-Climate Data From Moxa Geodynamic Observatory.
Earth and Space Science. 2024. (accepted)
[bibtex] [abstract]
Highly sensitive laser strainmeters at Moxa Geodynamic Observatory (MGO) measure motions of the upper Earth's crust. Since the mountain overburden of the laser strainmeters installed in the gallery of the observatory is relatively low, the recorded time series are strongly influenced by local meteorological phenomena. To estimate the nonlinear effect of the meteorological variables on strain measurements in a non-stationary environment, advanced methods capable of learning the nonlinearity and discovering causal relationships in the non-stationary multivariate tectonic-climate time series are needed. Methods for causal inference generally perform well in identifying linear causal relationships but often struggle to retrieve complex nonlinear causal structures prevalent in real-world systems. This work presents a novel model invariance-based causal discovery (CDMI) method that utilizes deep networks to model nonlinearity in a multivariate time series system. We propose to use the theoretically well-established Knockoffs framework to generate in-distribution, uncorrelated copies of the original data as interventional variables and test the model invariance for causal discovery. To deal with the non-stationary behavior of the tectonic-climate time series recorded at the MGO, we propose a regime identification approach that we apply before causal analysis to generate segments of time series that possess locally consistent statistical properties. First, we evaluate our method on synthetically generated time series by comparing it to other causal analysis methods. We then investigate the hypothesized effect of meteorological variables on strain measurements. Our approach outperforms other causality methods and provides meaningful insights into tectonic-climate causal interactions.
2023
Dimitri Korsch, Maha Shadaydeh, Joachim Denzler:
Simplified Concrete Dropout - Improving the Generation of Attribution Masks for Fine-grained Classification.
DAGM German Conference on Pattern Recognition (DAGM-GCPR). 2023.
[bibtex] [pdf] [code] [supplementary] [abstract]
Simplified Concrete Dropout - Improving the Generation of Attribution Masks for Fine-grained Classification.
DAGM German Conference on Pattern Recognition (DAGM-GCPR). 2023.
[bibtex] [pdf] [code] [supplementary] [abstract]
Fine-grained classification is a particular case of a classification problem, aiming to classify objects that share the visual appearance and can only be distinguished by subtle differences. Fine-grained classification models are often deployed to determine animal species or individuals in automated animal monitoring systems. Precise visual explanations of the model's decision are crucial to analyze systematic errors. Attention- or gradient-based methods are commonly used to identify regions in the image that contribute the most to the classification decision. These methods deliver either too coarse or too noisy explanations, unsuitable for identifying subtle visual differences reliably. However, perturbation-based methods can precisely identify pixels causally responsible for the classification result. Fill-in of the dropout (FIDO) algorithm is one of those methods. It utilizes the concrete dropout (CD) to sample a set of attribution masks and updates the sampling parameters based on the output of the classification model. A known problem of the algorithm is a high variance in the gradient estimates, which the authors have mitigated until now by mini-batch updates of the sampling parameters. This paper presents a solution to circumvent these computational instabilities by simplifying the CD sampling and reducing reliance on large mini-batch sizes. First, it allows estimating the parameters with smaller mini-batch sizes without losing the quality of the estimates but with a reduced computational effort. Furthermore, our solution produces finer and more coherent attribution masks. Finally, we use the resulting attribution masks to improve the classification performance of a trained model without additional fine-tuning of the model.
Yuanyuan Huang, Gideon Stein, Olaf Kolle, Karl Kuebler, Ernst-Detlef Schulze, Hui Dong, David Eichenberg, Gerd Gleixner, Anke Hildebrandt, Markus Lange, Christiane Roscher, Holger Schielzeth, Bernhard Schmid, Alexandra Weigelt, Wolfgang W. Weisser, Maha Shadaydeh, Joachim Denzler, Anne Ebeling, Nico Eisenhauer:
Enhanced Stability of Grassland Soil Temperature by Plant Diversity.
Nature Geoscience. pp. 1-7. 2023.
[bibtex] [doi] [abstract]
Enhanced Stability of Grassland Soil Temperature by Plant Diversity.
Nature Geoscience. pp. 1-7. 2023.
[bibtex] [doi] [abstract]
Extreme weather events are occurring more frequently, and research has shown that plant diversity can help mitigate the impacts of climate change by increasing plant productivity and ecosystem stability. Although soil temperature and its stability are key determinants of essential ecosystem processes, no study has yet investigated whether plant diversity buffers soil temperature fluctuations over long-term community development. Here we have conducted a comprehensive analysis of a continuous 18-year dataset from a grassland biodiversity experiment with high spatial and temporal resolutions. Our findings reveal that plant diversity acts as a natural buffer, preventing soil heating in hot weather and cooling in cold weather. This diversity effect persists year-round, intensifying with the aging of experimental communities and being even stronger under extreme climate conditions, such as hot days or dry years. Using structural equation modelling, we found that plant diversity stabilizes soil temperature by increasing soil organic carbon concentrations and, to a lesser extent, plant leaf area index. Our results suggest that, in lowland grasslands, the diversity-induced stabilization of soil temperature may help to mitigate the negative effects of extreme climatic events such as soil carbon decomposition, thus slowing global warming.
Yuanyuan Huang, Gideon Stein, Olaf Kolle, Karl Kuebler, Ernst-Detlef Schulze, Hui Dong, David Eichenberg, Gerd Gleixner, Anke Hildebrandt, Markus Lange, Christiane Roscher, Holger Schielzeth, Bernhard Schmid, Alexandra Weigelt, Wolfgang W. Weisser, Maha Shadaydeh, Joachim Denzler, Anne Ebeling, Nico Eisenhauer:
Plant Diversity Stabilizes Soil Temperature.
bioRxiv. pp. 2023-03. 2023.
[bibtex] [pdf]
Plant Diversity Stabilizes Soil Temperature.
bioRxiv. pp. 2023-03. 2023.
[bibtex] [pdf]
2022
Cem Doǧdu, Thomas Kessler, Dana Schneider, Maha Shadaydeh, Stefan R. Schweinberger:
A Comparison of Machine Learning Algorithms and Feature Sets for Automatic Vocal Emotion Recognition in Speech.
Sensors. 22 (19) : 2022.
[bibtex] [web] [doi] [abstract]
A Comparison of Machine Learning Algorithms and Feature Sets for Automatic Vocal Emotion Recognition in Speech.
Sensors. 22 (19) : 2022.
[bibtex] [web] [doi] [abstract]
Vocal emotion recognition (VER) in natural speech, often referred to as speech emotion recognition (SER), remains challenging for both humans and computers. Applied fields including clinical diagnosis and intervention, social interaction research or Human Computer Interaction (HCI) increasingly benefit from efficient VER algorithms. Several feature sets were used with machine-learning (ML) algorithms for discrete emotion classification. However, there is no consensus for which low-level-descriptors and classifiers are optimal. Therefore, we aimed to compare the performance of machine-learning algorithms with several different feature sets. Concretely, seven ML algorithms were compared on the Berlin Database of Emotional Speech: Multilayer Perceptron Neural Network (MLP), J48 Decision Tree (DT), Support Vector Machine with Sequential Minimal Optimization (SMO), Random Forest (RF), k-Nearest Neighbor (KNN), Simple Logistic Regression (LOG) and Multinomial Logistic Regression (MLR) with 10-fold cross validation using four openSMILE feature sets (i.e., IS-09, emobase, GeMAPS and eGeMAPS). Results indicated that SMO, MLP and LOG show better performance (reaching to 87.85%, 84.00% and 83.74% accuracies, respectively) compared to RF, DT, MLR and KNN (with minimum 73.46%, 53.08%, 70.65% and 58.69% accuracies, respectively). Overall, the emobase feature set performed best. We discuss the implications of these findings for applications in diagnosis, intervention or HCI.
Clemence Dubois, Jannik Jänichen, Maha Shadaydeh, Gideon Stein, Alexandra Katz, Daniel Klöpper, Joachim Denzler, Christiane Schmullius, Katja Last:
KI4KI: Neues Projekt zur regelmässigen Überwachung von Stauanlagen aus dem All.
