Moien Rangzan, M.Sc.
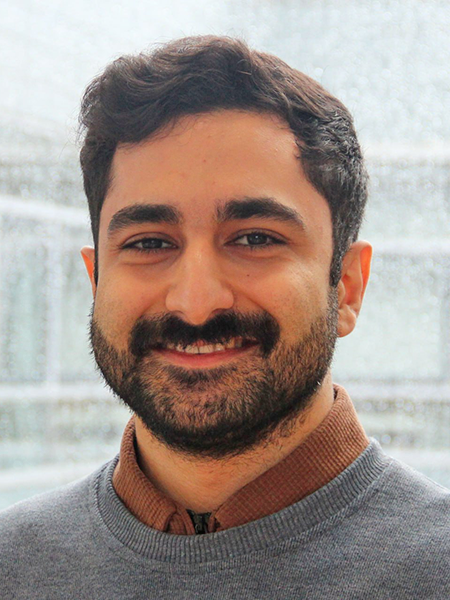
Address: | Computer Vision Group |
Department of Mathematics and Computer Science | |
Friedrich Schiller University of Jena | |
Ernst-Abbe-Platz 2 | |
07743 Jena | |
Germany | |
Phone: | +49 (0) 3641 9 46427 |
E-mail: | mrangzan (at) bgc-jena (dot) mpg (dot) de |
Room: | 1221 |
Links: |
Curriculum Vitae
since 2025 | PhD Student |
Friedrich Schiller University of Jena and | |
Max Planck Institute for Biogeochemistry | |
Research topic: “Stable Feature Learning for Naturalness Mapping” | |
2021 – 2024 | M.Sc. in Remote Sensing |
University of Tehran, Iran | |
Focus: Synthetic Aperture Radar (SAR) and Deep Learning | |
Master Thesis: “SAR Temporal Shifting: A New Approach for Optical-to-SAR Translation | |
with Consistent Viewing Geometry” (Best M.Sc. Thesis Award) | |
2015 – 2021 | B.Sc. In Electrical Engineering with a Major in Telecoms. |
K.N.Toosi University of Technology | |
Bachelor Thesis: “A Reinforcement Learning Approach for Stable Power Allocation | |
in NOMA(5G) Modulation” |
Research Interests
- Robust ML
- Radar Remote Sensing
- Computer Vision
Publications
2024
Moien Rangzan, Sara Attarchi, Richard Gloaguen, Seyed Kazem Alavipanah:
SAR Temporal Shifting: A New Approach for Optical-to-SAR Translation with Consistent Viewing Geometry.
Remote Sensing. 16 (16) : 2024.
[bibtex] [web] [doi] [abstract]
SAR Temporal Shifting: A New Approach for Optical-to-SAR Translation with Consistent Viewing Geometry.
Remote Sensing. 16 (16) : 2024.
[bibtex] [web] [doi] [abstract]
In contrast to the well-investigated field of Synthetic Aperture Radar (SAR)-to-Optical translation, this study explores the lesser-investigated domain of Optical-to-SAR translation, which is a challenging field due to the ill-posed nature of this translation. The complexity arises as single optical data can have multiple SAR representations based on the SAR viewing geometry. To generate an SAR image with a specific viewing geometry, we propose a novel approach, which is termed SAR Temporal Shifting. Our model takes an optical image from the target timestamp and an SAR image from a different temporal point but with a consistent viewing geometry as the expected SAR image. Both of these inputs are complemented with a change map derived from optical images during the intervening period. This model then modifies the SAR data based on the changes observed in the optical data to generate the SAR data for the desired timestamp. Although similar strategies have been explored in the opposite SAR-to-Optical translation, our approach innovates by introducing new spatial evaluation metrics and cost functions. These metrics reveal that simply adding same-domain data as model input, without accounting for the distribution changes in the dataset, can result in model overfitting—even if traditional metrics suggest positive outcomes. To address this issue, we have introduced a change-weighted loss function that discourages the model from merely replicating input data by assigning greater cost to changes in the areas of interest. Our approach surpasses traditional translation methods by eliminating the Generative Adversarial Network’s (GAN’s) fiction phenomenon by learning to change the SAR data based on the optical data instead of solely relying on translation. Furthering the field, we have introduced a novel automated framework to build a despeckled multitemporal SAR–Optical dataset with consistent viewing geometry. We provide the code and the dataset used in our study.
