Gideon Stein, M.Sc.
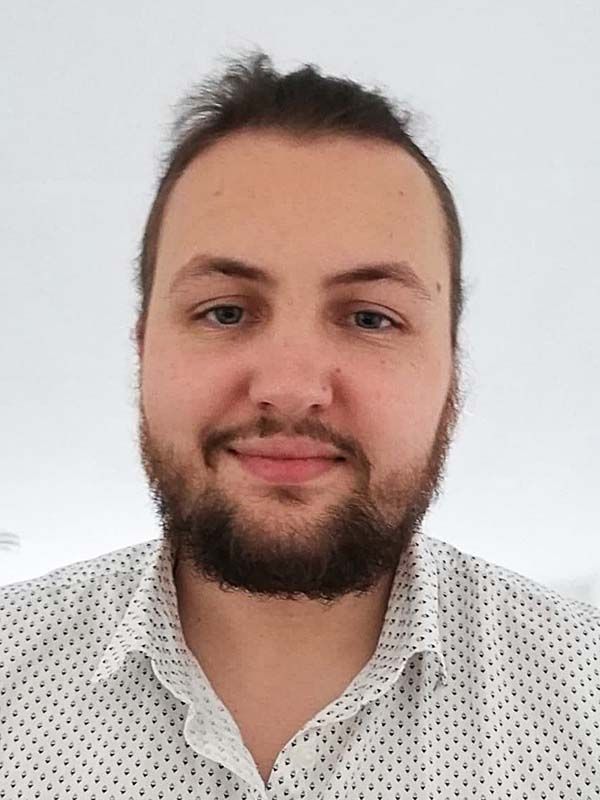
Address: | Computer Vision Group |
Department of Mathematics and Computer Science | |
Friedrich Schiller University of Jena | |
Ernst-Abbe-Platz 2 | |
07743 Jena | |
Germany | |
Phone: | +49 (0) 3641 9 46425 |
E-mail: | gideon (dot) stein (at) uni-jena (dot) de |
Room: | 1221 |
Links: |
Curriculum Vitae
since 2021 | PhD Student |
Friedrich Schiller University of Jena and iDiv | |
Research topic: “Causal Reasoning and Deep Learning for Understanding Changes int the | |
Soil-Plant-Climate Interactions” | |
2018 – 2020 | M.Sc. in Machine Learning & Data Analysis |
ITMO University, St. Petersburg, Russia | |
Focus: Machine Learning | |
Master Thesis: “Transformer based action sequence generation in reinforcement learning settings” | |
2014 – 2018 | B.A. in Philosophy and Economics |
University Bayreuth | |
Bachelor Thesis: “Reinforcement learning and the provision of public goods” |
Research Interests
- Deep Learning
- Causality
- Interdisciplinarity
- Language Generation
- Reinforcement Learning
Publications
2024
Gideon Stein, Jonas Ziemer, Carolin Wicker, Jannik Jaenichen, Gabriele Demisch, Daniel Kloepper, Katja Last, Joachim Denzler, Christiane Schmullius, Maha Shadaydeh, Clémence Dubois:
Data-driven Prediction of Large Infrastructure Movements Through Persistent Scatterer Time Series Modeling.
IEEE International Geoscience and Remote Sensing Symposium (IGARSS). 2024. (accepted)
[bibtex] [pdf]
Data-driven Prediction of Large Infrastructure Movements Through Persistent Scatterer Time Series Modeling.
IEEE International Geoscience and Remote Sensing Symposium (IGARSS). 2024. (accepted)
[bibtex] [pdf]
Gideon Stein, Maha Shadaydeh, Joachim Denzler:
Embracing the Black Box: Heading Towards Foundation Models for Causal Discovery from Time Series Data.
AAAI Workshop on AI for Time-series (AAAI-WS). 2024.
[bibtex] [pdf] [web] [abstract]
Embracing the Black Box: Heading Towards Foundation Models for Causal Discovery from Time Series Data.
AAAI Workshop on AI for Time-series (AAAI-WS). 2024.
[bibtex] [pdf] [web] [abstract]
Causal discovery from time series data encompasses many existing solutions, including those based on deep learning techniques. However, these methods typically do not endorse one of the most prevalent paradigms in deep learning: End-to-end learning. To address this gap, we explore what we call Causal Pretraining. A methodology that aims to learn a direct mapping from multivariate time series to the underlying causal graphs in a supervised manner. Our empirical findings suggest that causal discovery in a supervised manner is possible, assuming that the training and test time series samples share most of their dynamics. More importantly, we found evidence that the performance of Causal Pretraining can increase with data and model size, even if the additional data do not share the same dynamics. Further, we provide examples where causal discovery for real-world data with causally pretrained neural networks is possible within limits. We argue that this hints at the possibility of a foundation model for causal discovery.