Messtechnische Überwachung von Stauanlagen ; XII.Mittweidaer Talsperrentag. Pages 15-19. 2022.
[bibtex] [web] [doi] [abstract]
KI4KI: Neues Projekt zur regelmässigen Überwachung von Stauanlagen aus dem All.
Messtechnische Überwachung von Stauanlagen ; XII.Mittweidaer Talsperrentag. Pages 15-19. 2022.
[bibtex] [web] [doi] [abstract]
Die Überwachung von Staubauwerken stellt Stauanlagenbetreiber vor viele Herausforderungen. Insbesondere aufgrund der Kosten und des Zeitaufwandes werden Staubauwerke oft nur ein- bis zweimal im Jahr durch trigonometrische Messungen überwacht. Seit einigen Jahrzehnten liefern jedoch Radarsatellitendaten nützliche Informationen zum Infrastrukturmonitoring. Satellitendaten der Copernicus Sentinel-1 Mission erlauben es, mittels der Technik der Persistent Scatterer Interferometrie (PSI), Deformationsmessungen von Staubauwerken im Millimeterbereich mit einem zeitlichen Abstand von 6 bis 12 Tagen durchzuführen. In einem Verbundprojekt zwischen der Friedrich-Schiller-Universität Jena und dem Ruhrverband soll ein Dienst entwickelt werden, der bisherige Überwachungsstrategien der Anlagen durch Nutzung der PSI Technik verbessert. Zudem sollen neuartige Geräte genutzt werden, die die Sichtbarkeit der Stauanlagen im Satellitenbild erhöhen sowie Methoden der künstlichen Intelligenz genutzt werden, um Deformationen im Falle von Extremwetterereignissen besser vorhersagen zu können.
Maha Shadaydeh, Joachim Denzler, Mirco Migliavacca:
Partitioning of Net Ecosystem Exchange Using Dynamic Mode Decomposition and Time Delay Embedding.
Engineering Proceedings. 18 (1) : 2022.
[bibtex] [web] [doi] [abstract]
Partitioning of Net Ecosystem Exchange Using Dynamic Mode Decomposition and Time Delay Embedding.
Engineering Proceedings. 18 (1) : 2022.
[bibtex] [web] [doi] [abstract]
Ecosystem respiration (Reco) represents a major component of the global carbon cycle. An accurate estimation of Reco dynamics is necessary for a better understanding of ecosystem-climate interactions and the impact of climate extremes on ecosystems. This paper proposes a new data-driven method for the estimation of the nonlinear dynamics of Reco using the method of dynamic mode decomposition with control input (DMDc). The method is validated on the half-hourly Fluxnet 2015 data. The model is first trained on the night-time net ecosystem exchange data. The day-time Reco values are then predicted using the obtained model with future values of a control input such as air temperature and soil water content. To deal with unobserved drivers of Reco other than the user control input, the method uses time-delay embedding of the history of Reco and the control input. Results indicate that, on the one hand, the prediction accuracy of Reco dynamics using DMDc is comparable to state-of-the-art deep learning-based methods, yet it has the advantages of being a simple and almost hyper-parameter-free method with a low computational load. On the other hand, the study of the impact of different control inputs on Reco dynamics showed that for most of the studied Fluxnet sites, air temperature is a better long-term predictor of Reco, while using soil water content as control input produced better short-term prediction accuracy.
Sven Festag, Gideon Stein, Tim Büchner, Maha Shadaydeh, Joachim Denzler, Cord Spreckelsen:
Outcome Prediction and Murmur Detection in Sets of Phonocardiograms by a Deep Learning-Based Ensemble Approach.
Computing in Cardiology (CinC). Pages 1-4. 2022.
[bibtex] [pdf] [doi] [abstract]
Outcome Prediction and Murmur Detection in Sets of Phonocardiograms by a Deep Learning-Based Ensemble Approach.
Computing in Cardiology (CinC). Pages 1-4. 2022.
[bibtex] [pdf] [doi] [abstract]
We, the team UKJ_FSU, propose a deep learning system for the prediction of congenital heart diseases. Our method is able to predict the clinical outcomes (normal, abnormal) of patients as well as to identify heart murmur (present, absent, unclear) based on phonocardiograms recorded at different auscultation locations. The system we propose is an ensemble of four temporal convolutional networks with identical topologies, each specialized in identifying murmurs and predicting patient outcome from a phonocardiogram taken at one specific auscultation location. Their intermediate outputs are augmented by the manually ascertained patient features such as age group, sex, height, and weight. The outputs of the four networks are combined to form a single final decision as demanded by the rules of the George B. Moody PhysioNet Challenge 2022. On the first task of this challenge, the murmur detection, our model reached a weighted accuracy of 0.567 with respect to the validation set. On the outcome prediction task (second task) the ensemble led to a mean outcome cost of 10679 on the same set. By focusing on the clinical outcome prediction and tuning some of the hyper-parameters only for this task, our model reached a cost score of 12373 on the official test set (rank 13 of 39). The same model scored a weighted accuracy of 0.458 regarding the murmur detection on the test set (rank 37 of 40).
Violeta Teodora Trifunov, Maha Shadaydeh, Joachim Denzler:
Sequential Causal Effect Variational Autoencoder: Time Series Causal Link Estimation under Hidden Confounding.
arXiv preprint arXiv:2209.11497. 2022.
[bibtex] [web] [doi] [abstract]
Sequential Causal Effect Variational Autoencoder: Time Series Causal Link Estimation under Hidden Confounding.
arXiv preprint arXiv:2209.11497. 2022.
[bibtex] [web] [doi] [abstract]
Estimating causal effects from observational data in the presence of latent variables sometimes leads to spurious relationships which can be misconceived as causal. This is an important issue in many fields such as finance and climate science. We propose Sequential Causal Effect Variational Autoencoder (SCEVAE), a novel method for time series causality analysis under hidden confounding. It is based on the CEVAE framework and recurrent neural networks. The causal link's intensity of the confounded variables is calculated by using direct causal criteria based on Pearl's do-calculus. We show the efficacy of SCEVAE by applying it to synthetic datasets with both linear and nonlinear causal links. Furthermore, we apply our method to real aerosol-cloud-climate observation data. We compare our approach to a time series deconfounding method with and without substitute confounders on the synthetic data. We demonstrate that our method performs better by comparing both methods to the ground truth. In the case of real data, we use the expert knowledge of causal links and show how the use of correct proxy variables aids data reconstruction.
Violeta Teodora Trifunov, Maha Shadaydeh, Joachim Denzler:
Time Series Causal Link Estimation under Hidden Confounding using Knockoff Interventions.
NeurIPS Workshop on A Causal View on Dynamical Systems (NeurIPS-WS). 2022.
[bibtex] [pdf] [web] [abstract]
Time Series Causal Link Estimation under Hidden Confounding using Knockoff Interventions.
NeurIPS Workshop on A Causal View on Dynamical Systems (NeurIPS-WS). 2022.
[bibtex] [pdf] [web] [abstract]
Latent variables often mask cause-effect relationships in observational data which provokes spurious links that may be misinterpreted as causal. This problem sparks great interest in the fields such as climate science and economics. We propose to estimate confounded causal links of time series using Sequential Causal Effect Variational Autoencoder (SCEVAE) while applying knockoff interventions. We show the advantage of knockoff interventions by applying SCEVAE to synthetic datasets with both linear and nonlinear causal links. Moreover, we apply SCEVAE with knockoffs to real aerosol-cloud-climate observational time series data. We compare our results on synthetic data to those of a time series deconfounding method both with and without estimated confounders. We show that our method outperforms this benchmark by comparing both methods to the ground truth. For the real data analysis, we rely on expert knowledge of causal links and demonstrate how using suitable proxy variables improves the causal link estimation in the presence of hidden confounders.
Wasim Ahmad, Maha Shadaydeh, Joachim Denzler:
Causal Discovery using Model Invariance through Knockoff Interventions.
ICML Workshop on Spurious Correlations, Invariance and Stability (ICML-WS). 2022.
[bibtex] [pdf] [web] [abstract]
Causal Discovery using Model Invariance through Knockoff Interventions.
