Laines Schmalwasser, M.Sc.
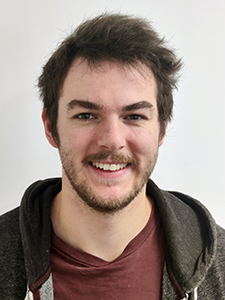
Address: | Computer Vision Group |
Department of Mathematics and Computer Science | |
Friedrich Schiller University of Jena | |
Ernst-Abbe-Platz 2 | |
07743 Jena | |
Germany | |
E-mail: | laines (dot) schmalwasser (at) dlr (dot) de |
Room: | 1212 |
Links: |
Curriculum Vitae
Research Interests
Projects
Publications
2024
Laines Schmalwasser, Jakob Gawlikowski, Joachim Denzler, Julia Niebling:
Exploiting Text-Image Latent Spaces for the Description of Visual Concepts.
International Conference on Pattern Recognition (ICPR). Pages 109-125. 2024.
[bibtex] [doi] [abstract]
Exploiting Text-Image Latent Spaces for the Description of Visual Concepts.
International Conference on Pattern Recognition (ICPR). Pages 109-125. 2024.
[bibtex] [doi] [abstract]
Concept Activation Vectors (CAVs) offer insights into neural network decision-making by linking human friendly concepts to the model's internal feature extraction process. However, when a new set of CAVs is discovered, they must still be translated into a human understandable description. For image-based neural networks, this is typically done by visualizing the most relevant images of a CAV, while the determination of the concept is left to humans. In this work, we introduce an approach to aid the interpretation of newly discovered concept sets by suggesting textual descriptions for each CAV. This is done by mapping the most relevant images representing a CAV into a text-image embedding where a joint description of these relevant images can be computed. We propose utilizing the most relevant receptive fields instead of full images encoded. We demonstrate the capabilities of this approach in multiple experiments with and without given CAV labels, showing that the proposed approach provides accurate descriptions for the CAVs and reduces the challenge of concept interpretation.