Gideon Stein, Sai Karthikeya Vemuri, Yuanyuan Huang, Anne Ebeling, Nico Eisenhauer, Maha Shadaydeh, Joachim Denzler:
Investigating the Effects of Plant Diversity on Soil Thermal Diffusivity Using Physics- Informed Neural Networks.
ICLR Workshop on AI4DifferentialEquations In Science (ICLR-WS). 2024.
[bibtex] [pdf] [web]
Investigating the Effects of Plant Diversity on Soil Thermal Diffusivity Using Physics- Informed Neural Networks.
ICLR Workshop on AI4DifferentialEquations In Science (ICLR-WS). 2024.
[bibtex] [pdf] [web]
Niklas Penzel, Gideon Stein, Joachim Denzler:
Reducing Bias in Pre-trained Models by Tuning while Penalizing Change.
International Conference on Computer Vision Theory and Applications (VISAPP). Pages 90-101. 2024.
[bibtex] [web] [doi] [abstract]
Reducing Bias in Pre-trained Models by Tuning while Penalizing Change.
International Conference on Computer Vision Theory and Applications (VISAPP). Pages 90-101. 2024.
[bibtex] [web] [doi] [abstract]
Deep models trained on large amounts of data often incorporate implicit biases present during training time. If later such a bias is discovered during inference or deployment, it is often necessary to acquire new data and retrain the model. This behavior is especially problematic in critical areas such as autonomous driving or medical decision-making. In these scenarios, new data is often expensive and hard to come by. In this work, we present a method based on change penalization that takes a pre-trained model and adapts the weights to mitigate a previously detected bias. We achieve this by tuning a zero-initialized copy of a frozen pre-trained network. Our method needs very few, in extreme cases only a single, examples that contradict the bias to increase performance. Additionally, we propose an early stopping criterion to modify baselines and reduce overfitting. We evaluate our approach on a well-known bias in skin lesion classification and three other datasets from the domain shift literature. We find that our approach works especially well with very few images. Simple fine-tuning combined with our early stopping also leads to performance benefits for a larger number of tuning samples.
Tristan Piater, Niklas Penzel, Gideon Stein, Joachim Denzler:
When Medical Imaging Met Self-Attention: A Love Story That Didn’t Quite Work Out.
International Conference on Computer Vision Theory and Applications (VISAPP). Pages 149-158. 2024.
[bibtex] [web] [doi] [abstract]
When Medical Imaging Met Self-Attention: A Love Story That Didn’t Quite Work Out.
International Conference on Computer Vision Theory and Applications (VISAPP). Pages 149-158. 2024.
[bibtex] [web] [doi] [abstract]
A substantial body of research has focused on developing systems that assist medical professionals during labor-intensive early screening processes, many based on convolutional deep-learning architectures. Recently, multiple studies explored the application of so-called self-attention mechanisms in the vision domain. These studies often report empirical improvements over fully convolutional approaches on various datasets and tasks. To evaluate this trend for medical imaging, we extend two widely adopted convolutional architectures with different self-attention variants on two different medical datasets. With this, we aim to specifically evaluate the possible advantages of additional self-attention. We compare our models with similarly sized convolutional and attention-based baselines and evaluate performance gains statistically. Additionally, we investigate how including such layers changes the features learned by these models during the training. Following a hyperparameter search, and contrary to our expectations, we observe no significant improvement in balanced accuracy over fully convolutional models. We also find that important features, such as dermoscopic structures in skin lesion images, are still not learned by employing self-attention. Finally, analyzing local explanations, we confirm biased feature usage. We conclude that merely incorporating attention is insufficient to surpass the performance of existing fully convolutional methods.
2023
Yuanyuan Huang, Gideon Stein, Olaf Kolle, Karl Kuebler, Ernst-Detlef Schulze, Hui Dong, David Eichenberg, Gerd Gleixner, Anke Hildebrandt, Markus Lange, Christiane Roscher, Holger Schielzeth, Bernhard Schmid, Alexandra Weigelt, Wolfgang W. Weisser, Maha Shadaydeh, Joachim Denzler, Anne Ebeling, Nico Eisenhauer:
Enhanced Stability of Grassland Soil Temperature by Plant Diversity.