ICML Workshop on Spurious Correlations, Invariance and Stability (ICML-WS). 2022.
[bibtex] [pdf] [web] [abstract]
Cause-effect analysis is crucial to understand the underlying mechanism of a system. We propose to exploit model invariance through interventions on the predictors to infer causality in nonlinear multivariate systems of time series. We model nonlinear interactions in time series using DeepAR and then expose the model to different environments using Knockoffs-based interventions to test model invariance. Knockoff samples are pairwise exchangeable, in-distribution and statistically null variables generated without knowing the response. We test model invariance where we show that the distribution of the response residual does not change significantly upon interventions on non-causal predictors. We evaluate our method on real and synthetically generated time series. Overall our method outperforms other widely used causality methods, i.e, VAR Granger causality, VARLiNGAM and PCMCI+.
2021
Maha Shadaydeh, Lea Müller, Dana Schneider, Martin Thümmel, Thomas Kessler, Joachim Denzler:
Analyzing the Direction of Emotional Influence in Nonverbal Dyadic Communication: A Facial-Expression Study.
IEEE Access. 9 : pp. 73780-73790. 2021.
[bibtex] [pdf] [web] [doi] [presentation] [abstract]
Analyzing the Direction of Emotional Influence in Nonverbal Dyadic Communication: A Facial-Expression Study.
IEEE Access. 9 : pp. 73780-73790. 2021.
[bibtex] [pdf] [web] [doi] [presentation] [abstract]
Identifying the direction of emotional influence in a dyadic dialogue is of increasing interest in the psychological sciences with applications in psychotherapy, analysis of political interactions, or interpersonal conflict behavior. Facial expressions are widely described as being automatic and thus hard to be overtly influenced. As such, they are a perfect measure for a better understanding of unintentional behavior cues about socio-emotional cognitive processes. With this view, this study is concerned with the analysis of the direction of emotional influence in dyadic dialogues based on facial expressions only. We exploit computer vision capabilities along with causal inference theory for quantitative verification of hypotheses on the direction of emotional influence, i.e., cause-effect relationships, in dyadic dialogues. We address two main issues. First, in a dyadic dialogue, emotional influence occurs over transient time intervals and with intensity and direction that are variant over time. To this end, we propose a relevant interval selection approach that we use prior to causal inference to identify those transient intervals where causal inference should be applied. Second, we propose to use fine-grained facial expressions that are present when strong distinct facial emotions are not visible. To specify the direction of influence, we apply the concept of Granger causality to the time-series of facial expressions over selected relevant intervals. We tested our approach on newly, experimentally obtained data. Based on quantitative verification of hypotheses on the direction of emotional influence, we were able to show that the proposed approach is promising to reveal the cause-effect pattern in various instructed interaction conditions.
Oana-Iuliana Popescu, Maha Shadaydeh, Joachim Denzler:
Counterfactual Generation with Knockoffs.
arXiv preprint arXiv:2102.00951. 2021.
[bibtex] [pdf] [web] [abstract]
Counterfactual Generation with Knockoffs.
arXiv preprint arXiv:2102.00951. 2021.
[bibtex] [pdf] [web] [abstract]
Human interpretability of deep neural networks' decisions is crucial, especially in domains where these directly affect human lives. Counterfactual explanations of already trained neural networks can be generated by perturbing input features and attributing importance according to the change in the classifier's outcome after perturbation. Perturbation can be done by replacing features using heuristic or generative in-filling methods. The choice of in-filling function significantly impacts the number of artifacts, i.e., false-positive attributions. Heuristic methods result in false-positive artifacts because the image after the perturbation is far from the original data distribution. Generative in-filling methods reduce artifacts by producing in-filling values that respect the original data distribution. However, current generative in-filling methods may also increase false-negatives due to the high correlation of in-filling values with the original data. In this paper, we propose to alleviate this by generating in-fillings with the statistically-grounded Knockoffs framework, which was developed by Barber and Candès in 2015 as a tool for variable selection with controllable false discovery rate. Knockoffs are statistically null-variables as decorrelated as possible from the original data, which can be swapped with the originals without changing the underlying data distribution. A comparison of different in-filling methods indicates that in-filling with knockoffs can reveal explanations in a more causal sense while still maintaining the compactness of the explanations.
Violeta Teodora Trifunov, Maha Shadaydeh, Björn Barz, Joachim Denzler:
Anomaly Attribution of Multivariate Time Series using Counterfactual Reasoning.
IEEE International Conference on Machine Learning and Applications (ICMLA). Pages 166-172. 2021.
[bibtex] [pdf] [web] [doi] [abstract]
Anomaly Attribution of Multivariate Time Series using Counterfactual Reasoning.
IEEE International Conference on Machine Learning and Applications (ICMLA). Pages 166-172. 2021.
[bibtex] [pdf] [web] [doi] [abstract]
There are numerous methods for detecting anomalies in time series, but that is only the first step to understanding them. We strive to exceed this by explaining those anomalies. Thus we develop a novel attribution scheme for multivariate time series relying on counterfactual reasoning. We aim to answer the counterfactual question of would the anomalous event have occurred if the subset of the involved variables had been more similarly distributed to the data outside of the anomalous interval. Specifically, we detect anomalous intervals using the Maximally Divergent Interval (MDI) algorithm, replace a subset of variables with their in-distribution values within the detected interval and observe if the interval has become less anomalous, by re-scoring it with MDI. We evaluate our method on multivariate temporal and spatio-temporal data and confirm the accuracy of our anomaly attribution of multiple well-understood extreme climate events such as heatwaves and hurricanes.
Violeta Teodora Trifunov, Maha Shadaydeh, Jakob Runge, Markus Reichstein, Joachim Denzler:
A Data-Driven Approach to Partitioning Net Ecosystem Exchange Using a Deep State Space Model.
IEEE Access. 9 : pp. 107873-107883. 2021.
[bibtex] [abstract]
A Data-Driven Approach to Partitioning Net Ecosystem Exchange Using a Deep State Space Model.
IEEE Access. 9 : pp. 107873-107883. 2021.
[bibtex] [abstract]
Describing ecosystem carbon fluxes is essential for deepening the understanding of the Earth system. However, partitioning net ecosystem exchange (NEE), i.e. the sum of ecosystem respiration (Reco) and gross primary production (GPP), into these summands is ill-posed since there can be infinitely many mathematically-valid solutions. We propose a novel data-driven approach to NEE partitioning using a deep state space model which combines the interpretability and uncertainty analysis of state space models with the ability of recurrent neural networks to learn the complex functions governing the data. We validate our proposed approach on the FLUXNET dataset. We suggest using both the past and the future of Reco’s predictors for training along with the nighttime NEE (NEEnight) to learn a dynamical model of Reco. We evaluate our nighttime Reco forecasts by comparing them to the ground truth NEEnight and obtain the best accuracy with respect to other partitioning methods. The learned nighttime Reco model is then used to forecast the daytime Reco conditioning on the future observations of different predictors, i.e., global radiation, air temperature, precipitation, vapor pressure deficit, and daytime NEE (NEEday). Subtracted from the NEEday, these estimates yield the GPP, finalizing the partitioning. Our purely data-driven daytime Reco forecasts are in line with the recent empirical partitioning studies reporting lower daytime Reco than the Reichstein method, which can be attributed to the Kok effect, i.e., the plant respiration being higher at night. We conclude that our approach is a good alternative for data-driven NEE partitioning and complements other partitioning methods.
Wasim Ahmad, Maha Shadaydeh, Joachim Denzler:
Causal Inference in Non-linear Time-series using Deep Networks and Knockoff Counterfactuals.
IEEE International Conference on Machine Learning and Applications (ICMLA). Pages 449-454. 2021.
[bibtex] [pdf] [web] [doi] [abstract]
Causal Inference in Non-linear Time-series using Deep Networks and Knockoff Counterfactuals.
IEEE International Conference on Machine Learning and Applications (ICMLA). Pages 449-454. 2021.
