Wasim Ahmad, M.Sc.
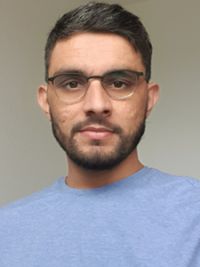
Address: | Computer Vision Group |
Department of Mathematics and Computer Science | |
Friedrich Schiller University of Jena Ernst-Abbe-Platz 2 | |
07743 Jena | |
Germany | |
Phone: | +49 (0) 3641 9 46427 |
E-mail: | wasim (dot) ahmad (at) uni-jena (dot) de |
Room: | 1221 |
Links: | GoogleScholar |
Curriculum Vitae
since 2020 | PhD Student |
Computer Vision Group Jena, Friedrich Schiller University Jena, Germany | |
Research Topic: “Causal Inference using Deep Learning” | |
2017 – 2019 | Professional Doctorate in Engineering (PDEng) |
Dynamics based Maintenance (DBM) Group, University of Twente, Enschede Netherlands | |
STRUKTON RAIL, Utrecht Netherlands | |
Project: “Artificial Intelligence based Condition Monitoring of Rail Infrastructure” | |
2015 – 2017 | M.Sc. Computer Engineering |
Ulsan Industrial Artificial Intelligence (UIAI) Laboratory, University of Ulsan, South Korea | |
Master Thesis: “Prognosis of Induction Motors using Machine Learning & Digital Signal Processing Techniques” | |
2008 – 2012 | B.Sc. Information and Communication Systems Engineering |
School of Electrical Engineering and Computer Science, National University of Sciences and Technology, Pakistan | |
Bachelor Thesis: “Next Generation Resource Tracking (XRT) Using RFID Technology” |
Research Interests
- Applied Deep Learning / Machine Learning
- Causal Reasoning
- Data-driven Predictive Maintenance
Publications
2024
Wasim Ahmad, Maha Shadaydeh, Joachim Denzler:
Deep Learning-based Group Causal Inference in Multivariate Time-series.
AAAI Workshop on AI for Time-series (AAAI-WS). 2024. (accepted)
[bibtex] [pdf] [web] [abstract]
Deep Learning-based Group Causal Inference in Multivariate Time-series.
AAAI Workshop on AI for Time-series (AAAI-WS). 2024. (accepted)
[bibtex] [pdf] [web] [abstract]
Causal inference in a nonlinear system of multivariate time series is instrumental in disentangling the intricate web of relationships among variables, enabling us to make more accurate predictions and gain deeper insights into real-world complex systems. Causality methods typically identify the causal structure of a multivariate system by considering the cause-effect relationship of each pair of variables while ignoring the collective effect of a group of variables or interactions involving more than two-time series variables. In this work, we test model invariance by group-level interventions on the trained deep networks to infer causal direction in groups of variables, such as climate and ecosystem, brain networks, etc. Extensive testing with synthetic and real-world time series data shows a significant improvement of our method over other applied group causality methods and provides us insights into real-world time series. The code for our method can be found at: https://github.com/wasimahmadpk/gCause.
2022
Wasim Ahmad, Maha Shadaydeh, Joachim Denzler:
Causal Discovery using Model Invariance through Knockoff Interventions.
ICML Workshop on Spurious Correlations, Invariance and Stability (ICML-WS). 2022.
[bibtex] [pdf] [web] [abstract]
Causal Discovery using Model Invariance through Knockoff Interventions.
ICML Workshop on Spurious Correlations, Invariance and Stability (ICML-WS). 2022.
[bibtex] [pdf] [web] [abstract]
Cause-effect analysis is crucial to understand the underlying mechanism of a system. We propose to exploit model invariance through interventions on the predictors to infer causality in nonlinear multivariate systems of time series. We model nonlinear interactions in time series using DeepAR and then expose the model to different environments using Knockoffs-based interventions to test model invariance. Knockoff samples are pairwise exchangeable, in-distribution and statistically null variables generated without knowing the response. We test model invariance where we show that the distribution of the response residual does not change significantly upon interventions on non-causal predictors. We evaluate our method on real and synthetically generated time series. Overall our method outperforms other widely used causality methods, i.e, VAR Granger causality, VARLiNGAM and PCMCI+.
2021
Wasim Ahmad, Maha Shadaydeh, Joachim Denzler:
Causal Inference in Non-linear Time-series using Deep Networks and Knockoff Counterfactuals.
IEEE International Conference on Machine Learning and Applications (ICMLA). Pages 449-454. 2021.
[bibtex] [pdf] [web] [doi] [abstract]
Causal Inference in Non-linear Time-series using Deep Networks and Knockoff Counterfactuals.
IEEE International Conference on Machine Learning and Applications (ICMLA). Pages 449-454. 2021.
[bibtex] [pdf] [web] [doi] [abstract]
Estimating causal relations is vital in understanding the complex interactions in multivariate time series. Non-linear coupling of variables is one of the major challenges in accurate estimation of cause-effect relations. In this paper, we propose to use deep autoregressive networks (DeepAR) in tandem with counterfactual analysis to infer nonlinear causal relations in multivariate time series. We extend the concept of Granger causality using probabilistic forecasting with DeepAR. Since deep networks can neither handle missing input nor out-of-distribution intervention, we propose to use the Knockoffs framework (Barber and Candes, 2015) for generating intervention variables and consequently counterfactual probabilistic forecasting. Knockoff samples are independent of their output given the observed variables and exchangeable with their counterpart variables without changing the underlying distribution of the data. We test our method on synthetic as well as real-world time series datasets. Overall our method outperforms the widely used vector autoregressive Granger causality and PCMCI in detecting nonlinear causal dependency in multivariate time series.