Nature Geoscience. pp. 1-7. 2023.
[bibtex] [doi] [abstract]
Enhanced Stability of Grassland Soil Temperature by Plant Diversity.
Nature Geoscience. pp. 1-7. 2023.
[bibtex] [doi] [abstract]
Extreme weather events are occurring more frequently, and research has shown that plant diversity can help mitigate the impacts of climate change by increasing plant productivity and ecosystem stability. Although soil temperature and its stability are key determinants of essential ecosystem processes, no study has yet investigated whether plant diversity buffers soil temperature fluctuations over long-term community development. Here we have conducted a comprehensive analysis of a continuous 18-year dataset from a grassland biodiversity experiment with high spatial and temporal resolutions. Our findings reveal that plant diversity acts as a natural buffer, preventing soil heating in hot weather and cooling in cold weather. This diversity effect persists year-round, intensifying with the aging of experimental communities and being even stronger under extreme climate conditions, such as hot days or dry years. Using structural equation modelling, we found that plant diversity stabilizes soil temperature by increasing soil organic carbon concentrations and, to a lesser extent, plant leaf area index. Our results suggest that, in lowland grasslands, the diversity-induced stabilization of soil temperature may help to mitigate the negative effects of extreme climatic events such as soil carbon decomposition, thus slowing global warming.
Yuanyuan Huang, Gideon Stein, Olaf Kolle, Karl Kuebler, Ernst-Detlef Schulze, Hui Dong, David Eichenberg, Gerd Gleixner, Anke Hildebrandt, Markus Lange, Christiane Roscher, Holger Schielzeth, Bernhard Schmid, Alexandra Weigelt, Wolfgang W. Weisser, Maha Shadaydeh, Joachim Denzler, Anne Ebeling, Nico Eisenhauer:
Plant Diversity Stabilizes Soil Temperature.
bioRxiv. pp. 2023-03. 2023.
[bibtex] [pdf]
Plant Diversity Stabilizes Soil Temperature.
bioRxiv. pp. 2023-03. 2023.
[bibtex] [pdf]
2022
Clemence Dubois, Jannik Jänichen, Maha Shadaydeh, Gideon Stein, Alexandra Katz, Daniel Klöpper, Joachim Denzler, Christiane Schmullius, Katja Last:
KI4KI: Neues Projekt zur regelmässigen Überwachung von Stauanlagen aus dem All.
Messtechnische Überwachung von Stauanlagen ; XII.Mittweidaer Talsperrentag. Pages 15-19. 2022.
[bibtex] [web] [doi] [abstract]
KI4KI: Neues Projekt zur regelmässigen Überwachung von Stauanlagen aus dem All.
Messtechnische Überwachung von Stauanlagen ; XII.Mittweidaer Talsperrentag. Pages 15-19. 2022.
[bibtex] [web] [doi] [abstract]
Die Überwachung von Staubauwerken stellt Stauanlagenbetreiber vor viele Herausforderungen. Insbesondere aufgrund der Kosten und des Zeitaufwandes werden Staubauwerke oft nur ein- bis zweimal im Jahr durch trigonometrische Messungen überwacht. Seit einigen Jahrzehnten liefern jedoch Radarsatellitendaten nützliche Informationen zum Infrastrukturmonitoring. Satellitendaten der Copernicus Sentinel-1 Mission erlauben es, mittels der Technik der Persistent Scatterer Interferometrie (PSI), Deformationsmessungen von Staubauwerken im Millimeterbereich mit einem zeitlichen Abstand von 6 bis 12 Tagen durchzuführen. In einem Verbundprojekt zwischen der Friedrich-Schiller-Universität Jena und dem Ruhrverband soll ein Dienst entwickelt werden, der bisherige Überwachungsstrategien der Anlagen durch Nutzung der PSI Technik verbessert. Zudem sollen neuartige Geräte genutzt werden, die die Sichtbarkeit der Stauanlagen im Satellitenbild erhöhen sowie Methoden der künstlichen Intelligenz genutzt werden, um Deformationen im Falle von Extremwetterereignissen besser vorhersagen zu können.
Sven Festag, Gideon Stein, Tim Büchner, Maha Shadaydeh, Joachim Denzler, Cord Spreckelsen:
Outcome Prediction and Murmur Detection in Sets of Phonocardiograms by a Deep Learning-Based Ensemble Approach.
Computing in Cardiology (CinC). Pages 1-4. 2022.