[bibtex] [pdf] [web] [doi] [abstract]
Estimating causal relations is vital in understanding the complex interactions in multivariate time series. Non-linear coupling of variables is one of the major challenges in accurate estimation of cause-effect relations. In this paper, we propose to use deep autoregressive networks (DeepAR) in tandem with counterfactual analysis to infer nonlinear causal relations in multivariate time series. We extend the concept of Granger causality using probabilistic forecasting with DeepAR. Since deep networks can neither handle missing input nor out-of-distribution intervention, we propose to use the Knockoffs framework (Barber and Candes, 2015) for generating intervention variables and consequently counterfactual probabilistic forecasting. Knockoff samples are independent of their output given the observed variables and exchangeable with their counterpart variables without changing the underlying distribution of the data. We test our method on synthetic as well as real-world time series datasets. Overall our method outperforms the widely used vector autoregressive Granger causality and PCMCI in detecting nonlinear causal dependency in multivariate time series.
2020
Maha Shadaydeh, Yanira Guanche Garcia, Miguel Mahecha, Joachim Denzler:
Understanding Changes in Environmental Time Series with Time-frequency Causality Analysis.
European Geosciences Union General Assembly (EGU): Abstract + Poster Presentation. 2020.
[bibtex] [pdf] [web] [abstract]
Understanding Changes in Environmental Time Series with Time-frequency Causality Analysis.
European Geosciences Union General Assembly (EGU): Abstract + Poster Presentation. 2020.
[bibtex] [pdf] [web] [abstract]
Understanding causal effect relationships between the different variables in dynamical systems is an important and challenging problem in different areas of research such as attribution of climate change, brain neural connectivity analysis, psychology, among many others. These relationships are guided by the process generating them. Hence, detecting changes or new patterns in the causal effect relationships can be used not only for the detection but also for the diagnosis and attribution of changes in the underlying process.Time series of environmental time series most often contain multiple periodical components, e.g. daily and seasonal cycles, induced by the meteorological forcing variables. This can significantly mask the underlying endogenous causality structure when using time-domain analysis and therefore results in several spurious links. Filtering these periodic components as preprocessing step might degrade causal inference. This motivates the use of time-frequency processing techniques such as Wavelet or short-time Fourier transform where the causality structure can be examined at each frequency component and on multiple time scales.In this study, we use a parametric time-frequency representation of vector autoregressive Granger causality for causal inference. We first show that causal inference using time-frequency domain analysis outperforms time-domain analysis when dealing with time series that contain periodic components, trends, or noise. The proposed approach allows for the estimation of the causal effect interaction between each pair of variables in the system on multiple time scales and hence for excluding links that result from periodic components.Second, we investigate whether anomalous events can be identified based on the observed changes in causal relationships. We consider two representative examples in environmental systems: land-atmosphere ecosystem and marine climate. Through these two examples, we show that an anomalous event can indeed be identified as the event where the causal intensities differ according to a distance measure from the average causal intensities. Two different methods are used for testing the statistical significance of the causal-effect intensity at each frequency component.Once the anomalous event is detected, the driver of the event can be identified based on the analysis of changes in the obtained causal effect relationships during the time duration of the event and consequently provide an explanation of the detected anomalous event. Current research efforts are directed towards the extension of this work by using nonlinear state-space models, both statistical and deep learning-based ones.
Sheeba Samuel, Maha M Month = February fShadaydeh, Sebastian Böcker, Bernd Brügmann, Solveig F. Bucher, Volker Deckert, Joachim Denzler, Peter Dittrich, Ferdinand von Eggeling, Daniel Güllmar, Orlando Guntinas-Lichius, Birgitta König-Ries, Frank Löffler, Lutz Maicher, Manja Marz, Mirco Migliavacca, Jürgen R. Reichenbach, Markus Reichstein, Christine Römermann, Andrea Wittig:
A Virtual "Werkstatt" for Digitization in the Sciences.
Research Ideas and Outcomes. 6 : pp. e54106. 2020.
[bibtex] [web] [doi] [abstract]
A Virtual "Werkstatt" for Digitization in the Sciences.
Research Ideas and Outcomes. 6 : pp. e54106. 2020.
[bibtex] [web] [doi] [abstract]
Data is central in almost all scientific disciplines nowadays. Furthermore, intelligent systems have developed rapidly in recent years, so that in many disciplines the expectation is emerging that with the help of intelligent systems, significant challenges can be overcome and science can be done in completely new ways. In order for this to succeed, however, first, fundamental research in computer science is still required, and, second, generic tools must be developed on which specialized solutions can be built. In this paper, we introduce a recently started collaborative project funded by the Carl Zeiss Foundation, a virtual manufactory for digitization in the sciences, the "Werkstatt", which is being established at the Michael Stifel Center Jena (MSCJ) for data-driven and simulation science to address fundamental questions in computer science and applications. The Werkstatt focuses on three key areas, which include generic tools for machine learning, knowledge generation using machine learning processes, and semantic methods for the data life cycle, as well as the application of these topics in different disciplines. Core and pilot projects address the key aspects of the topics and form the basis for sustainable work in the Werkstatt.
2019
Furrukh Sana, Tarig Ballal, Maha Shadaydeh, Ibrahim Hoteit, Tareq Y. Al-Naffouri:
Fetal ECG extraction exploiting joint sparse supports in a dual dictionary framework.
Biomedical Signal Processing and Control. 48 : pp. 46-60. 2019.
[bibtex] [pdf] [web] [doi] [abstract]
Fetal ECG extraction exploiting joint sparse supports in a dual dictionary framework.
Biomedical Signal Processing and Control. 48 : pp. 46-60. 2019.
[bibtex] [pdf] [web] [doi] [abstract]
Electrocardiogram (ECG) signals are vital tools in assessing the health of the mother and the fetus during pregnancy. Extraction of fetal ECG (FECG) signal from the mother's abdominal recordings requires challenging signal processing tasks to eliminate the effects of the mother's ECG (MECG) signal, noise and other distortion sources. The availability of ECG data from multiple electrodes provides an opportunity to leverage the collective information in a collaborative manner. We propose a new scheme for extracting the fetal ECG signals from the abdominal ECG recordings of the mother using the multiple measurement vectors approach. The scheme proposes a dual dictionary framework that employs a learned dictionary for eliminating the MECG signals through sparse domain representation and a wavelet dictionary for the noise reduced sparse estimation of the fetal ECG signals. We also propose a novel methodology for inferring a single estimate of the fetal ECG source signal from the individual sensor estimates. Simulation results with real ECG recordings demonstrate that the proposed scheme provides a comprehensive framework for eliminating the mother's ECG component in the abdominal recordings, effectively filters out noise and distortions, and leads to more accurate recovery of the fetal ECG source signal compared to other state-of-the-art algorithms.
Lea Müller, Maha Shadaydeh, Martin Thümmel, Thomas Kessler, Dana Schneider, Joachim Denzler:
Causal Inference in Nonverbal Dyadic Communication with Relevant Interval Selection and Granger Causality.
International Conference on Computer Vision Theory and Applications (VISAPP). Pages 490-497. 2019.
[bibtex] [pdf] [web] [doi] [abstract]
Causal Inference in Nonverbal Dyadic Communication with Relevant Interval Selection and Granger Causality.
International Conference on Computer Vision Theory and Applications (VISAPP). Pages 490-497. 2019.
[bibtex] [pdf] [web] [doi] [abstract]
Human nonverbal emotional communication in dyadic dialogs is a process of mutual influence and adaptation. Identifying the direction of influence, or cause-effect relation between participants, is a challenging task due to two main obstacles. First, distinct emotions might not be clearly visible. Second, participants cause-effect relation is transient and variant over time. In this paper, we address these difficulties by using facial expressions that can be present even when strong distinct facial emotions are not visible. We also propose to apply a relevant interval selection approach prior to causal inference to identify those transient intervals where adaptation process occurs. To identify the direction of influence, we apply the concept of Granger causality to the time series of facial expressions on the set of relevant intervals. We tested our approach on synthetic data and then applied it to newly, experimentally obtained data. Here, we were able to show that a more sensitive facial expression detection algorithm and a relevant interval detection approach is most promising to reveal the cause-effect pattern for dyadic communication in various instructed interaction conditions.