Nafiseh Kakhani, Moien Rangzan, Ali Jamali, Sara Attarchi, Seyed Kazem Alavipanah, Michael Mommert:
SSL-SoilNet: A Hybrid Transformer-Based Framework With Self-Supervised Learning for Large-Scale Soil Organic Carbon Prediction.
IEEE Transactions on Geoscience and Remote Sensing. 62 : pp. 1-15. 2024.
[bibtex] [web] [doi] [abstract]
SSL-SoilNet: A Hybrid Transformer-Based Framework With Self-Supervised Learning for Large-Scale Soil Organic Carbon Prediction.
IEEE Transactions on Geoscience and Remote Sensing. 62 : pp. 1-15. 2024.
[bibtex] [web] [doi] [abstract]
Soil organic carbon (SOC) constitutes a fundamental component of terrestrial ecosystem functionality, playing a pivotal role in nutrient cycling, hydrological balance, and erosion mitigation. Precise mapping of SOC distribution is imperative for the quantification of ecosystem services, notably carbon sequestration and soil fertility enhancement. Digital soil mapping (DSM) leverages statistical models and advanced technologies, including machine learning (ML), to accurately map soil properties, such as SOC, utilizing diverse data sources like satellite imagery, topography, remote sensing indices, and climate series. Within the domain of ML, self-supervised learning (SSL), which exploits unlabeled data, has gained prominence in recent years. This study introduces a novel approach that aims to learn the geographical link between multimodal features via self-supervised contrastive learning, employing pretrained Vision Transformers (ViT) for image inputs and Transformers for climate data, before fine-tuning the model with ground reference samples. The proposed approach has undergone rigorous testing on two distinct large-scale datasets, with results indicating its superiority over traditional supervised learning models, which depends solely on labeled data. Furthermore, through the utilization of various evaluation metrics (e.g., root-mean-square error (RMSE), mean absolute error (MAE), concordance correlation coefficient (CCC), etc.), the proposed model exhibits higher accuracy when compared to other conventional ML algorithms like random forest and gradient boosting. This model is a robust tool for predicting SOC and contributes to the advancement of DSM techniques, thereby facilitating land management and decision-making processes based on accurate information.
2022
Moien Rangzan, Sara Attarchi:
Removing Stripe Noise from Satellite Images using Convolutional Neural Networks in Frequency Domain.
European Geosciences Union General Assembly (EGU): Abstract + Oral Presentation. 2022.
[bibtex] [doi] [abstract]
Removing Stripe Noise from Satellite Images using Convolutional Neural Networks in Frequency Domain.
European Geosciences Union General Assembly (EGU): Abstract + Oral Presentation. 2022.
[bibtex] [doi] [abstract]
Many satellite images are corrupted by stripping; this noise degrades the visual quality of the images and inevitably introduces errors in processing. Thermal and hyperspectral images often suffer from stripping. The frequency distribution characteristic of stripe noise makes it difficult to remove such noise in the spatial domain; contrariwise, this noise can be efficiently detected in the frequency domain. Numerous solutions have been proposed to eliminate such noise using Fourier transform; however, most are subjective and time-consuming approaches.The lack of a fast and automated tool in this subject has motivated us to introduce a Convolutional Neural Network-based tool that uses the U-Net architecture in the frequency domain to suppress the anomalies caused by stripe noise. We added synthetic noise to satellite images to train the model. Then, we taught the network how to mask these anomalies in the frequency domain. The input image dataset was down-sampled to a size of 128 x128 pixels for a fast training time. However, our results suggest that the output mask can be up-scaled and applied on the original Fourier transform of the image and still achieve satisfying results; this means that the proposed algorithm is applicable on images regardless of their size. After the training step, the U-Net architecture can confidently find the anomalies and create an acceptable bounding mask; the results show that - with enough training data- the proposed procedure can efficiently remove stripe noise from all sorts of images. At this stage, we are trying to further develop the model to detect and suppress more complex synthetic noise. Next, we will focus on removing real stripe noise on satellite images to present a robust tool.