[bibtex] [pdf] [doi] [abstract]
Outcome Prediction and Murmur Detection in Sets of Phonocardiograms by a Deep Learning-Based Ensemble Approach.
Computing in Cardiology (CinC). Pages 1-4. 2022.
[bibtex] [pdf] [doi] [abstract]
We, the team UKJ_FSU, propose a deep learning system for the prediction of congenital heart diseases. Our method is able to predict the clinical outcomes (normal, abnormal) of patients as well as to identify heart murmur (present, absent, unclear) based on phonocardiograms recorded at different auscultation locations. The system we propose is an ensemble of four temporal convolutional networks with identical topologies, each specialized in identifying murmurs and predicting patient outcome from a phonocardiogram taken at one specific auscultation location. Their intermediate outputs are augmented by the manually ascertained patient features such as age group, sex, height, and weight. The outputs of the four networks are combined to form a single final decision as demanded by the rules of the George B. Moody PhysioNet Challenge 2022. On the first task of this challenge, the murmur detection, our model reached a weighted accuracy of 0.567 with respect to the validation set. On the outcome prediction task (second task) the ensemble led to a mean outcome cost of 10679 on the same set. By focusing on the clinical outcome prediction and tuning some of the hyper-parameters only for this task, our model reached a cost score of 12373 on the official test set (rank 13 of 39). The same model scored a weighted accuracy of 0.458 regarding the murmur detection on the test set (rank 37 of 40).
2020
Arip Asadulaev, Igor Kuznetsov, Gideon Stein, Andrey Filchenkov:
Exploring and Exploiting Conditioning of Reinforcement Learning Agents.
IEEE Access. 8 : pp. 211951-211960. 2020.
[bibtex] [abstract]
Exploring and Exploiting Conditioning of Reinforcement Learning Agents.
IEEE Access. 8 : pp. 211951-211960. 2020.
[bibtex] [abstract]
The outcome of Jacobian singular values regularization was studied for supervised learning problems. In supervised learning settings for linear and nonlinear networks, Jacobian regularization allows for faster learning. It also was shown that Jacobian conditioning regularization can help to avoid the “mode-collapse” problem in Generative Adversarial Networks. In this paper, we try to answer the following question: Can information about policy network Jacobian conditioning help to shape a more stable and general policy of reinforcement learning agents? To answer this question, we conduct a study of Jacobian conditioning behavior during policy optimization. We analyze the behavior of the agent conditioning on different policies under the different sets of hyperparameters and study a correspondence between the conditioning and the ratio of achieved rewards. Based on these observations, we propose a conditioning regularization technique. We apply it to Trust Region Policy Optimization and Proximal Policy Optimization (PPO) algorithms and compare their performance on 8 continuous control tasks. Models with the proposed regularization outperformed other models on most of the tasks. Also, we showed that the regularization improves the agent's generalization by comparing the PPO performance on CoinRun environments. Also, we propose an algorithm that uses the condition number of the agent to form a robust policy, which we call Jacobian Policy Optimization (JPO). It directly estimates the condition number of an agent's Jacobian and changes the policy trend. We compare it with PPO on several continuous control tasks in PyBullet environments and the proposed algorithm provides a more stable and efficient reward growth on a range of agents.
Gideon Stein, Andrey Filchenkov, Arip Asadulaev:
Stabilizing Transformer-Based Action Sequence Generation For Q-Learning.
arXiv preprint 2010.12698. 2020.
[bibtex] [pdf] [abstract]
Stabilizing Transformer-Based Action Sequence Generation For Q-Learning.
arXiv preprint 2010.12698. 2020.
[bibtex] [pdf] [abstract]
Since the publication of the original Transformer architecture (Vaswani et al. 2017), Transformers revolutionized the field of Natural Language Processing. This, mainly due to their ability to understand timely dependencies better than competing RNN-based architectures. Surprisingly, this architecture change does not affect the field of Reinforcement Learning (RL), even though RNNs are quite popular in RL, and time dependencies are very common in RL. Recently, Parisotto et al. 2019) conducted the first promising research of Transformers in RL. To support the findings of this work, this paper seeks to provide an additional example of a Transformer-based RL method. Specifically, the goal is a simple Transformer-based Deep Q-Learning method that is stable over several environments. Due to the unstable nature of Transformers and RL, an extensive method search was conducted to arrive at a final method that leverages developments around Transformers as well as Q-learning. The proposed method can match the performance of classic Q-learning on control environments while showing potential on some selected Atari benchmarks. Furthermore, it was critically evaluated to give additional insights into the relation between Transformers and RL.