Maha Shadaydeh, Joachim Denzler, Yanira Guanche Garcia, Miguel Mahecha:
Time-Frequency Causal Inference Uncovers Anomalous Events in Environmental Systems.
DAGM German Conference on Pattern Recognition (DAGM-GCPR). Pages 499-512. 2019.
[bibtex] [pdf] [doi] [abstract]
Time-Frequency Causal Inference Uncovers Anomalous Events in Environmental Systems.
DAGM German Conference on Pattern Recognition (DAGM-GCPR). Pages 499-512. 2019.
[bibtex] [pdf] [doi] [abstract]
Causal inference in dynamical systems is a challenge for dif- ferent research areas. So far it is mostly about understanding to what extent the underlying causal mechanisms can be derived from observed time series. Here we investigate whether anomalous events can also be identified based on the observed changes in causal relationships. We use a parametric time-frequency representation of vector autoregressive Granger causality for causal inference. The use of time-frequency ap- proach allows for dealing with the nonstationarity of the time series as well as for defining the time scale on which changes occur. We present two representative examples in environmental systems: land-atmosphere ecosystem and marine climate. We show that an anomalous event can be identified as the event where the causal intensities differ according to a distance measure from the average causal intensities. The driver of the anomalous event can then be identified based on the analysis of changes in the causal effect relationships.
Stefan Hoffmann, Clemens-Alexander Brust, Maha Shadaydeh, Joachim Denzler:
Registration of High Resolution Sar and Optical Satellite Imagery Using Fully Convolutional Networks.
International Geoscience and Remote Sensing Symposium (IGARSS). Pages 5152-5155. 2019.
[bibtex] [pdf] [doi] [abstract]
Registration of High Resolution Sar and Optical Satellite Imagery Using Fully Convolutional Networks.
International Geoscience and Remote Sensing Symposium (IGARSS). Pages 5152-5155. 2019.
[bibtex] [pdf] [doi] [abstract]
Multi-modal image registration is a crucial step when fusing images which show different physical/chemical properties of an object. Depending on the compared modalities and the used registration metric, this process exhibits varying reliability. We propose a deep metric based on a fully convo-lutional neural network (FCN). It is trained from scratch on SAR-optical image pairs to predict whether certain image areas are aligned or not. Tests on the affine registration of SAR and optical images showing suburban areas verify an enormous improvement of the registration accuracy in comparison to registration metrics that are based on mutual information (MI).
Violeta Teodora Trifunov, Maha Shadaydeh, Jakob Runge, Veronika Eyring, Markus Reichstein, Joachim Denzler:
Causal Link Estimation under Hidden Confounding in Ecological Time Series.
International Workshop on Climate Informatics (CI). 2019.
[bibtex] [pdf] [abstract]
Causal Link Estimation under Hidden Confounding in Ecological Time Series.
International Workshop on Climate Informatics (CI). 2019.
[bibtex] [pdf] [abstract]
Understanding the causes of natural phe- nomena is a subject of continuous interest in many research fields such as climate and environmental science. We address the problem of recovering nonlinear causal relationships between time series of ecological variables in the presence of a hidden confounder. We suggest a deep learning approach with domain knowledge integration based on the Causal Effect Variational Autoencoder (CEVAE) which we extend and apply to ecological time series. We compare our method’s performance to that of vector autoregressive Granger Causality (VAR-GC) to emphasize its benefits.
Violeta Teodora Trifunov, Maha Shadaydeh, Jakob Runge, Veronika Eyring, Markus Reichstein, Joachim Denzler:
Nonlinear Causal Link Estimation under Hidden Confounding with an Application to Time-Series Anomaly Detection.
DAGM German Conference on Pattern Recognition (DAGM-GCPR). Pages 261-273. 2019.
[bibtex] [pdf] [abstract]
Nonlinear Causal Link Estimation under Hidden Confounding with an Application to Time-Series Anomaly Detection.
DAGM German Conference on Pattern Recognition (DAGM-GCPR). Pages 261-273. 2019.
[bibtex] [pdf] [abstract]
Causality analysis represents one of the most important tasks when examining dynamical systems such as ecological time series. We propose to mitigate the problem of inferring nonlinear cause-effect de- pendencies in the presence of a hidden confounder by using deep learning with domain knowledge integration. Moreover, we suggest a time series anomaly detection approach using causal link intensity increase as an indicator of the anomaly. Our proposed method is based on the Causal Effect Variational Autoencoder (CEVAE) which we extend and apply to anomaly detection in time series. We evaluate our method on synthetic data having properties of ecological time series and compare to the vector autoregressive Granger causality (VAR-GC) baseline.
Yanira Guanche, Maha Shadaydeh, Miguel Mahecha, Joachim Denzler:
Attribution of Multivariate Extreme Events.
International Workshop on Climate Informatics (CI). 2019.
[bibtex] [pdf] [abstract]
Attribution of Multivariate Extreme Events.
International Workshop on Climate Informatics (CI). 2019.
[bibtex] [pdf] [abstract]
The detection of multivariate extreme events is crucial to monitor the Earth system and to analyze their impacts on ecosystems and society. Once an abnormal event is detected, the following natural question is: what is causing this anomaly? Answering this question we try to understand these anomalies, to explain why they happened. In a previous work, the authors presented a multivariate anomaly detection approach based on the combination of a vector autoregressive model and the Mahalanobis distance metric. In this paper, we present an approach for the attribution of the detected anomalous events based on the decomposition of the Mahalanobis distance. The decomposed form of this metric provides an answer to the question: how much does each variable contribute to this distance metric? The method is applied to the extreme events detected in the land-atmosphere exchange fluxes: Gross Primary Productivity, Latent Energy, Net Ecosystem Exchange, Sensible Heat and Terrestrial Ecosystem Respiration. The attribution results of the proposed method for different known historic events are presented and compared with the univariate Z-score attribution method.
2018
Maha Shadaydeh, Yanira Guanche Garcia, Joachim Denzler:
Classification of Spatiotemporal Marine Climate Patterns using Wavelet Coherence and Markov Random Field.
American Geophysical Union Fall Meeting (AGU): Abstract + Oral Presentation. 2018.
[bibtex] [web] [abstract]
Classification of Spatiotemporal Marine Climate Patterns using Wavelet Coherence and Markov Random Field.
American Geophysical Union Fall Meeting (AGU): Abstract + Oral Presentation. 2018.
[bibtex] [web] [abstract]
Sea condition characterization and classification is a widely studied topic but rather challenging one due to the spatial and temporal variability in marine climate. The aim of this study is to develop a data-driven method for the classification of marine climate patterns. The proposed method consists of two main steps: i) Feature extraction applied to the time series of each point of the grid independently. ii) Spatiotemporal classification applied on the obtained features over the entire study area. The causal intensity between coupled marine variables can be efficiently visualized at different time-scales using wavelet coherence. To this end, we extract a set of features from the statistically significant wavelet coherence of each pair of the used marine variables: significant wave height (hs), mean wave period (Tm), and wave direction (θm). The obtained features, in addition to the sea level pressure (SLP), over the entire study area are then treated as multi-channel images. For the spatiotemporal classification of these images, we first apply the unsupervised K-means clustering method on the images of each three consecutive time instances. The K clusters represent K different marine climate patterns. Markov Random Fields (MRFs) provide an effective methodology for integrating spatiotemporal dependency between adjacent points into the image classification process. In this study, we use a MRF model for defining the spatiotemporal extent of the detected marine climate patterns, with the statistics of the detected K clusters used for the initial training. Experimental results show that the proposed method allows for a practical classification of marine climate into representative patterns that can be used for an accurate characterization of sea conditions, the analysis of extreme events and its impacts along the coast. A case study in the North Sea will be presented using the coasDat dataset [1]. [1] Weisse, R., H. v. Storch, U. Callies, A. Chrastansky, F. Feser, I. Grabemann, H. Guenther, A. Pluess, Th. Stoye, J. Tellkamp, J. Winterfeldt and K. Woth, (2008): Regional meteo-marine reanalyses and climate change projections: Results for Northern Europe and potentials for coastal and offshore applications. Bull. Amer. Meteor. Soc., 90, 849-860.
Maha Shadaydeh, Yanira Guanche Garcia, Miguel Mahecha, Markus Reichstein, Joachim Denzler:
Analyzing the Time Variant Causality in Ecological Time Series: A Time-Frequency Approach.
International Conference on Ecological Informatics (ICEI). Pages 151-152. 2018.
[bibtex] [abstract]
Analyzing the Time Variant Causality in Ecological Time Series: A Time-Frequency Approach.
International Conference on Ecological Informatics (ICEI). Pages 151-152. 2018.
[bibtex] [abstract]
Attribution in ecosystems aims to identify the cause-effect relationships between the variables involved. The availability of high temporal resolution data along with the powerful computing platforms further enhance the capacity of data-driven methods in capturing the complex relationships between the variables of the underlying system. Time series of ecological variables most often contain different periodical components that can significantly mask the underling causality structure in time domain. This motivates the use of time-frequency processing techniques such as wavelet analysis or short time Fourier transform. In this study we present a time-frequency approach for causality analysis where the coupling between the variables is assumed to follow a locally time-invariant multivariate autoregressive (MVAR) model. We propose a sliding time window approach to examine the change of interactions, i.e. direction and strength of causality, between the different variables over seasons. The cause-effect relationships are extracted using the frequency domain representation of the MVAR Granger causality (MVAR-GC) [1,2] based on the generalized partial directed coherence (gPDC) [3]. We have first applied the proposed method to synthetic data to evaluate its sensitivity to different issues such as the selection of the model order, the sampling frequency, the absence of cause as well as the presence of non-linear coupling. The method is then applied to half-hourly meteorological observations and land flux eddy covariance data to investigate the causal-effect relationships between global radiation (Rg), air temperature (Tair), and the CO2 land fluxes: gross primary productivity (GPP), net ecosystem exchange (NEE) and ecosystem respiration (Reco). The results show that time-frequency analysis based on MVAR-GC has promising potential in identifying the time variant causality structure within these variables along with the main time delay between different cause- effect pairs. Further research work is currently going for the investigation of the selection criteria of the model order, the sampling frequency, and the size of the time window at different time scales of causality analysis. This study is carried out within the framework of the project BACI which in part aims at developing an attribution scheme for changes in ecosystem functioning and studying the impacts of these changes on biodiversity patterns.
Violeta Teodora Trifunov, Maha Shadaydeh, Jakob Runge, Veronika Eyring, Markus Reichstein, Joachim Denzler:
Domain knowledge integration for causality analysis of carbon-cycle variables.
American Geophysical Union Fall Meeting (AGU): Abstract + Poster Presentation. 2018.
[bibtex] [web] [abstract]
Domain knowledge integration for causality analysis of carbon-cycle variables.
American Geophysical Union Fall Meeting (AGU): Abstract + Poster Presentation. 2018.
[bibtex] [web] [abstract]
Climate data has been vastly accumulated over the past several years, making climate science one of the most data-rich domains. Despite the abundance of data to process, data science has not had a lot of impact on climate research so far, due to the fact that ample expert knowledge is rarely exploited. Furthermore, the complex nature and the continuously changing climate system both contribute to the slow data science advances in the field. This issue was shown to be amend- able through the development of data-driven methodologies that are guided by theory to constrain search, discover more meaningful patterns, and produce more accurate models [1]. Causality analysis represents one of the most important tasks in climate research, its principal difficulties being the often found non-linearities in the data, in addition to hidden causes of the observed phenomena. We propose to ameliorate the problem of determining causal-effect dependencies to a certain extent by using deep learning methods together with domain knowledge integration. The suggested method is to be based on the causal effect variational auto-encoders (CEVAE) [2] and applied to half-hourly meteorological observations and land flux eddy covariance data. This will allow for exploration of the causal-effect relationships between air temperature (Tair), global radiation (Rg) and the CO2 fluxes gross primary productivity (GPP), net ecosystem exchange (NEE) and ecosystem respiration (Reco). The aim of this study is to show whether prior domain knowledge could aid discovery of new causal relationships between certain carbon-cycle variables. In addition, the proposed method is presumed to find its application to similar problems, such as those related to CO2 concentration estimation and facilitate efforts towards better understanding of the Earth system.
Yanira Guanche Garcia, Maha Shadaydeh, Miguel Mahecha, Joachim Denzler:
Extreme anomaly event detection in biosphere using linear regression and a spatiotemporal MRF model.
Natural Hazards. pp. 1-19. 2018.
[bibtex] [pdf] [web] [doi] [abstract]
Extreme anomaly event detection in biosphere using linear regression and a spatiotemporal MRF model.
Natural Hazards. pp. 1-19. 2018.
[bibtex] [pdf] [web] [doi] [abstract]
Detecting abnormal events within time series is crucial for analyzing and understanding the dynamics of the system in many research areas. In this paper, we propose a methodology to detect these anomalies in multivariate environmental data. Five biosphere variables from a preliminary version of the Earth System Data Cube have been used in this study: Gross Primary Productivity, Latent Energy, Net Ecosystem Exchange, Sensible Heat and Terrestrial Ecosystem Respiration. To tackle the spatiotemporal dependencies of the biosphere variables, the proposed methodology after preprocessing the data is divided into two steps: a feature extraction step applied to each time series in the grid independently, followed by a spatiotemporal event detection step applied to the obtained novelty scores over the entire study area. The first step is based on the assumption that the time series of each variable can be represented by an autoregressive moving average (ARMA) process, and the anomalies are those time instances that are not well represented by the estimated ARMA model. The Mahalanobis distance of the ARMA models’ multivariate residuals is used as a novelty score. In the second step, the obtained novelty scores of the entire study are treated as time series of images. Markov random fields (MRFs) provide an effective and theoretically well-established methodology for integrating spatiotemporal dependency into the classification of image time series. In this study, the classification of the novelty score images into three classes, intense anomaly, possible anomaly, and normal, is performed using unsupervised K-means clustering followed by multi-temporal MRF segmentation applied recursively on the images of each consecutive \(L \ge \) 1 time steps. The proposed methodology was applied to an area covering Europe and Africa. Experimental results and validation based on known historic events show that the method is able to detect historic events and also provides a useful tool to define sensitive regions.
2017
Maha Shadaydeh, Andras Zlinszky, Andrea Manno-Kovacs, Tamas Sziranyi:
Wetland mapping by fusion of airborne laser scanning and multi-temporal multispectral satellite imagery.
International Journal of Remote Sensing. 38 (23) : pp. 7422-7440. 2017.
[bibtex] [pdf] [web] [doi] [abstract]
Wetland mapping by fusion of airborne laser scanning and multi-temporal multispectral satellite imagery.
International Journal of Remote Sensing. 38 (23) : pp. 7422-7440. 2017.
[bibtex] [pdf] [web] [doi] [abstract]
Wetlands play a major role in Europe’s biodiversity. Despite their importance, wetlands are suffering from constant degradation and loss, therefore, they require constant monitoring. This article presents an automatic method for the mapping and monitoring of wetlands based on the fused processing of laser scans and multispectral satellite imagery, with validations and evaluations performed over an area of Lake Balaton in Hungary. Markov Random Field models have already been shown to successfully integrate various image properties in several remote sensing applications. In this article, we propose the multi-layer fusion Markov Random Field model for classifying wetland areas, built into an automatic classification process that combines multi-temporal multispectral images with a wetland classification reference map derived from airborne laser scanning (ALS) data acquired in an earlier year. Using an ALS-based wetland classification map that relied on a limited amount of ground truthing proved to improve the discrimination of land-cover classes with similar spectral characteristics. Based on the produced classifications, we also present an unsupervised method to track temporal changes of wetland areas by comparing the class labellings of different time layers. During the evaluations, the classification model is validated against manually interpreted independent aerial orthoimages. The results show that the proposed fusion model performs better than solely image-based processing, producing a non-supervised/semi-supervised wetland classification accuracy of 81-93 \% observed over different years.
Muzammil Behzad, Mudassir Masood, Tarig Ballal, Maha Shadaydeh, Tareq. Y. Al-Naffouri:
Image denoising via collaborative support-agnostic recovery.
IEEE International Conference on Acoustics, Speech and Signal Processing (ICASSP). Pages 1343-1347. 2017.
[bibtex] [web] [doi] [abstract]
Image denoising via collaborative support-agnostic recovery.
IEEE International Conference on Acoustics, Speech and Signal Processing (ICASSP). Pages 1343-1347. 2017.
[bibtex] [web] [doi] [abstract]
In this paper, we propose a novel patch-based image denoising algorithm using collaborative support-agnostic sparse reconstruction. In the proposed collaborative scheme, similar patches are assumed to share the same support taps. For sparse reconstruction, the likelihood of a tap being active in a patch is computed and refined through a collaboration process with other similar patches in the similarity group. This provides a very good patch support estimation, hence enhancing the quality of image restoration. Performance comparisons with state-of-the-art algorithms, in terms of PSNR and SSIM, demonstrate the superiority of the proposed algorithm.
2015
Csaba Benedek, Maha Shadaydeh, Zoltan Kato, Tamas Sziranyi, Josiane Zerubia:
Multilayer Markov Random Field models for change detection in optical remote sensing images.
ISPRS Journal of Photogrammetry and Remote Sensing. 107 : pp. 22-37. 2015.
[bibtex] [pdf] [web] [doi] [abstract]
Multilayer Markov Random Field models for change detection in optical remote sensing images.
ISPRS Journal of Photogrammetry and Remote Sensing. 107 : pp. 22-37. 2015.
[bibtex] [pdf] [web] [doi] [abstract]
In this paper, we give a comparative study on three Multilayer Markov Random Field (MRF) based sol utions proposed for change detection in optical remote sensing images, called Multicue MRF, Conditional Mixed Markov model, and Fusion MRF. Our purposes are twofold. On one hand, we highlight the significance of the focused model family and we set them against various state-of-the-art approaches through a thematic analysis and quantitative tests. We discuss the advantages and drawbacks of class comparison vs. direct approaches, usage of training data, various targeted application fields and different ways of Ground Truth generation, meantime informing the Reader in which roles the Multilayer MRFs can be efficiently applied. On the other hand we also emphasize the differences between the three focused models at various levels, considering the model structures, feature extraction, layer interpretation, change concept definition, parameter tuning and performance. We provide qualitative and quantitative comparison results using principally a publicly available change detection database which contains aerial image pairs and Ground Truth change masks. We conclude that the discussed models are competitive against alternative state-of-the-art solutions, if one uses them as pre-processing filters in multitemporal optical image analysis. In addition, they cover together a large range of applications, considering the different usage options of the three approaches.
Maha Shadaydeh, Andrea Manno-Kovacs, Tamas Sziranyi:
Change Detection in Wetlands using Multi-layer Fusion Markov Random Field.
What can Remote Sensing do for the Conservation of Wetlands? - 1st International Symposium. 2015.
[bibtex]
Change Detection in Wetlands using Multi-layer Fusion Markov Random Field.
What can Remote Sensing do for the Conservation of Wetlands? - 1st International Symposium. 2015.
[bibtex]
Maha Shadaydeh, Tamas Sziranyi:
An improved mutual information similarity measure for registration of multi-modal remote sensing images.
Proc. SPIE Remote Sensing. 9643 : pp. 9643 - 9643 - 7. 2015.
[bibtex] [web] [doi] [abstract]
An improved mutual information similarity measure for registration of multi-modal remote sensing images.
Proc. SPIE Remote Sensing. 9643 : pp. 9643 - 9643 - 7. 2015.
[bibtex] [web] [doi] [abstract]
Registration of multi-modal remote sensing images is an essential and challenging task in different remote sensing applications such as image fusion and multi-temporal change detection. Mutual Information (MI) has shown to be successful similarity measure for multi-modal image registration applications, however it has some drawbacks. 1. MI surface is highly non-convex with many local maxima. 2. Spatial information is completely lost in the calculation of the joint intensity probability distribution. In this paper, we present an improved MI similarity measure based on a new concept in integrating other image features as well as spatial information in the estimation of the joint intensity histogram which is used as an estimate of the joint probability distribution. The proposed method is based on the idea that each pixel in the reference image is assigned a weight, then each bin in the joint histogram is calculated as the summations of the weights of the pixels corresponding to that bin. The weight given to each pixel in the reference image is an exponential function of the corresponding pixel values in a distance image and a normalized gradient image such that higher weights are given to points close to one or more selected key points as well as points with high normalized gradient values. The proposed method is in essence a kind of calculating similarity measure using irregular sampling where sampling frequency is higher in areas close to key-points or areas with higher gradients. We have compared the proposed method with the conventional MI and Normalized MI methods for registration of pairs of multi-temporal multi-modal remote sensing images. We observed that the proposed method produces considerably better registration function containing fewer erroneous maxima and leading to higher success rate.
2014
Maha Shadaydeh, Tamas Sziranyi:
An improved local similarity measure estimation for change detection in remote sensing images.
International Conference on Aerospace Electronics and Remote Sensing Technology. Pages 234-238. 2014.
[bibtex] [web] [abstract]
An improved local similarity measure estimation for change detection in remote sensing images.
International Conference on Aerospace Electronics and Remote Sensing Technology. Pages 234-238. 2014.
[bibtex] [web] [abstract]
Detecting changes in remote sensing images taken at different times is challenging when images' data come from different sensors. The performance of change detection algorithms based on radiometric values alone is not satisfactory and need the fusion of other features. Local similarity measures such as Mutual Information, Kullback-Leibler Divergence, and Cluster Reward Algorithm can be used for enhancing change detection. In the paper, we propose an improved local similarity measure using weighted local histogram. Each pixel contributes to the calculation of the histogram according to its weight only. The weight assigned to each pixel in the histogram estimation window follows an exponential function of its distance from the center of the window and the corresponding pixel value in an initial change map image which is derived from other micro-structure or radiometric information. The proposed improved similarity measure benefits from the good detection ability of small estimation window and the good estimation accuracy of large estimation window; hence it can replace the time-consuming multi-scale selection approaches for statistics based similarity measures in remote sensing. The efficiency of this useful improvement has been validated on change detection in remote sensing image series.
Tamas Sziranyi, Maha Shadaydeh:
Segmentation of Remote Sensing Images Using Similarity-Measure-Based Fusion-MRF Model.
IEEE Geoscience and Remote Sensing Letters. 11 (9) : pp. 1544-1548. 2014.
[bibtex] [pdf] [web] [doi] [abstract]
Segmentation of Remote Sensing Images Using Similarity-Measure-Based Fusion-MRF Model.
IEEE Geoscience and Remote Sensing Letters. 11 (9) : pp. 1544-1548. 2014.
[bibtex] [pdf] [web] [doi] [abstract]
Classifying segments and detecting changes in terrestrial areas are important and time-consuming efforts for remote sensing image analysis tasks, including comparison and retrieval in repositories containing multitemporal remote image samples for the same area in very different quality and details. We propose a multilayer fusion model for adaptive segmentation and change detection of optical remote sensing image series, where trajectory analysis or direct comparison is not applicable. Our method applies unsupervised or partly supervised clustering on a fused-image series by using cross-layer similarity measure, followed by multilayer Markov random field segmentation. The resulted label map is applied for the automatic training of single layers. After the segmentation of each single layer separately, changes are detected between single label maps. The significant benefit of the proposed method has been numerically validated on remotely sensed image series with ground-truth data.
2013
Tamas Sziranyi, Maha Shadaydeh:
Improved segmentation of a series of remote sensing images by using a fusion MRF model.
International Workshop on Content-Based Multimedia Indexing (CBMI). Pages 137-142. 2013.
[bibtex] [web] [doi] [abstract]
Improved segmentation of a series of remote sensing images by using a fusion MRF model.
International Workshop on Content-Based Multimedia Indexing (CBMI). Pages 137-142. 2013.
[bibtex] [web] [doi] [abstract]
Classifying segments and detection of changes in terrestrial areas are important and time-consuming efforts for remote-sensing image repositories. Some country areas are scanned frequently (e.g. year-by-year) to spot relevant changes, and several repositories contain multi-temporal image samples for the same area in very different quality and details. We propose a Multi-Layer Markovian adaptive fusion on Luv color images and similarity measure for the segmentation and detection of changes in a series of remote sensing images. We aim the problem of detecting details in rarely scanned remote sensing areas, where trajectory analysis or direct comparison is not applicable. Our method applies unsupervised or partly supervised clustering based on a cross-image featuring, followed by multilayer MRF segmentation in the mixed dimensionality. On the base of the fused segmentation, the clusters of the single layers are trained by clusters of the mixed results. The improvement of this (partly) unsupervised method has been validated on remotely sensed image series.
2008
Maha Shadaydeh, Yegui Xiao, Rabab K. Ward:
Extraction of fetal ECG using adaptive Volterra filters.
European Signal Processing Conference. Pages 1-5. 2008.
[bibtex] [pdf] [web] [abstract]
Extraction of fetal ECG using adaptive Volterra filters.
European Signal Processing Conference. Pages 1-5. 2008.
[bibtex] [pdf] [web] [abstract]
In this paper we present a new method for extracting the fetal electrocardiogram (FECG) signal from one thoracic ECG signal and one or more abdominal signals. Our method is based on the use of an adaptive Volterra filter (AVF) that is capable of synthesizing the nonlinear relation between the mother thoracic ECG signal and the abdominal signals which contains a transformed mother ECG, the fetal ECG and other noise elements. An adaptive multi-sensory noise canceler structure is adopted for the extraction purpose. In the case where more than one abdominal signals are used, the proposed algorithm uses a linear combiner (LC) to form a primary signal from those abdominal signals. The LC and the AVF are updated by the RLS algorithm. The proposed method is applied to real ECG measurements to demonstrate its superior effectiveness.
Yegui Xiao, Maha Shadaydeh, Rabab K. Ward:
New narrowband active noise control systems with significantly less computational requirements.
IEEE International Symposium on Circuits and Systems. Pages 41-44. 2008.
[bibtex] [web] [doi] [abstract]
New narrowband active noise control systems with significantly less computational requirements.
IEEE International Symposium on Circuits and Systems. Pages 41-44. 2008.
[bibtex] [web] [doi] [abstract]
In a typical conventional narrowband ANC system, the discrete Fourier coefficients (DFC) for each frequency are estimated by a linear combiner. Each reference (cosine or sine) wave has to be filtered by an estimate of the secondary-path before it is fed to the LMS algorithm. We call this part x-filtering block. The number of x-filtering blocks is 2q where q is the number of targeted frequencies. For larger q and/or higher order (M) of estimated FIR-type secondary-path, the computational cost due to x-filtering may become a bottleneck in real system implementation. Here, we propose a new narrowband ANC system structure which requires only two (2) x-filtering blocks regardless of q. All the cosine waves (or sine waves) are combined as an input to a x-filtering block. The output of this block is decomposed by an efficient bandpass filter bank into filtered-x cosine or sine waves for the FXLMS that follows. The computational cost of the new system is significantly reduced especially for large q and/or M. The new structure is also modified to cope with the frequency mismatch (FM). Simulations demonstrate that the new systems present performance which is very similar to that of the conventional system, but enjoy great advantages in system implementation.
2003
2002
Maha Shadaydeh, Yegui Xiao, Masayuki Kawawata:
Steady StateAnalysis of the P-Power Algorithm for Constrained Adaptive IIR Notch Filter.
IEEE Midwest Symposium on Circuits and Systems (MWSCAS). Pages 664-667. 2002.
[bibtex] [abstract]
Steady StateAnalysis of the P-Power Algorithm for Constrained Adaptive IIR Notch Filter.
IEEE Midwest Symposium on Circuits and Systems (MWSCAS). Pages 664-667. 2002.
[bibtex] [abstract]
In this paper, we present the steady state analysis of the p-power algorithm for an adaptive IIR notch filter with constrained poles and zeros for the case when p=3 and the sinusoidal signal is contaminated with additive Gaussian noise. We first derive two difference equations for the convergence of the mean and the Mean Square Error (MSE), and then give the steady state estimation bias and MSE. Stability conditions based on the step size value are also discussed.
1999
Maha Shadaydeh, Masayuki Kawamata:
Bias Removal Algorithm for 2-D Equation Error Adaptive IIR Filters.
Multidimensional Systems and Signal Processing. 10 (4) : pp. 429-441. 1999.
[bibtex] [pdf] [web] [doi] [abstract]
Bias Removal Algorithm for 2-D Equation Error Adaptive IIR Filters.
Multidimensional Systems and Signal Processing. 10 (4) : pp. 429-441. 1999.
[bibtex] [pdf] [web] [doi] [abstract]
This paper proposes a bias removal algorithm for equation error-based 2-D adaptive cascade IIR filters with separable denominator function. As well known, equation error-based adaptive IIR filtering algorithms have the advantages of fast convergence and unimodal mean-square-error surface. These advantages, however, come along with the drawback of biased parameter estimates in the presence of measurement noise. The adaptive filter structure in the proposed algorithm is based on the concept of backpropagating the desired signal through a cascade of the denominator vertical and horizontal sections. To handle the bias problem, the proposed algorithm uses a scaled value of the output error of each of the cascaded sections as an estimate for the measurement noise embedded in the signal part of the coefficient-update procedure of that section. Thus, while maintaining the advantages of easy stability monitoring, fast convergence, and low computational load, the effect of the measurement noise is suppressed. Input-Output stability analysis is carried out, and the constraints required to maintain stability are derived. Simulation examples are presented to support the effectiveness and the usability of the proposed bias removal algorithm in 2-D system identification and image enhancement applications.
1998
Maha Shadaydeh, Masayuki Kawawata:
Convergence Analysis of 2-D Doubly-Indexed Block LMS Adaptive Filters.
IEEE International Workshop on Intelligent Signal Processing and Communication Systems. 1998.
[bibtex]
Convergence Analysis of 2-D Doubly-Indexed Block LMS Adaptive Filters.
IEEE International Workshop on Intelligent Signal Processing and Communication Systems. 1998.
[bibtex]
1997
Maha Shadaydeh, Yegui Xiao, Yoshiaki Tadokoro:
Identification of nonminimum phase FIR systems via fourth-order cumulants and genetic algorithm.
Signal Processing. 60 (3) : pp. 339-347. 1997.
[bibtex] [pdf] [web] [doi] [abstract]
Identification of nonminimum phase FIR systems via fourth-order cumulants and genetic algorithm.
Signal Processing. 60 (3) : pp. 339-347. 1997.
[bibtex] [pdf] [web] [doi] [abstract]
Abstract In this paper, the problem of estimating the parameters of an FIR system from only the fourth-order cumulants of the noisy system output is considered. The FIR system is driven by a symmetric, independent, and identically distributed (i.i.d) non-Gaussian sequence. We propose a new formula called Weighted Overdetermined C(q, k) (WOC(q, k)) by extending the conventional C(q, k) formula. The optimal selection of the weights in WOC(q, k) is performed by using the Genetic Algorithm (GA) optimization method which minimizes a nonlinear error function based on the fourth-order cumulants alone. Simulations are provided to reveal the effectiveness and the superiority of this novel technique over the C(q, k) and other existing techniques.
1996
Yegui Xiao, Maha Shadaydeh, Yoshiaki Tadokoro:
Overdetermined C(q,k) formula using third and fourth order cumulants.
Electronics Letters. 32 (6) : pp. 601-603. 1996.
[bibtex] [web] [doi] [abstract]
Overdetermined C(q,k) formula using third and fourth order cumulants.
Electronics Letters. 32 (6) : pp. 601-603. 1996.
[bibtex] [web] [doi] [abstract]
A new overdetermined C(q, k) formula using both third and fourth order cumulants is proposed for FIR system identification. This closed form formula is based on a scaled combination of two C(q, k) formulas using third and fourth order cumulants, respectively. It has a very simple form, and can offer good estimation performance that is comparable to those of other cumulant-based system identification techniques developed in the literature. Extensive simulations reveal its marked performance in coloured additive noise environments